An Entropy-Guided Reinforced Partial Convolutional Network for Zero-Shot Learning
IEEE Transactions on Circuits and Systems for Video Technology(2022)
摘要
Zero-Shot Learning (ZSL) aims to transfer learned knowledge from observed classes to unseen classes via semantic correlations. A promising strategy is to learn a global-local representation that incorporates global information with extra localities (i.e., small parts/regions of inputs). However, existing methods discover localities based on explicit features without digging into the inherent properties and relationships among regions. In this work, we propose a novel Entropy-guided Reinforced Partial Convolutional Network (ERPCNet), which extracts and aggregates localities progressively based on semantic relevance and visual correlations without human-annotated regions. ERPCNet uses reinforced partial convolution and entropy guidance; it not only discovers global-cooperative localities dynamically but also converges faster for policy gradient optimization. We conduct extensive experiments to demonstrate ERPCNet's performance through comparisons with state-of-the-art methods under ZSL and Generalized Zero-Shot Learning (GZSL) settings on four benchmark datasets. We also show that ERPCNet is time efficient and explainable through visualization analysis.
更多查看译文
关键词
Convolution,Feature extraction,Semantics,Visualization,Training,Optimization,Kernel,Zero-shot learning,reinforcement learning,image representation
AI 理解论文
溯源树
样例
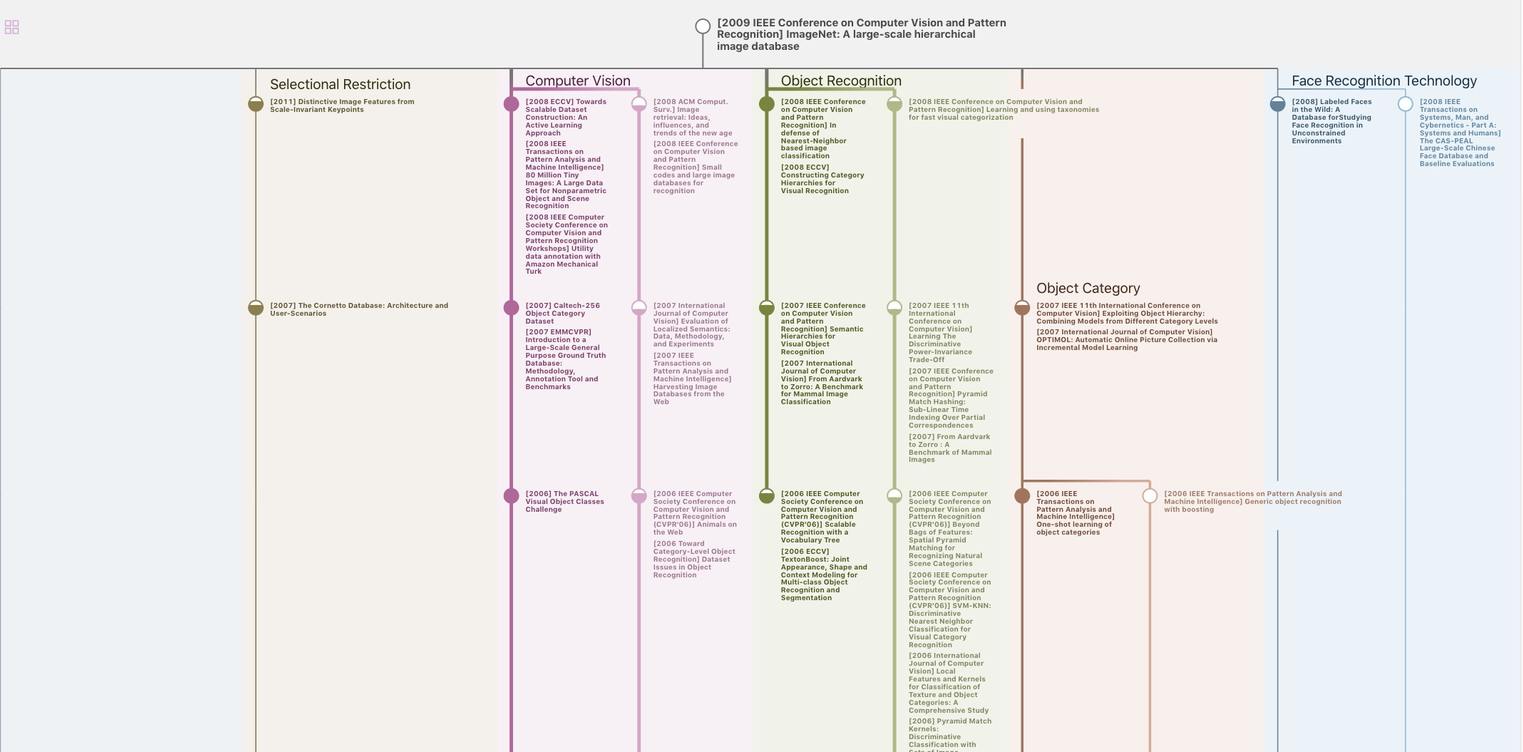
生成溯源树,研究论文发展脉络
Chat Paper
正在生成论文摘要