Towards Sparse Federated Analytics: Location Heatmaps under Distributed Differential Privacy with Secure Aggregation.
Proceedings on Privacy Enhancing Technologies(2022)
摘要
We design a scalable algorithm to privately generate location heatmaps over decentralized data from millions of user devices. It aims to ensure differential privacy before data becomes visible to a service provider while maintaining high data accuracy and minimizing resource consumption on users' devices. To achieve this, we revisit distributed differential privacy based on recent results in secure multiparty computation, and we design a scalable and adaptive distributed differential privacy approach for location analytics. Evaluation on public location datasets shows that this approach successfully generates metropolitan-scale heatmaps from millions of user samples with a worst-case client communication overhead that is significantly smaller than existing state-of-the-art private protocols of similar accuracy.
更多查看译文
关键词
sparse federated analytics,differential privacy,location heatmaps,aggregation
AI 理解论文
溯源树
样例
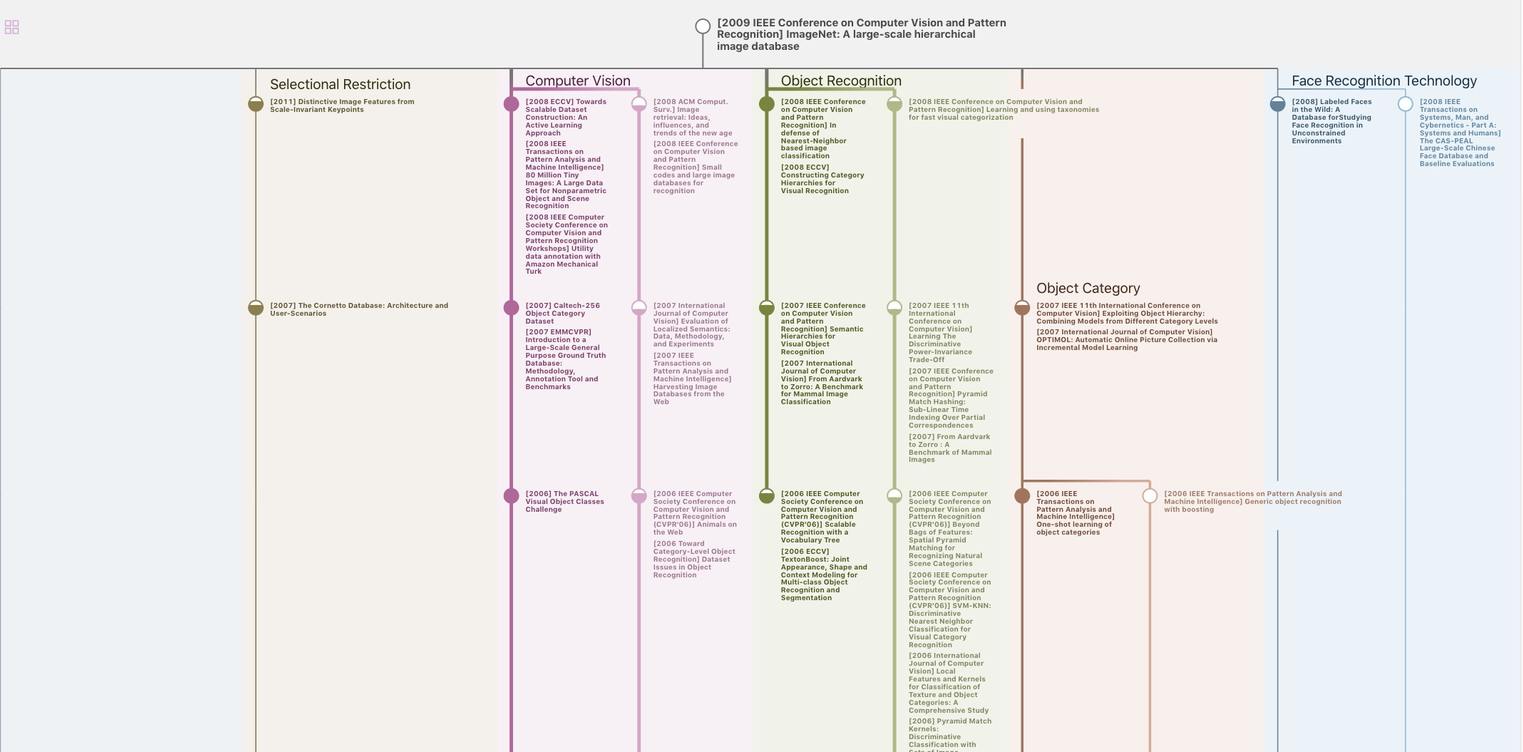
生成溯源树,研究论文发展脉络
Chat Paper
正在生成论文摘要