Scalable mixed-domain Gaussian processes
arxiv(2021)
摘要
Gaussian process (GP) models that combine both categorical and continuous input variables have found use e.g. in longitudinal data analysis and computer experiments. However, standard inference for these models has the typical cubic scaling, and common scalable approximation schemes for GPs cannot be applied since the covariance function is non-continuous. In this work, we derive a basis function approximation scheme for mixed-domain covariance functions, which scales linearly with respect to the number of observations and total number of basis functions. The proposed approach is naturally applicable to Bayesian GP regression with arbitrary observation models. We demonstrate the approach in a longitudinal data modelling context and show that it approximates the exact GP model accurately, requiring only a fraction of the runtime compared to fitting the corresponding exact model.
更多查看译文
关键词
processes,mixed-domain
AI 理解论文
溯源树
样例
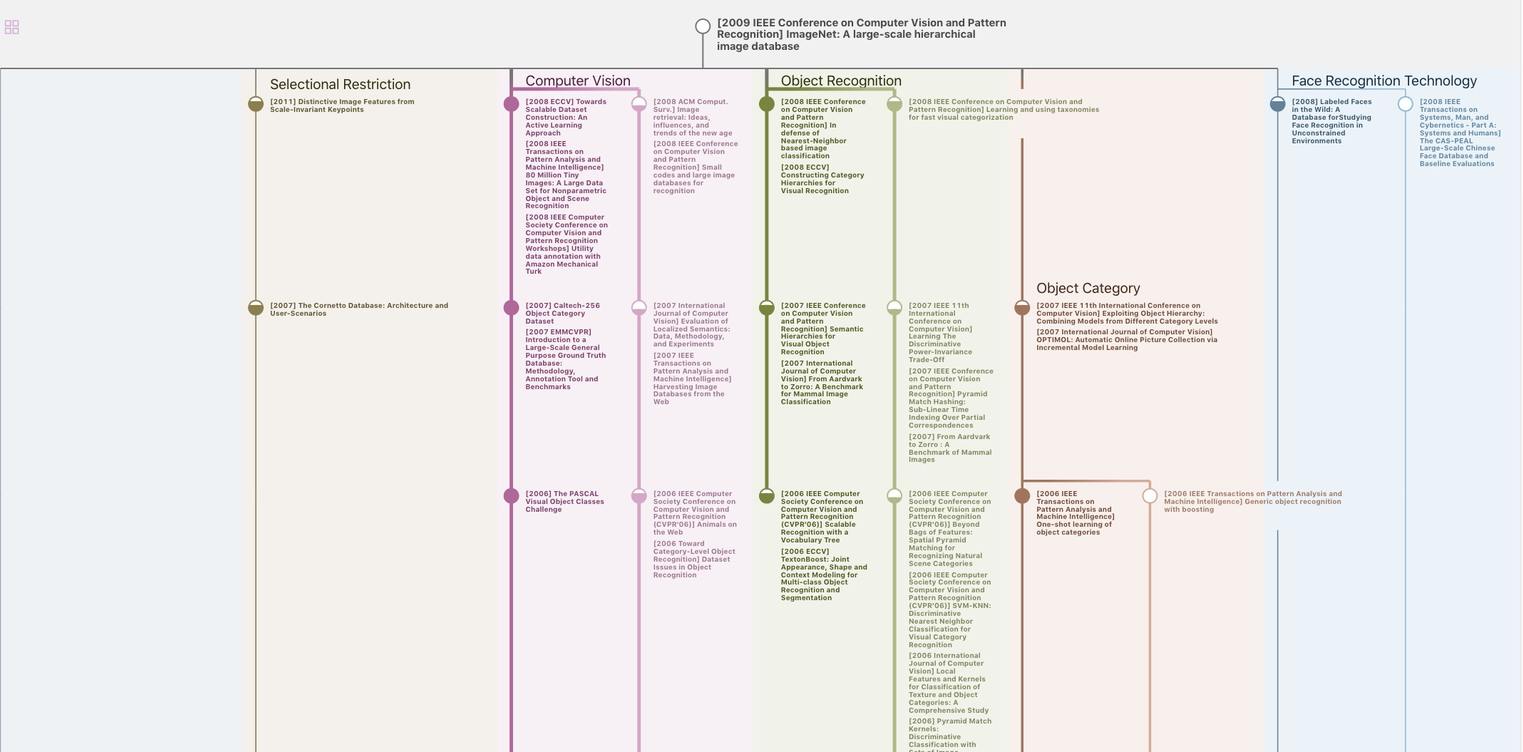
生成溯源树,研究论文发展脉络
Chat Paper
正在生成论文摘要