MRI-Based Machine Learning in Differentiation Between Benign and Malignant Breast Lesions
FRONTIERS IN ONCOLOGY(2021)
摘要
Background Differential diagnosis between benign and malignant breast lesions is of crucial importance relating to follow-up treatment. Recent development in texture analysis and machine learning may lead to a new solution to this problem. Method This current study enrolled a total number of 265 patients (benign breast lesions:malignant breast lesions = 71:194) diagnosed in our hospital and received magnetic resonance imaging between January 2014 and August 2017. Patients were randomly divided into the training group and validation group (4:1), and two radiologists extracted their texture features from the contrast-enhanced T1-weighted images. We performed five different feature selection methods including Distance correlation, Gradient Boosting Decision Tree (GBDT), least absolute shrinkage and selection operator (LASSO), random forest (RF), eXtreme gradient boosting (Xgboost) and five independent classification models were built based on Linear discriminant analysis (LDA) algorithm. Results All five models showed promising results to discriminate malignant breast lesions from benign breast lesions, and the areas under the curve (AUCs) of receiver operating characteristic (ROC) were all above 0.830 in both training and validation groups. The model with a better discriminating ability was the combination of LDA + gradient boosting decision tree (GBDT). The sensitivity, specificity, AUC, and accuracy in the training group were 0.814, 0.883, 0.922, and 0.868, respectively; LDA + random forest (RF) also suggests promising results with the AUC of 0.906 in the training group. Conclusion The evidence of this study, while preliminary, suggested that a combination of MRI texture analysis and LDA algorithm could discriminate benign breast lesions from malignant breast lesions. Further multicenter researches in this field would be of great help in the validation of the result.
更多查看译文
关键词
linear discriminant analysis, differential diagnosis, machine learning, breast lesion, texture analysis, MRI
AI 理解论文
溯源树
样例
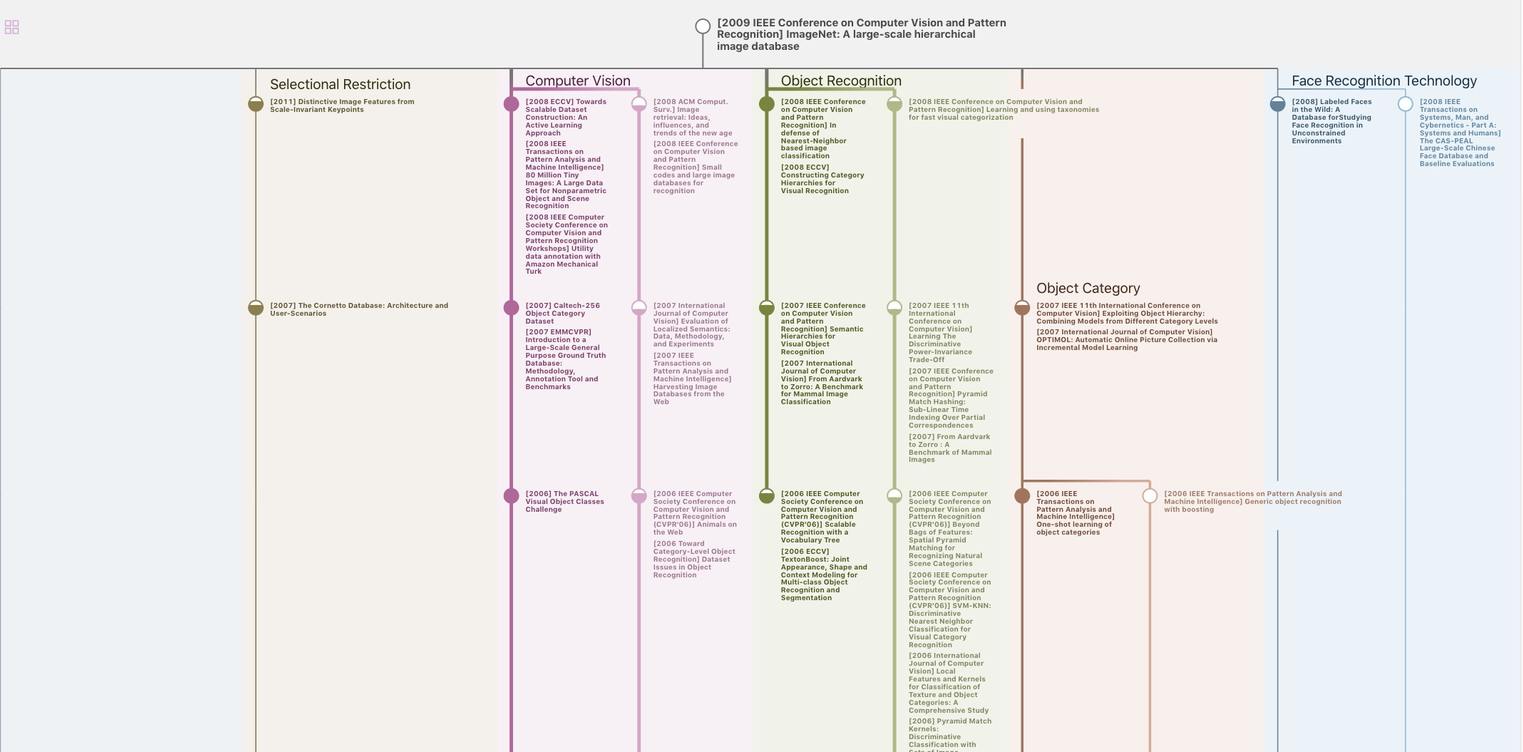
生成溯源树,研究论文发展脉络
Chat Paper
正在生成论文摘要