Interpretable Personalized Experimentation
ACM SIGKDD Conference on Knowledge Discovery and Data Mining (KDD)(2022)
Abstract
Black-box heterogeneous treatment effect (HTE) models are increasingly being used to create personalized policies that assign individuals to their optimal treatments. However, they are difficult to understand, and can be burdensome to maintain in a production environment. In this paper, we present a scalable, interpretable personalized experimentation system, implemented and deployed in production at Meta. The system works in a multiple treatment, multiple outcome setting typical at Meta to: (1) learn explanations for black-box HTE models; (2) generate interpretable personalized policies. We evaluate the methods used in the system on publicly available data and Meta use cases, and discuss lessons learnt during the development of the system.
MoreTranslated text
Key words
heterogeneous treatment effects,personalization,interpretability
AI Read Science
Must-Reading Tree
Example
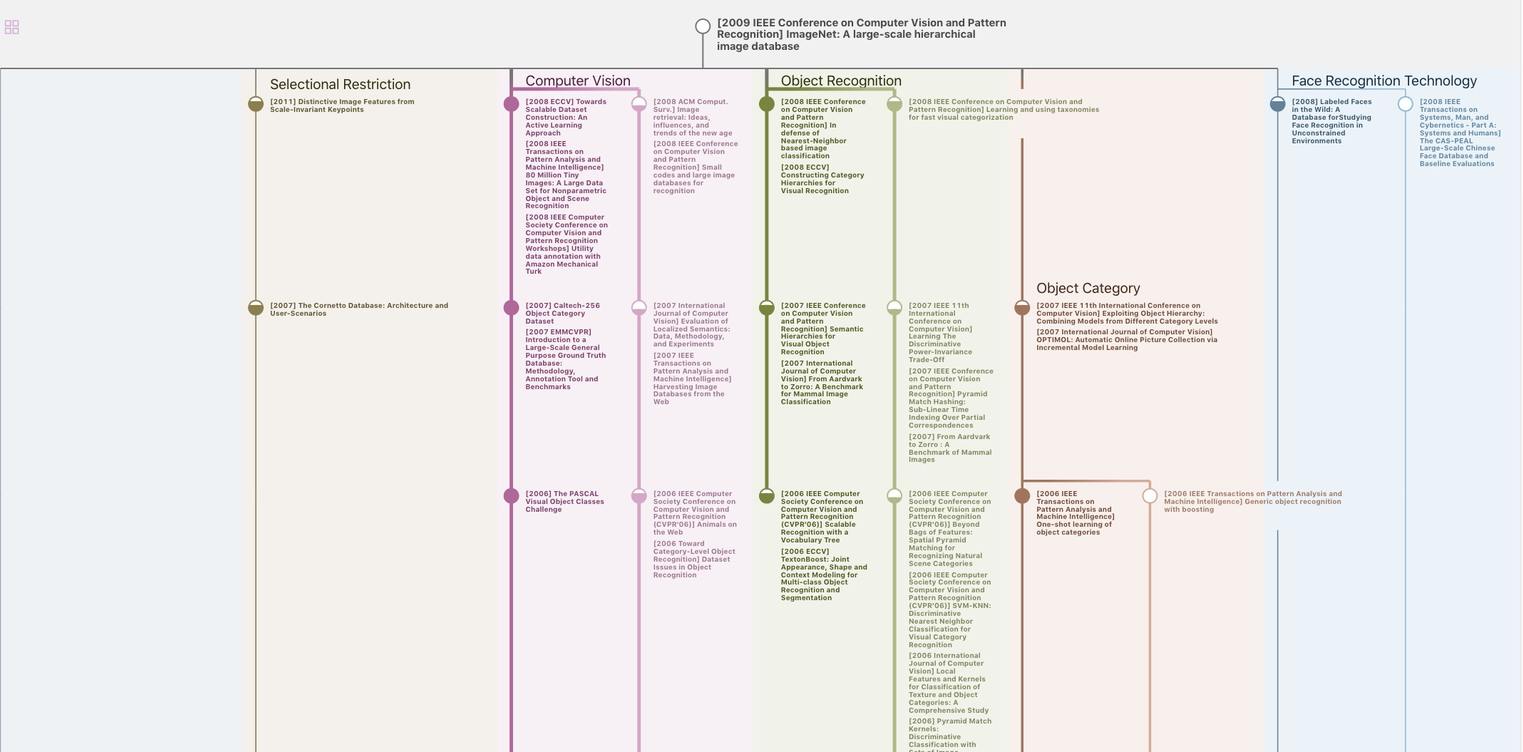
Generate MRT to find the research sequence of this paper
Chat Paper
Summary is being generated by the instructions you defined