Self-Supervised Radio-Visual Representation Learning for 6G Sensing
IEEE International Conference on Communications (ICC)(2022)
摘要
In future 6G cellular networks, a joint communication and sensing protocol will allow the network to perceive the environment, opening the door for many new applications atop a unified communication-perception infrastructure. However, interpreting the sparse radio representation of sensing scenes is challenging, which hinders the potential of these emergent systems. We propose to combine radio and vision to automatically learn a radio-only sensing model with minimal human intervention. We want to build a radio sensing model that can feed on millions of uncurated data points. To this end, we leverage recent advances in self-supervised learning and formulate a new label-free radio-visual co-learning scheme, whereby vision trains radio via cross-modal mutual information. We implement and evaluate our scheme according to the common linear classification benchmark, and report qualitative and quantitative performance metrics. In our evaluation, the representation learnt by radio-visual self-supervision works well for a downstream sensing demonstrator, and outperforms its fully-supervised counterpart when less labelled data is used. This indicates that self-supervised learning could be an important enabler for future scalable radio sensing systems.
更多查看译文
关键词
radio-visual learning,self-supervised learning,deep learning,sensing,perception,6G
AI 理解论文
溯源树
样例
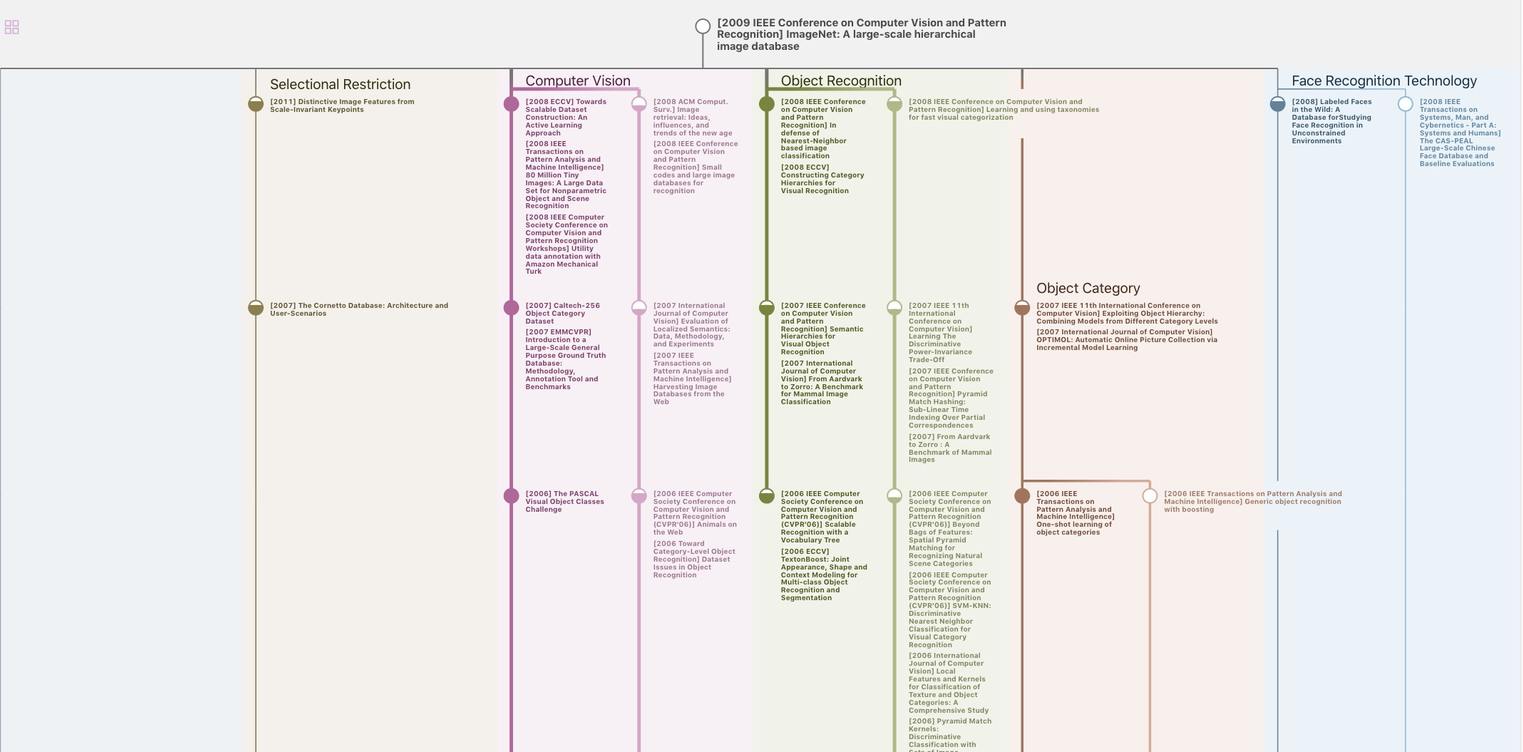
生成溯源树,研究论文发展脉络
Chat Paper
正在生成论文摘要