A machine learning-based approach for quantitative grading of vesicoureteral reflux from voiding cystourethrograms: Methods and proof of concept
Journal of Pediatric Urology(2022)
摘要
Introduction The objectivity of vesicoureteral reflux (VUR) grading has come into question for low inter-rater reliability. Using quantitative image features to aid in VUR grading may make it more consistent. Objective To develop a novel quantitative approach to the assignment of VUR from voiding cystourethrograms (VCUG) alone. Study design An online dataset of VCUGs was abstracted and individual renal units were graded as low-grade (I-III) or high-grade (IV-V). We developed an image analysis and machine learning workflow to automatically calculate and normalize the ureteropelvic junction (UPJ) width, ureterovesical junction (UVJ) width, maximum ureter width, and tortuosity of the ureter based on three simple user annotations. A random forest classifier was trained to distinguish between low-vs high-grade VUR. An external validation cohort was generated from the institutional imaging repository. Discriminative capability was quantified using receiver-operating-characteristic and precision-recall curve analysis. We used Shapley Additive exPlanations to interpret the model's predictions. Results 41 renal units were abstracted from an online dataset, and 44 renal units were collected from the institutional imaging repository. Significant differences observed in UVJ width, UPJ width, maximum ureter width, and tortuosity between low- and high-grade VUR. A random-forest classifier performed favourably with an accuracy of 0.83, AUROC of 0.90 and AUPRC of 0.89 on leave-one-out cross-validation, and accuracy of 0.84, AUROC of 0.88 and AUPRC of 0.89 on external validation. Tortuosity had the highest feature importance, followed by maximum ureter width, UVJ width, and UPJ width. We deployed this tool as a web-application, qVUR (quantitative VUR), where users are able to upload any VCUG for automated grading using the model generated here (https://akhondker.shinyapps.io/ qVUR/). Discussion This study provides the first step towards creating an automated and more objective standard for determining the significance of VUR features. Our findings suggest that tortuosity and ureter dilatation are predictors of high-grade VUR. Moreover, this proof-of-concept model was deployed in a simple-to-use web application. Conclusion Grading of VUR using quantitative metrics is possible, even in non-standardized datasets of VCUG. Machine learning methods can be applied to objectively grade VUR in the future. [GRAPHICS]
更多查看译文
关键词
Vesicoureteral reflux,Voiding cystourethrogram,Machine learning,Explainable artificial intelligence
AI 理解论文
溯源树
样例
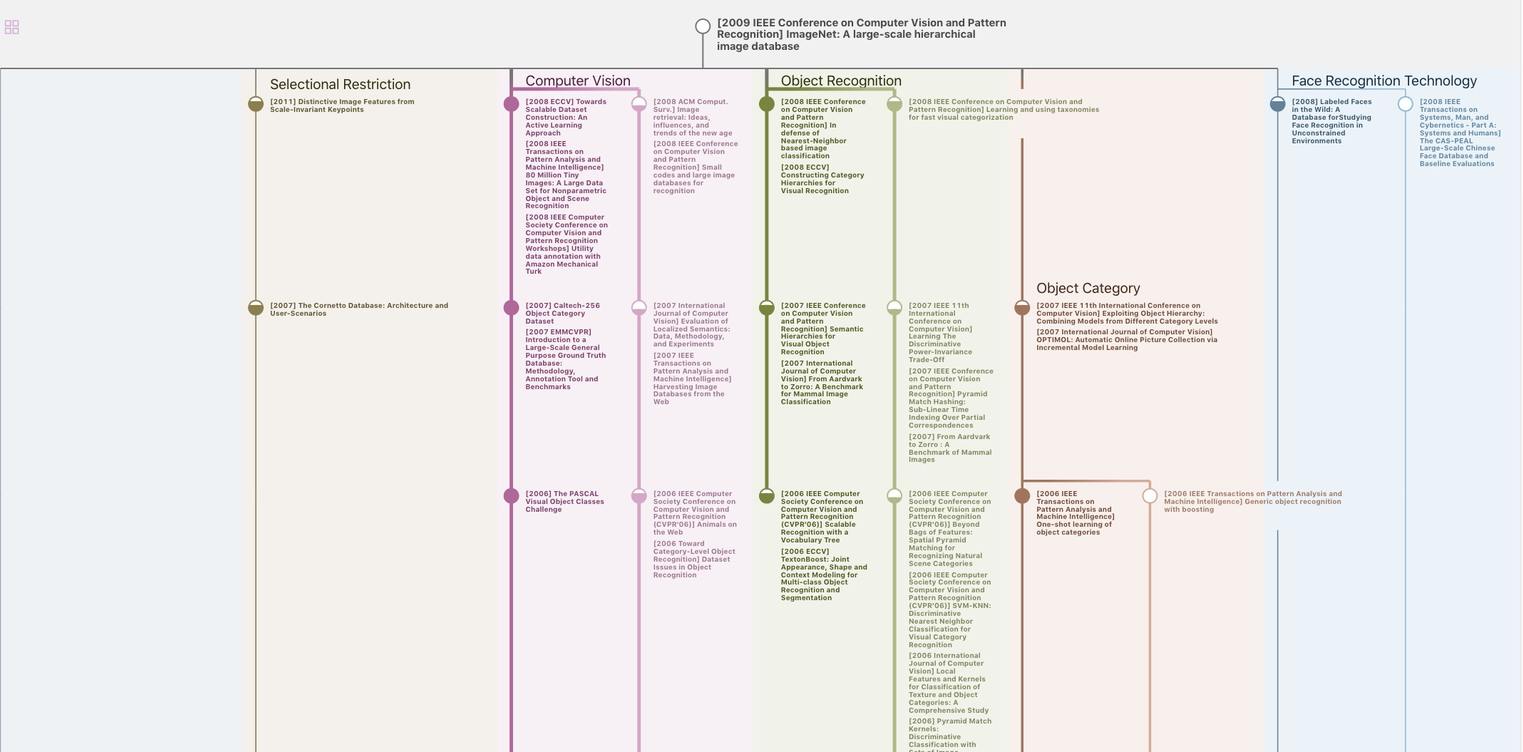
生成溯源树,研究论文发展脉络
Chat Paper
正在生成论文摘要