A Methodology for Training Homomorphic Encryption Friendly Neural Networks
arxiv(2022)
Abstract
Privacy-preserving deep neural network (DNN) inference is a necessity in different regulated industries such as healthcare, finance, and retail. Recently, homomorphic encryption (HE) has been used as a method to enable analytics while addressing privacy concerns. HE enables secure predictions over encrypted data. However, there are several challenges related to the use of HE, including DNN size limitations and the lack of support for some operation types. Most notably, the commonly used ReLU activation is not supported under some HE schemes. We propose a structured methodology to replace ReLU with a quadratic polynomial activation. To address the accuracy degradation issue, we use a pre-trained model that trains another HE-friendly model, using techniques such as 'trainable activation' functions and knowledge distillation. We demonstrate our methodology on the AlexNet architecture, using the chest X-Ray and CT datasets for COVID-19 detection. Experiments using our approach reduced the gap between the F-1 score and accuracy of the models trained with ReLU and the HE-friendly model to within a mere 0.32-5.3% degradation. We also demonstrate our methodology using the SqueezeNet architecture, for which we observed 7% accuracy and F-1 improvements over training similar networks with other HE-friendly training methods.
MoreTranslated text
Key words
Deep learning,Homomorphic encryption,HE-friendly neural networks,DNN training,AlexNet,SqueezeNet
AI Read Science
Must-Reading Tree
Example
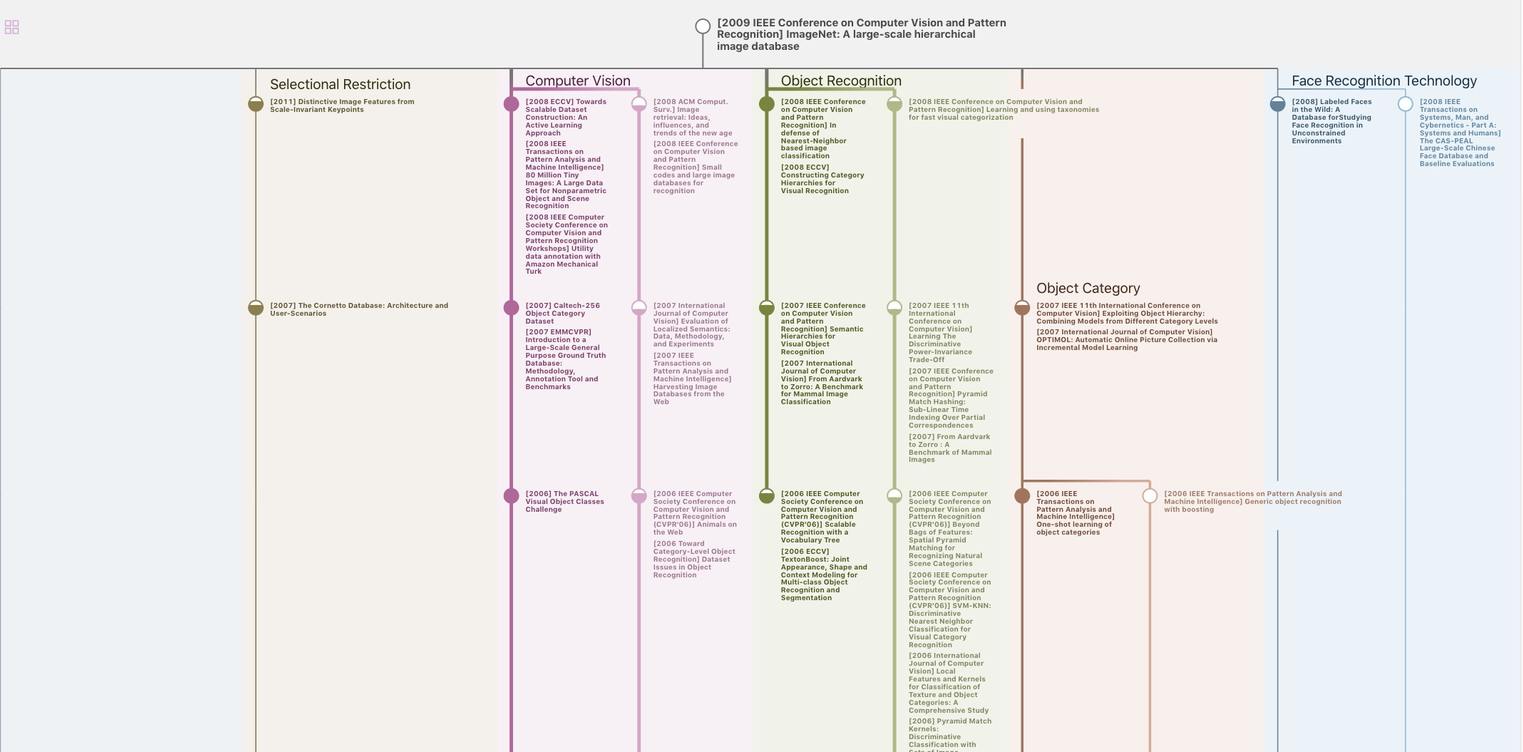
Generate MRT to find the research sequence of this paper
Chat Paper
Summary is being generated by the instructions you defined