A Multi-objective Structure Variant Bacterial Heuristic Feature Selection Method in High-dimensional Data Classification.
DMBD(2021)
Abstract
Feature selection (FS) has been studied as a multi-objective problem in recent years to improve the classification effect. In this paper, to expand the ability of Bacterial Foraging Optimization (BFO) in feature selection problems for classification, a Multi-objective Adapting Chemotaxis Bacterial Foraging Optimization (abbreviated as MOACBFO) is proposed. In MOACBFO, a structural variation strategic model is proposed to reduce the computational complexity for multi-objective problems and applied with a dynamically updated external matrix to record the performance of bacteria on two objectives. In addition, an adaptive chemotaxis step mechanism is designed to help the bacteria jump out of the local optimality. To further enhance the diversity of bacteria searching capability, a feature subset updating strategy is developed. The optimal feature subset and fitness value are stored in the external matrix continuously by comparing them with the historical value records. The performance of the proposed algorithm is demonstrated by comparing it with four other advanced swarm intelligent algorithms on 11 high-dimensional microarray datasets. The results indicate that the MOACBFO performs better in achieving a lower classification error rate using a small number of features.
MoreTranslated text
Key words
feature selection,classification,multi-objective,high-dimensional
AI Read Science
Must-Reading Tree
Example
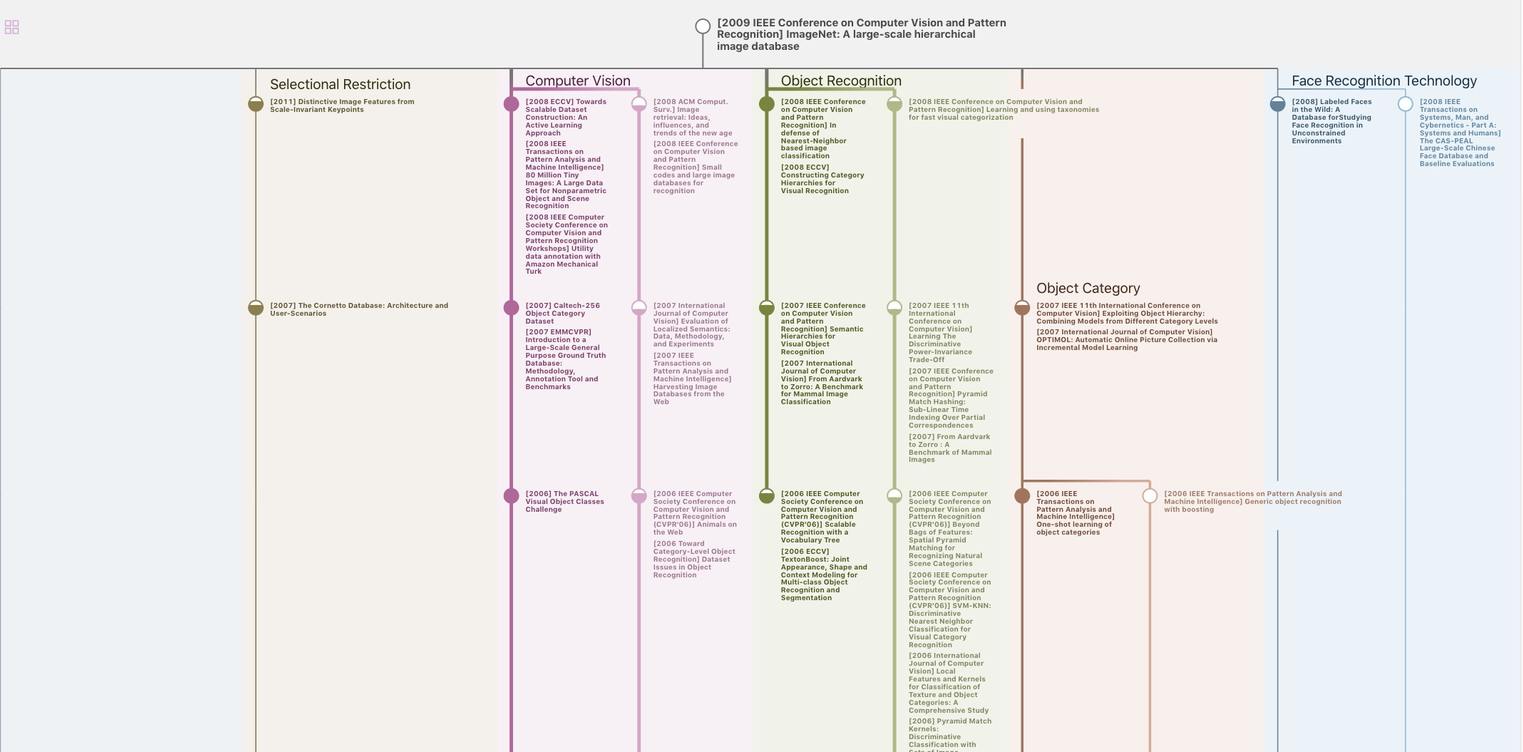
Generate MRT to find the research sequence of this paper
Chat Paper
Summary is being generated by the instructions you defined