Residual-Guided Learning Representation for Self-Supervised Monocular Depth Estimation
CoRR(2021)
摘要
Photometric consistency loss is one of the representative objective functions commonly used for self-supervised monocular depth estimation. However, this loss often causes unstable depth predictions in textureless or occluded regions due to incorrect guidance. Recent self-supervised learning approaches tackle this issue by utilizing feature representations explicitly learned from auto-encoders, expecting better discriminability than the input image. Despite the use of auto-encoded features, we observe that the method does not embed features as discriminative as auto-encoded features. In this paper, we propose residual guidance loss that enables the depth estimation network to embed the discriminative feature by transferring the discriminability of auto-encoded features. We conducted experiments on the KITTI benchmark and verified our method's superiority and orthogonality on other state-of-the-art methods.
更多查看译文
关键词
depth estimation,learning,residual-guided,self-supervised
AI 理解论文
溯源树
样例
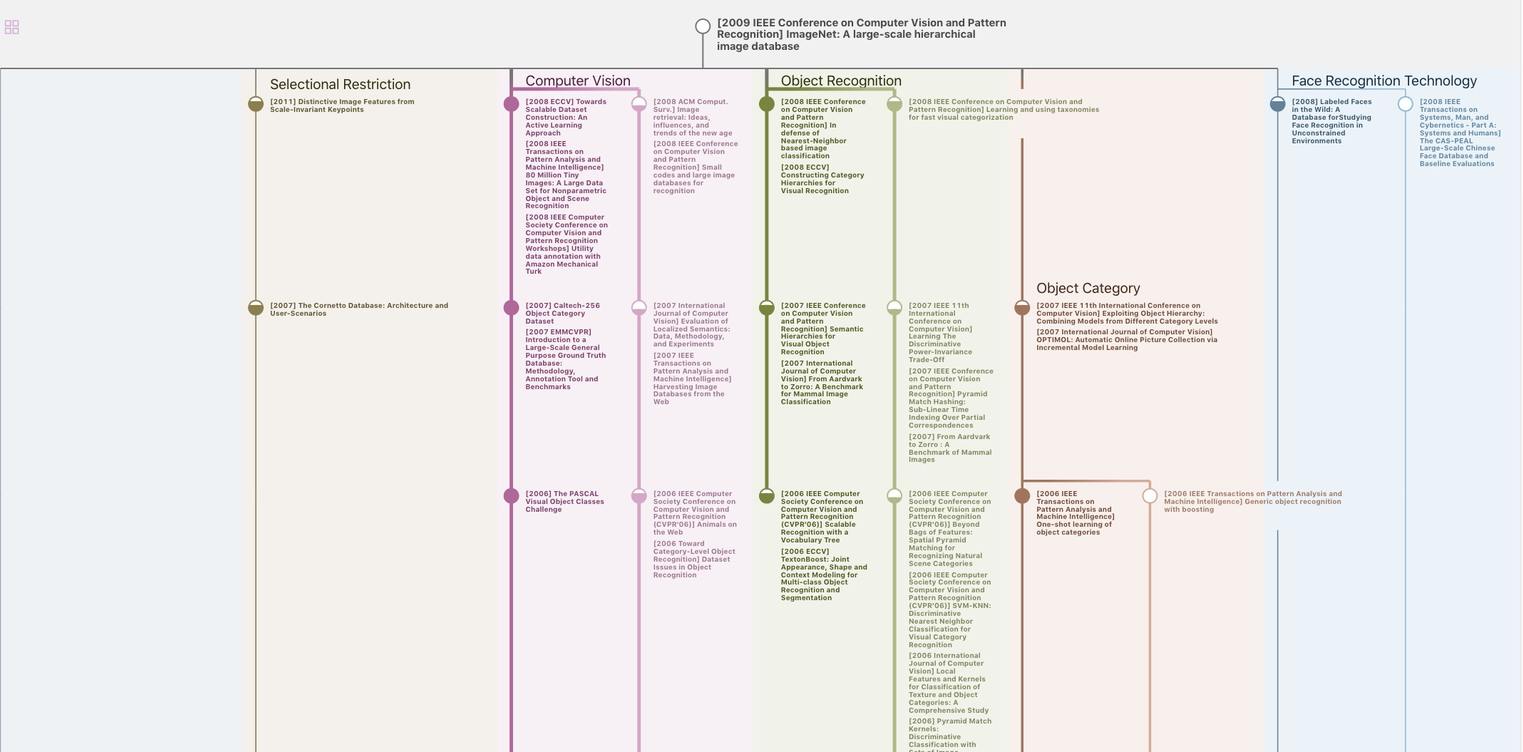
生成溯源树,研究论文发展脉络
Chat Paper
正在生成论文摘要