Explaining anomalies detected by autoencoders using Shapley Additive Explanations
Expert Systems with Applications(2021)
摘要
Deep learning algorithms for anomaly detection, such as autoencoders, point out the outliers, saving experts the time-consuming task of examining normal cases in order to find anomalies. Most outlier detection algorithms output a score for each instance in the database. The top-k most intense outliers are returned to the user for further inspection; however, the manual validation of results becomes challenging without justification or additional clues. An explanation of why an instance is anomalous enables the experts to focus their investigation on the most important anomalies and may increase their trust in the algorithm. Recently, a game theory-based framework known as SHapley Additive exPlanations (SHAP) was shown to be effective in explaining various supervised learning models.
更多查看译文
关键词
Explainable black-box models,XAI,Autoencoder,Shapley values,SHAP,Anomaly detection
AI 理解论文
溯源树
样例
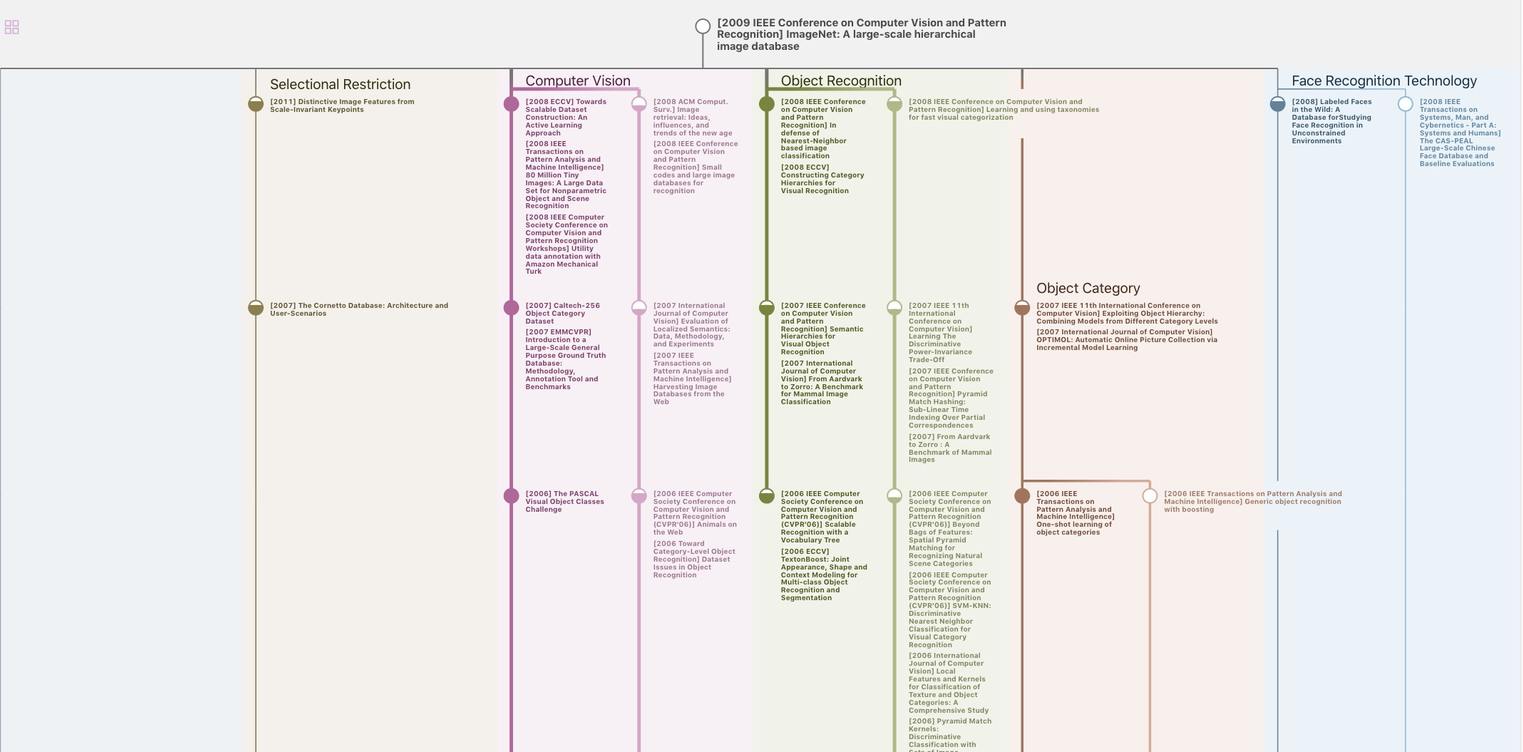
生成溯源树,研究论文发展脉络
Chat Paper
正在生成论文摘要