Sampling Equivariant Self-Attention Networks for Object Detection in Aerial Images
IEEE TRANSACTIONS ON IMAGE PROCESSING(2023)
摘要
Objects in aerial images show greater variations in scale and orientation than in other images, making them harder to detect using vanilla deep convolutional neural networks. Networks with sampling equivariance can adapt sampling from input feature maps to object transformation, allowing a convolutional kernel to extract effective object features under different transformations. However, methods such as deformable convolutional networks can only provide sampling equivariance under certain circumstances, as they sample by location. We propose sampling equivariant self-attention networks, which treat self-attention restricted to a local image patch as convolution sampling by masks instead of locations, and a transformation embedding module to improve the equivariant sampling further. We further propose a novel randomized normalization module to enhance network generalization and a quantitative evaluation metric to fairly evaluate the ability of sampling equivariance of different models. Experiments show that our model provides significantly better sampling equivariance than existing methods without additional supervision and can thus extract more effective image features. Our model achieves state-of-the-art results on the DOTA-v1.0, DOTA-v1.5, and HRSC2016 datasets without additional computations or parameters.
更多查看译文
关键词
Sampling equivariance,aerial images,object detection,self-attention
AI 理解论文
溯源树
样例
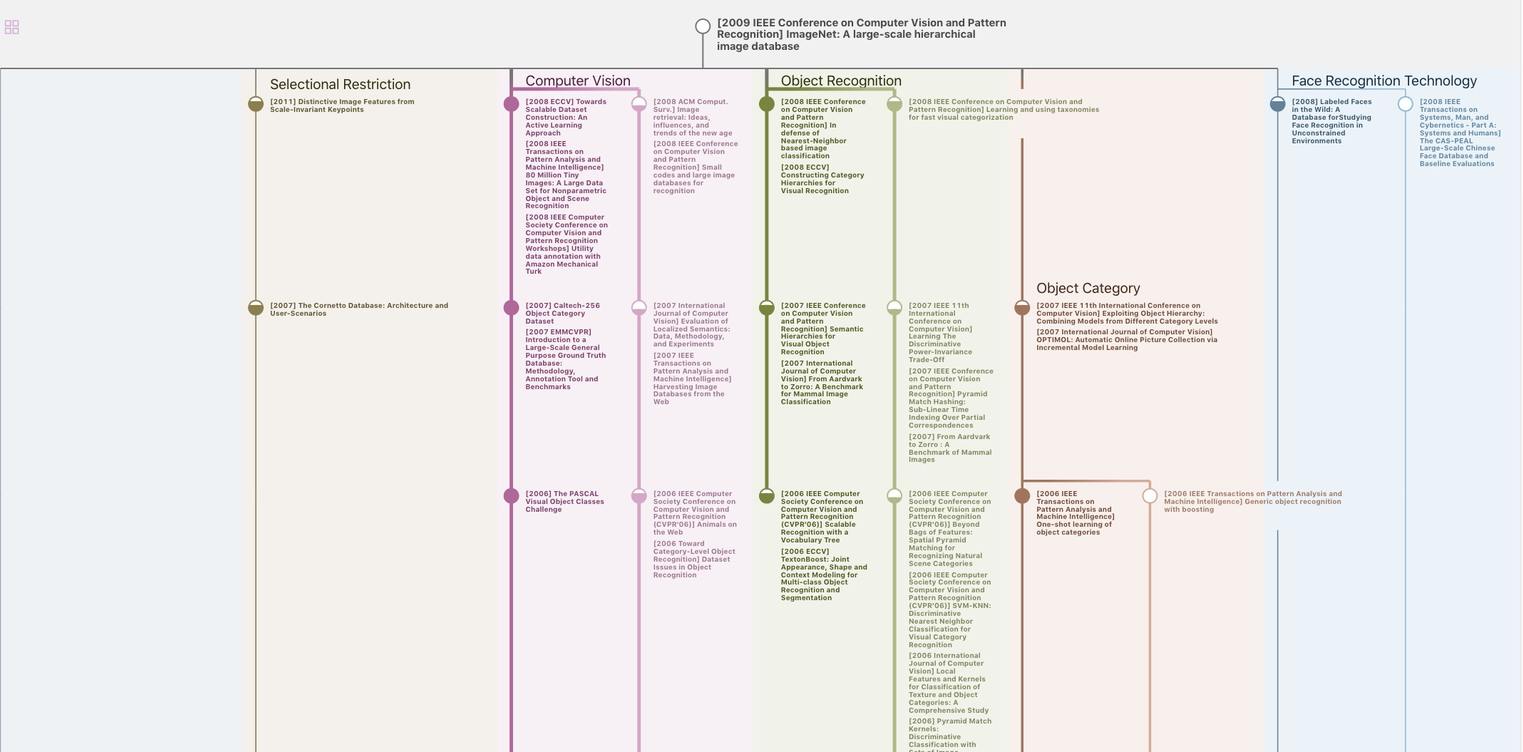
生成溯源树,研究论文发展脉络
Chat Paper
正在生成论文摘要