Causal-BALD: Deep Bayesian Active Learning of Outcomes to Infer Treatment-Effects from Observational Data.
Annual Conference on Neural Information Processing Systems(2021)
摘要
Estimating personalized treatment effects from high-dimensional observational data is essential in situations where experimental designs are infeasible, unethical, or expensive. Existing approaches rely on fitting deep models on outcomes observed for treated and control populations. However, when measuring individual outcomes is costly, as is the case of a tumor biopsy, a sample-efficient strategy for acquiring each result is required. Deep Bayesian active learning provides a framework for efficient data acquisition by selecting points with high uncertainty. However, existing methods bias training data acquisition towards regions of non-overlapping support between the treated and control populations. These are not sample-efficient because the treatment effect is not identifiable in such regions. We introduce causal, Bayesian acquisition functions grounded in information theory that bias data acquisition towards regions with overlapping support to maximize sample efficiency for learning personalized treatment effects. We demonstrate the performance of the proposed acquisition strategies on synthetic and semi-synthetic datasets IHDP and CMNIST and their extensions, which aim to simulate common dataset biases and pathologies.
更多查看译文
关键词
deep bayesian active learning,outcomes,causal-bald,treatment-effects
AI 理解论文
溯源树
样例
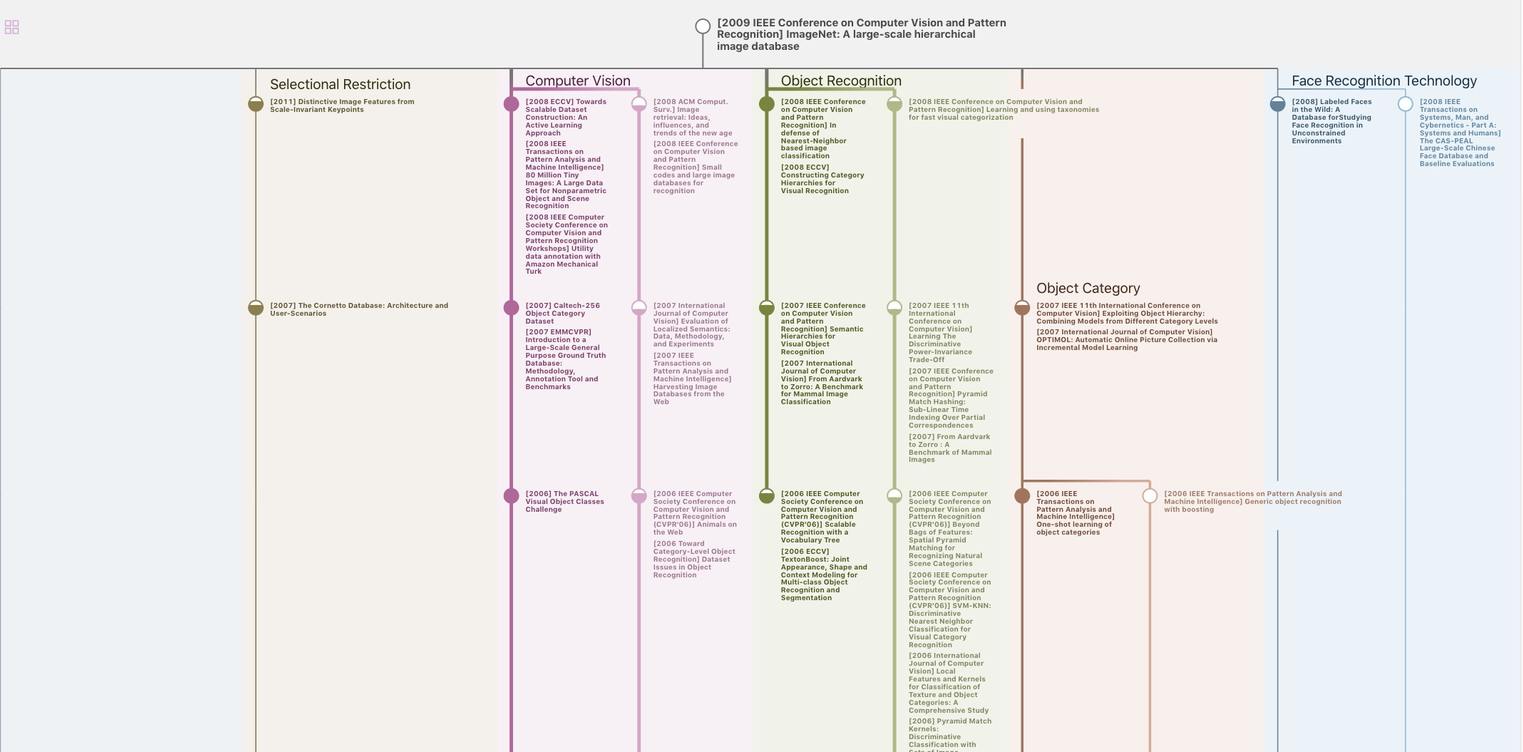
生成溯源树,研究论文发展脉络
Chat Paper
正在生成论文摘要