Norma: A Hybrid Feature Alignment for Class-Aware Unsupervised Domain Adaptation
Conference on Information and Knowledge Management(2021)
摘要
ABSTRACTUnsupervised domain adaptation is the problem of transferring extracted knowledge from a labeled source domain to an unlabeled target domain. To achieve discriminative domain adaptation recent studies take advantage of target sample pseudo-labels to impose class-aware distribution alignment across the source and target domains. Still, they have some shortcomings such as making decisions based on inaccurate pseudo-labeled samples that mislead the adaptation process. In this paper, we propose a progressive deep feature alignment, called Norma, to tackle class-aware unsupervised domain adaptation for image classification by enforcing inter-class compactness and intra-class discrepancy through a hybrid learning process. To this end, Norma's optimization process is defined based on a novel triplet loss which not only addresses soft prototype alignment but also pushes away multiple negative centroids. Also, to extract maximum discriminative domain knowledge per iteration, we propose a joint positive and negative learning procedure along with an uncertainty-guided progressive pseudo-labeling on the basis of prototype-based clustering and conditional probability. Our experimental results on several benchmarks demonstrate that Norma outperforms the state-of-the-art methods.
更多查看译文
AI 理解论文
溯源树
样例
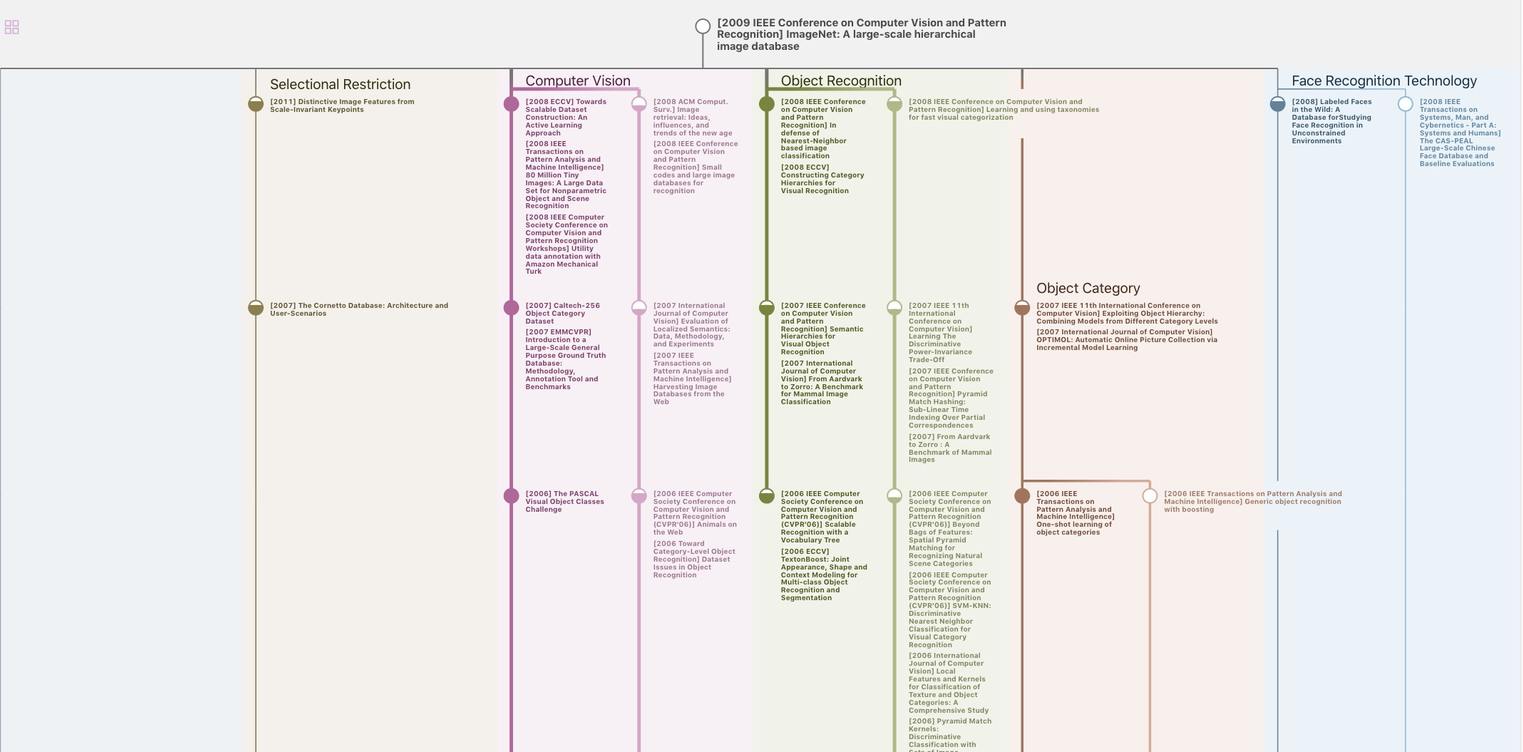
生成溯源树,研究论文发展脉络
Chat Paper
正在生成论文摘要