LiDAR-based 3D Video Object Detection with Foreground Context Modeling and Spatiotemporal Graph Reasoning.
ITSC(2021)
摘要
The strong demand for autonomous driving in the industry has promoted researches on 3D object detection algorithms. However, the vast majority of algorithms use a single-frame detection diagram, ignoring the spatiotemporal correlations across the point cloud frames. In this work, a novel Foreground Context Modeling Block (FCMB) is proposed to model the foreground spatial context and channel-wise dependency of point cloud features which maintains the original inference speed. Besides, to explore the information of multiple frames, we design a two-stage Spatial-Temporal Graph Neural Network (STGNN). In STGNN, the first stage consumes the coarse proposals of each point cloud frame and conducts intra-frame proposals refinement by massage update functions. And the second stage performs multiple graph convolutions based on the similarity graph to aggregate the semantically similar objects across the input frames. Experimental results show that our 3D video object detector outperforms the LiDAR-based state-of-the-art (SOTA) models on the nuScenes benchmark.
更多查看译文
关键词
STGNN,point cloud frame,conducts intra-frame proposals refinement,multiple graph convolutions,similarity graph,semantically similar objects,input frames,3D video object detector,LiDAR-based state-of-the-art models,LiDAR-based 3D video object detection,autonomous driving,3D object detection algorithms,single-frame detection diagram,spatiotemporal correlations,point cloud frames,novel Foreground Context Modeling Block,foreground spatial context,channel-wise dependency,point cloud features,original inference speed,two-stage Spatial-Temporal Graph Neural Network
AI 理解论文
溯源树
样例
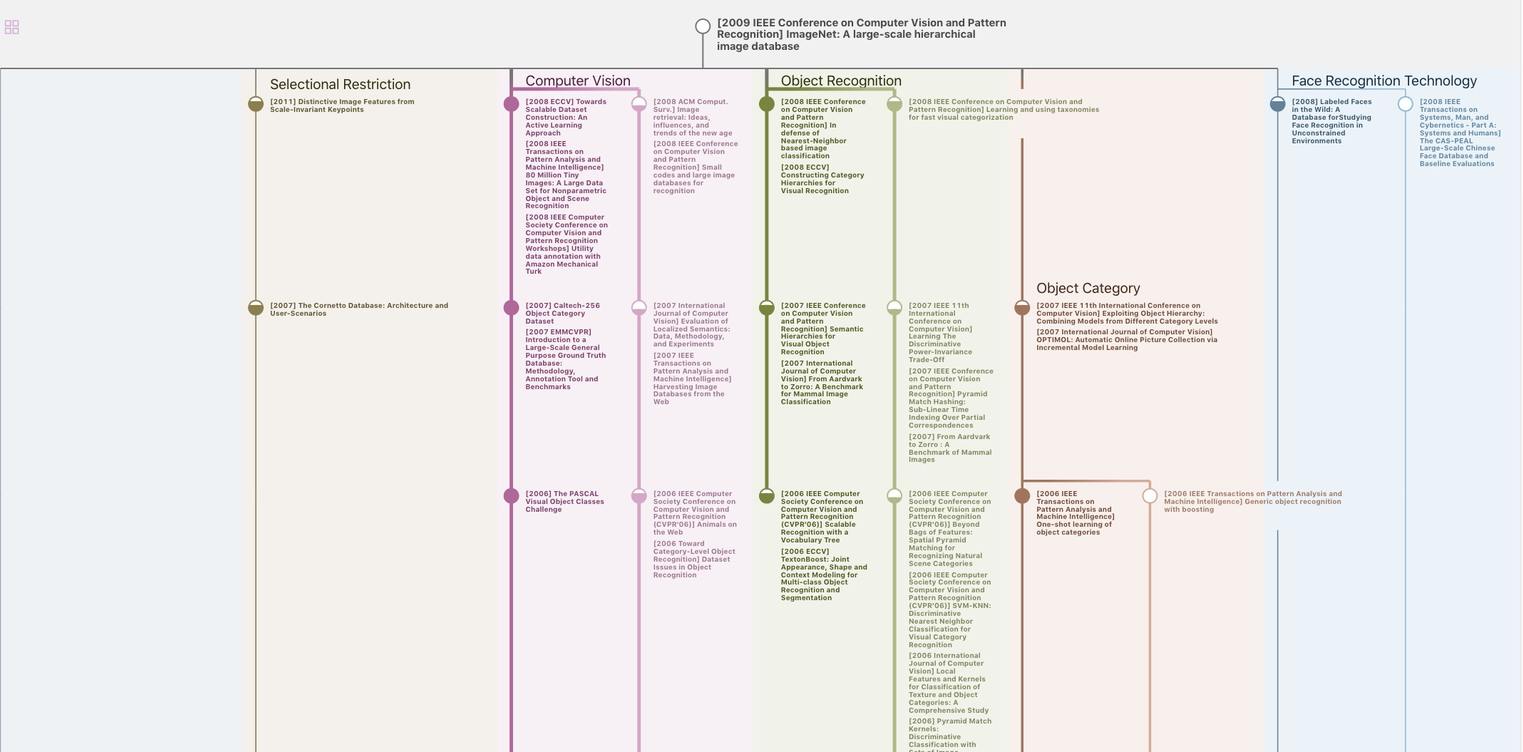
生成溯源树,研究论文发展脉络
Chat Paper
正在生成论文摘要