Prediction Of Consumer Preference For The Bottom Of The Pyramid Using Eeg-Based Deep Model
INTERNATIONAL JOURNAL OF COMPUTATIONAL SCIENCE AND ENGINEERING(2021)
摘要
Emotion detection using electroencephalogram (EEG) signals has gained widespread acceptance in consumer preference study. It has been observed that emotion classification using brain signals has great potential over rating-based quantitative analysis. In the consumer segment, the bottom of the pyramid (BoP) people have also been considered an essential consumer base. This study aims to classify consumer preferences while visualising advertisements for BoP consumers. Four types of consumer preferences (most like, like, dislike, most dislike) have been classified while visualising different advertisements. A robust long short-term memory (LSTM)-based deep neural network model has been developed for classifying consumer preferences using the EEG signal. The proposed model has achieved 94.18% classification accuracy. The proposed model has attained a significant improvement of 11.71% and 3.24% in terms of classification accuracy over other machine learning classifiers (support vector machine and random forest), respectively. This study aims to add a significant contribution to the research domain of consumer behaviour, as it provides a guideline about the consumer preferences of the BoPs after seeing the online advertisements.
更多查看译文
关键词
neuromarketing, deep learning, electroencephalogram, EEG, bottom of the pyramid, BoP, consumer behaviour
AI 理解论文
溯源树
样例
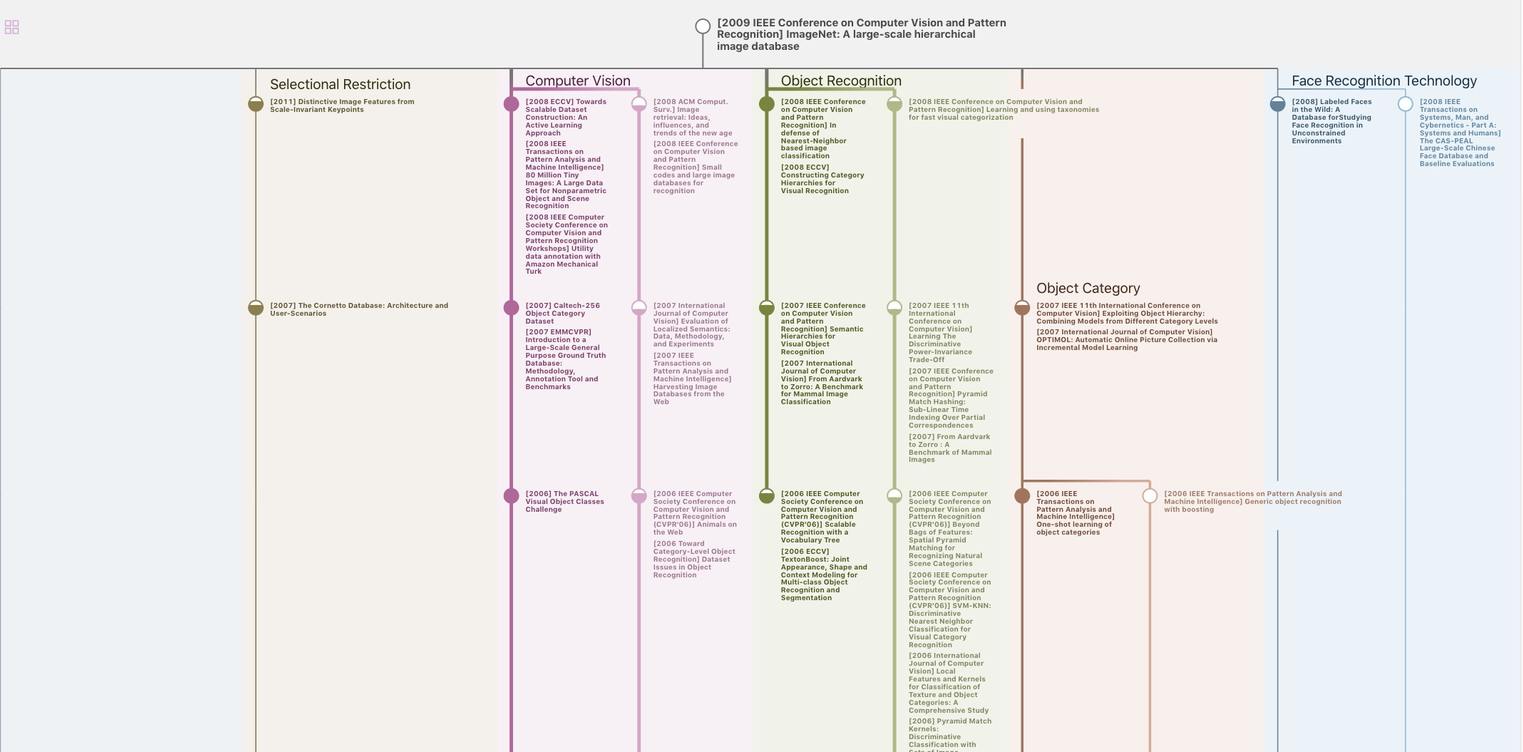
生成溯源树,研究论文发展脉络
Chat Paper
正在生成论文摘要