Automated anatomical labeling of a topologically variant abdominal arterial system via probabilistic hypergraph matching.
Medical image analysis(2021)
摘要
Automated anatomical vessel labeling of the abdominal arterial system is a crucial topic in medical image processing. One reason for this is the importance of the abdominal arterial system in the human body, and another is that such labeling is necessary for the related disease diagnoses, treatments and epidemiological population analyses. We define a hypergraph representation of the abdominal arterial system as a family tree model with a probabilistic hypergraph matching framework for automated vessel labeling. Then we treat the labelling problem as the convex optimization problem and solve it with the maximum a posteriori(MAP) combined the likelihood obtained by geometric labelling with the family tree topology-based knowledge. Geometrically, we utilize XGBoost ensemble learning with an intrinsic geometric feature importance analysis for branch-level labeling. In topology, the defined family tree model of the abdominal arterial system is transferred as a Markov chain model using a constrained traversal order method and further the Markov chain model is optimized by a hidden Markov model (HMM). The probability distribution of the target branches for each candidate anatomical name is predicted and effectively embedded in the HMM model. This approach is evaluated with the leave-one-out method on 37 clinical patients' abdominal arteries, and the average accuracy is 91.94%. The obtained results are better than those of the state-of-art method with an F1 score of 93.00% and a recall of 93.00%, as the proposed method simultaneously handles the anatomical structural variability and discriminates between the symmetric branches. It is demonstrated to be suitable for labelling branches of the abdominal arterial system and can also be extended to similar tubular organ networks, such as arterial or airway networks.
更多查看译文
AI 理解论文
溯源树
样例
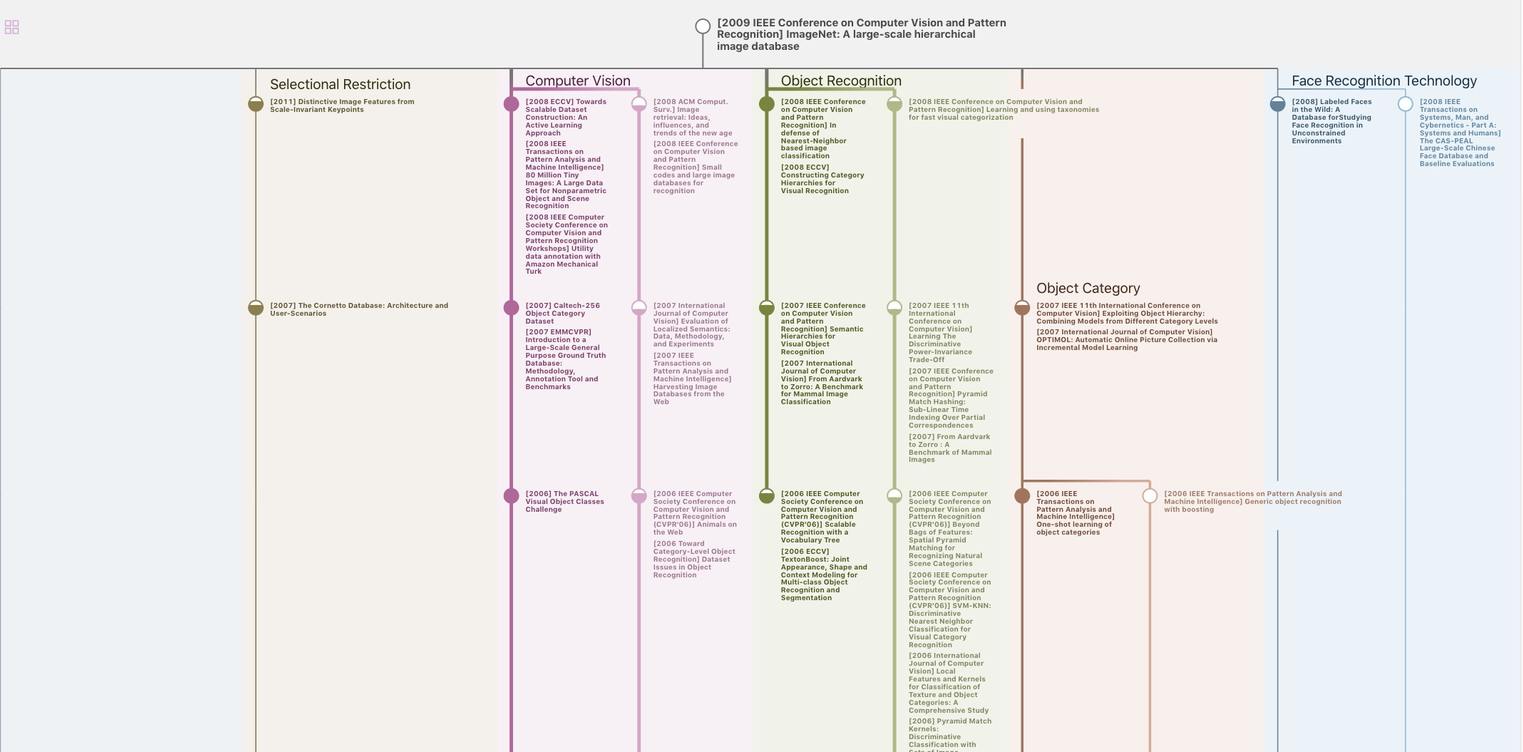
生成溯源树,研究论文发展脉络
Chat Paper
正在生成论文摘要