Efficient continual cohesive subgraph search in large temporal graphs
WORLD WIDE WEB-INTERNET AND WEB INFORMATION SYSTEMS(2021)
摘要
Temporal graphs are equipped with entities and the relationships between entities associated with time stamps. Cohesive subgraph mining (CSM) is a fundamental task in temporal graph analysis, which has gathered great research interests. It benefits from reflecting the dynamism of graphs and has many real-world applications. Yet, most existing work focus on the cohesive subgraph detection (CSD) problem, which identifies all the defined subgraphs in the entire temporal graphs. When graph size becoming too large, it is impractical. In this paper, we are the first to concern about the cohesive subgraph search (CSS) problem in large temporal graphs. In specific, given a query vertex, we are seeking the continual densely connected subgraph including the query vertex. To this end, (1) we model the cohesive subgraph in temporal graphs as a ( 𝜃 , τ )-continual k -core and prove its NP-hardness; (2) we develop two exact algorithms based on different vertex enumeration strategies, called Exact-VD and Exact-VE , respectively. Exact-VD uses depth-first search to find the target subgraphs in a top-down way by gradually deleting vertices from the current subgraph; while Exact-VE starts from the query vertex and continuously expands the ranked vertices in the candidate group until reaching the target subgraphs. Meanwhile, several elegant pruning rules are designed to reduce the search space; (3) to further speed up, we propose an efficient approximate local search method, called Approx-LS , which greedily expands the current subgraph guided by the developed heuristic functions until identifying the results. Comprehensive experiments on four real-life datasets verify the efficiency and effectiveness of our proposed approaches.
更多查看译文
关键词
(𝜃τ)-continual k-core model,Cohesive subgraph search (CSS),Exact and approximate algorithms,Large temporal graphs
AI 理解论文
溯源树
样例
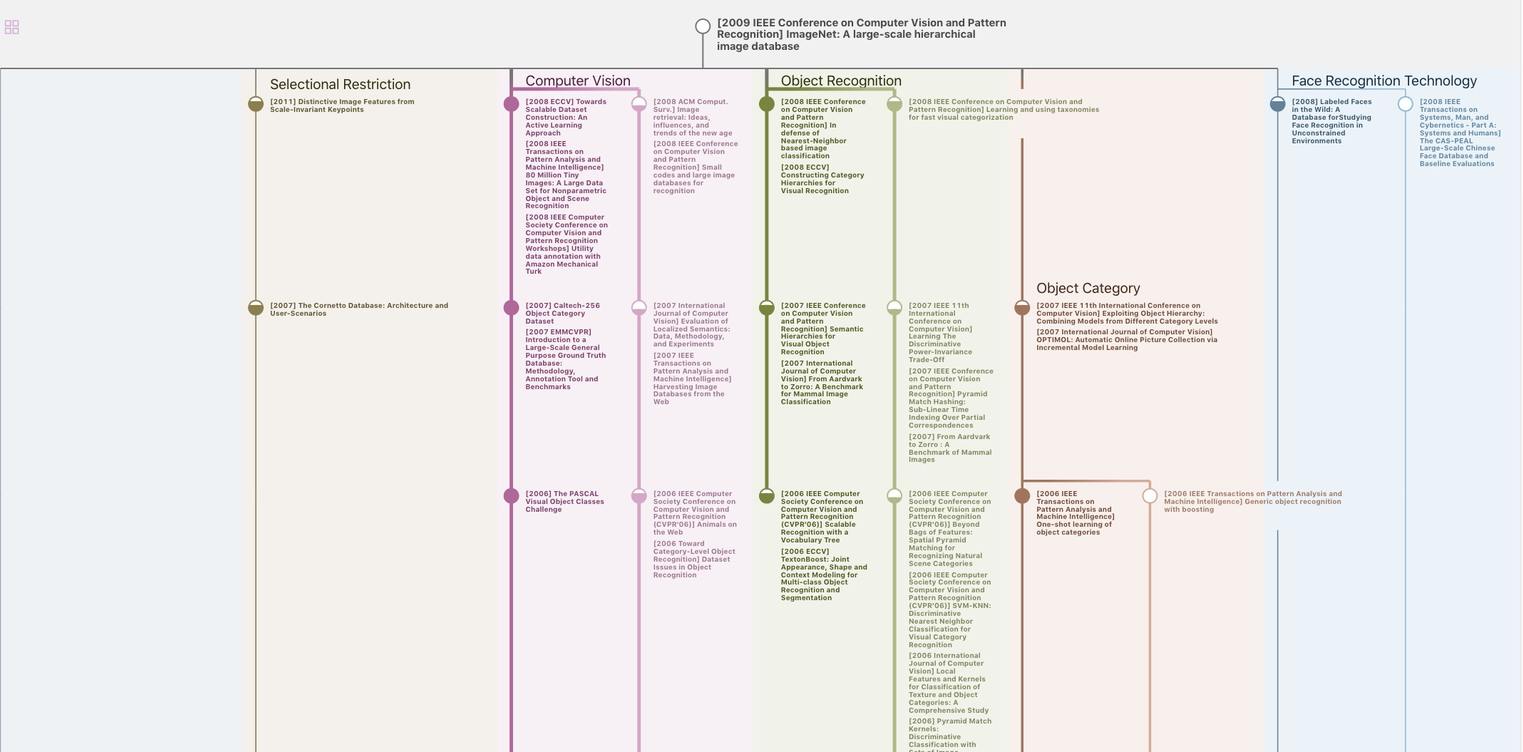
生成溯源树,研究论文发展脉络
Chat Paper
正在生成论文摘要