Effective Cross-Utterance Language Modeling for Conversational Speech Recognition
arxiv(2022)
摘要
Conversational speech normally is embodied with loose syntactic structures at the utterance level but simultaneously exhibits topical coherence relations across consecutive utterances. Prior work has shown that capturing longer context information with a recurrent neural network or long short-term memory language model (LM) may suffer from the recent bias while excluding the long-range context. In order to capture the long-term semantic interactions among words and across utterances, we put forward disparate conversation history fusion methods for language modeling in automatic speech recognition (ASR) of conversational speech. Furthermore, a novel audio-fusion mechanism is introduced, which manages to fuse and utilize the acoustic embeddings of a current utterance and the semantic content of its corresponding conversation history in a cooperative way. To flesh out our ideas, we frame the ASR N-best hypothesis rescoring task as a prediction problem, leveraging BERT, an iconic pre-trained LM, as the ingredient vehicle to facilitate selection of the oracle hypothesis from a given N-best hypothesis list. Empirical experiments conducted on the AMI benchmark dataset seem to demonstrate the feasibility and efficacy of our methods in relation to some current top-of-line methods. The proposed methods not only achieve significant inference time reduction but also improve the ASR performance for conversational speech.
更多查看译文
关键词
language modeling,conversational speech,automatic speech recognition,N-best hypothesis reranking
AI 理解论文
溯源树
样例
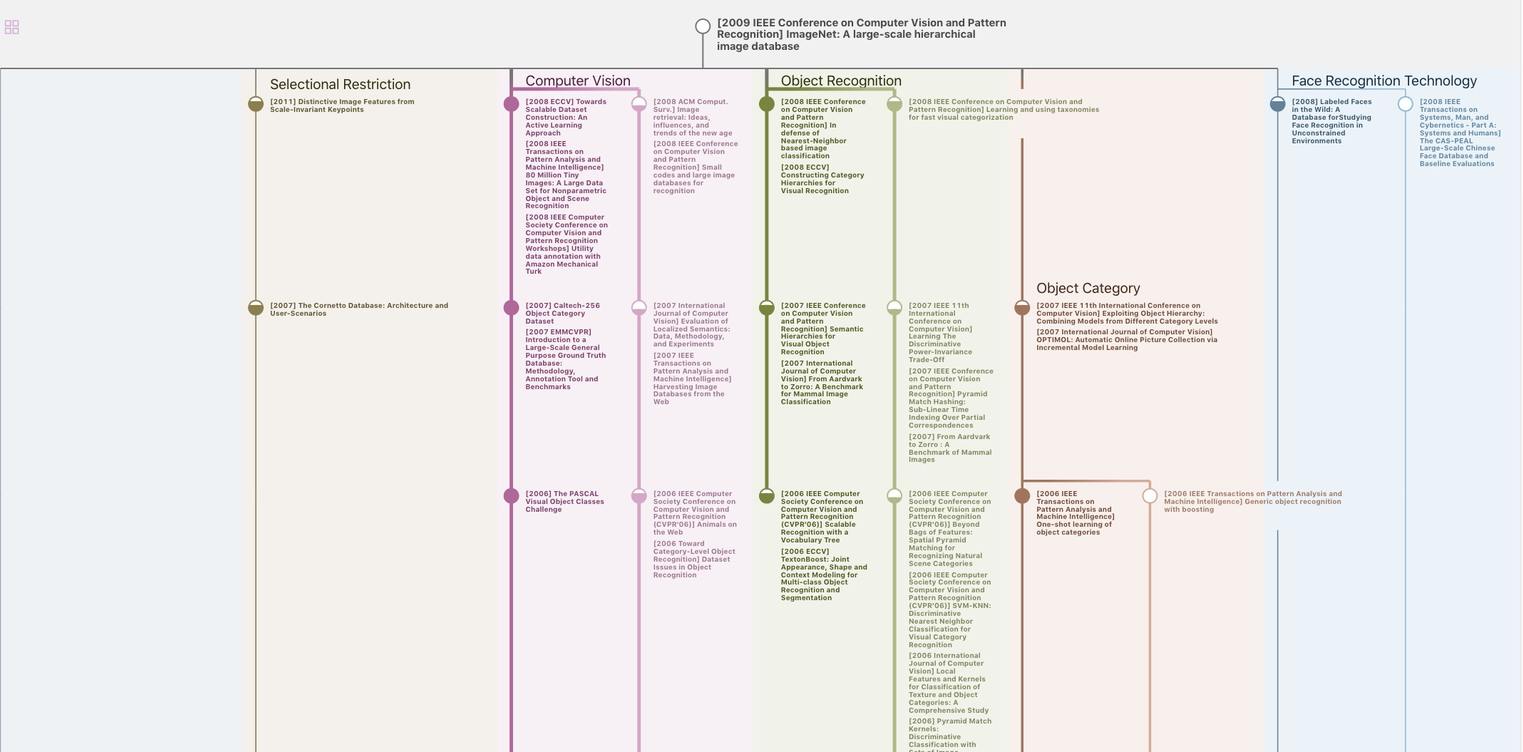
生成溯源树,研究论文发展脉络
Chat Paper
正在生成论文摘要