Leveraging Domain Information to Classify Financial Documents via Unsupervised Graph Momentum Contrast
Conference on Information and Knowledge Management(2021)
摘要
BSTRACTFinancial documents often contain rich domain information, such as named entities, which could be used to indicate the documents' classification categories. Existing classification methods either ignore such contained financial domain information, achieving less optimal performances, or train document representations in supervised ways, with expensive data labeling costs. In this paper, we propose to leverage domain information to improve classification performance for financial documents, via a graph representation learning model, namely G-MoCo, based on unsupervised graph momentum contrast. With G-MoCo, we could extract latent features from massive unlabeled raw data, and then further use the learned representations for document classification. Compared with the state-of-the-art baselines, representations learned by our method could improve performances by significant margins on a financial document dataset and three non-financial public graph datasets.
更多查看译文
AI 理解论文
溯源树
样例
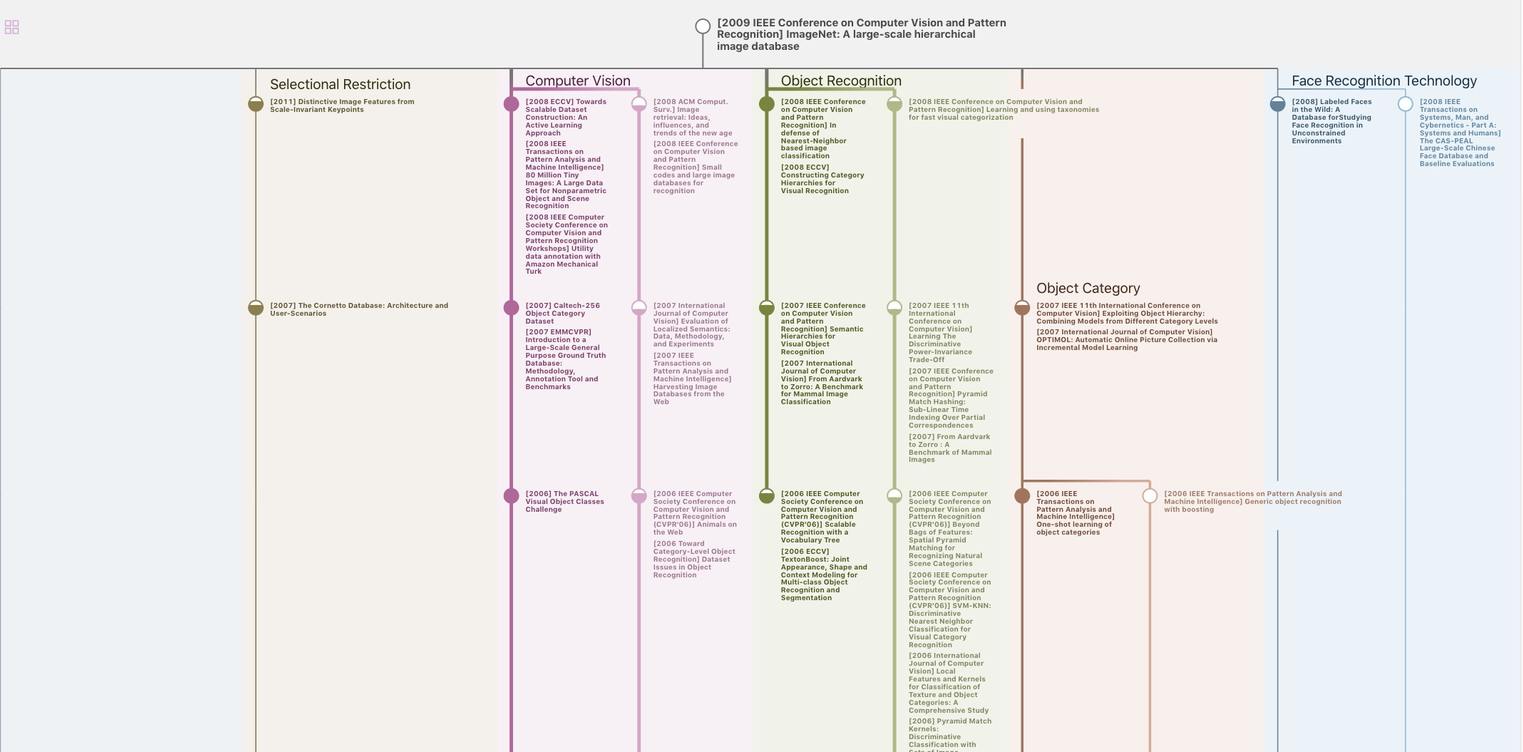
生成溯源树,研究论文发展脉络
Chat Paper
正在生成论文摘要