On learning disentangled representations for individual treatment effect estimation
Journal of Biomedical Informatics(2021)
摘要
•To alleviate the problem of selection bias in individualized treatment effect estimation.•To disentangle outcome-oriented and treatment-oriented factors from input patient features by using representation learning.•To employ an individualized orthogonal regularization to eliminate confounders in the latent representations.•Evaluation is done using a semi-simulated dataset and a real-world datasetfor the task of individualized treatment effect estimation.
更多查看译文
关键词
Individualized treatment effect,Causal inference,Deep learning,Disentangled representation,Auxiliary-task learning,Observational data
AI 理解论文
溯源树
样例
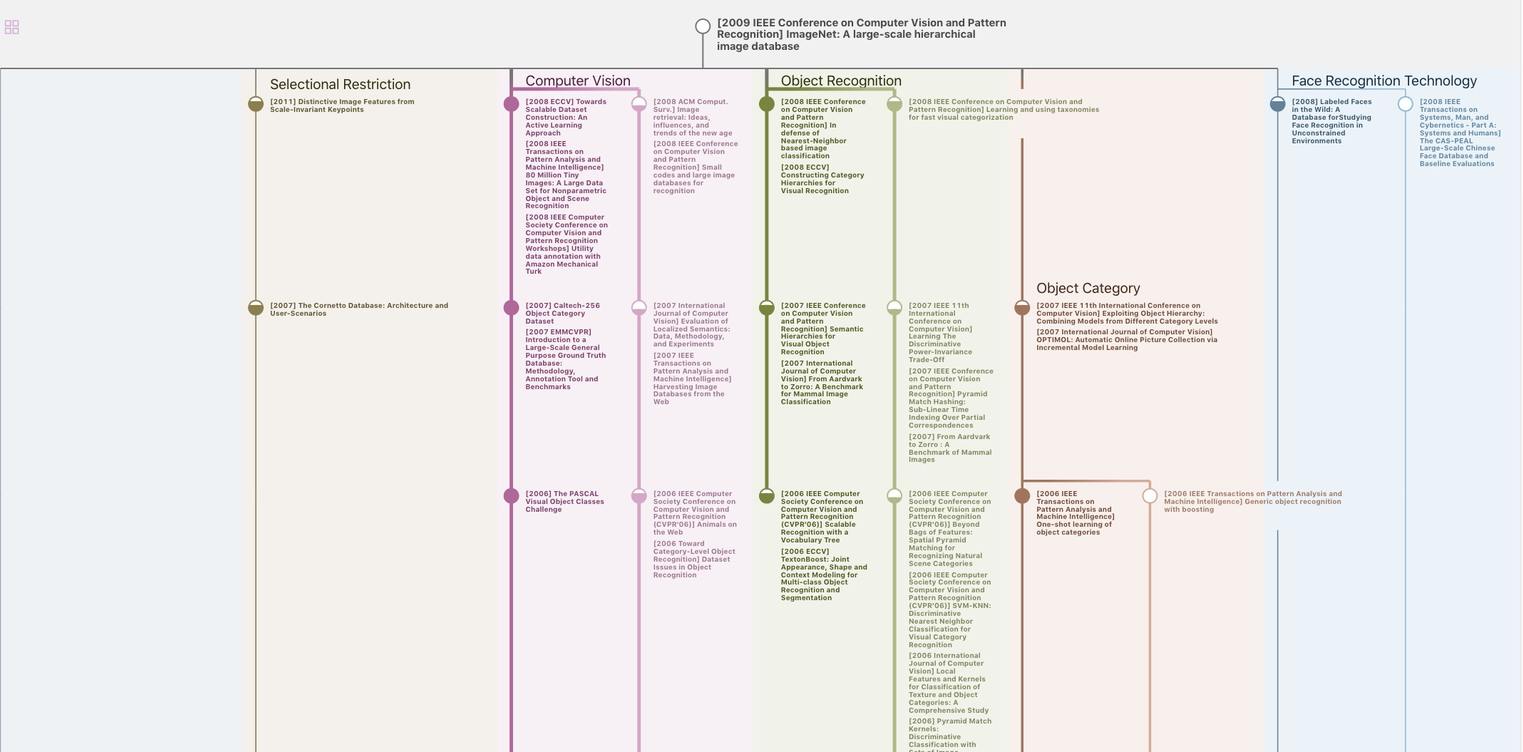
生成溯源树,研究论文发展脉络
Chat Paper
正在生成论文摘要