Learning to explore by reinforcement over high-level options
Machine Vision and Applications(2024)
摘要
Autonomous 3D environment exploration is a fundamental task for various applications such as navigation and object searching. The goal of exploration is to investigate a new environment and build a map efficiently. In this paper, we propose a new method which grants an agent two intertwined options of behaviors: “look-around” and “frontier navigation.” This is implemented by an option-critic architecture and trained by reinforcement learning algorithms. In each time step, an agent produces an option and a corresponding action according to the policy. We also take advantage of macro-actions by incorporating classic path-planning techniques to increase training efficiency. We demonstrate the effectiveness of the proposed method on two publicly available 3D environment datasets, and the results show our method achieves higher coverage than competing techniques with better efficiency. We also show that our method can be transferred and applied on a rover robot in real-world environments.
更多查看译文
关键词
Exploration,Option-critic,Reinforcement
AI 理解论文
溯源树
样例
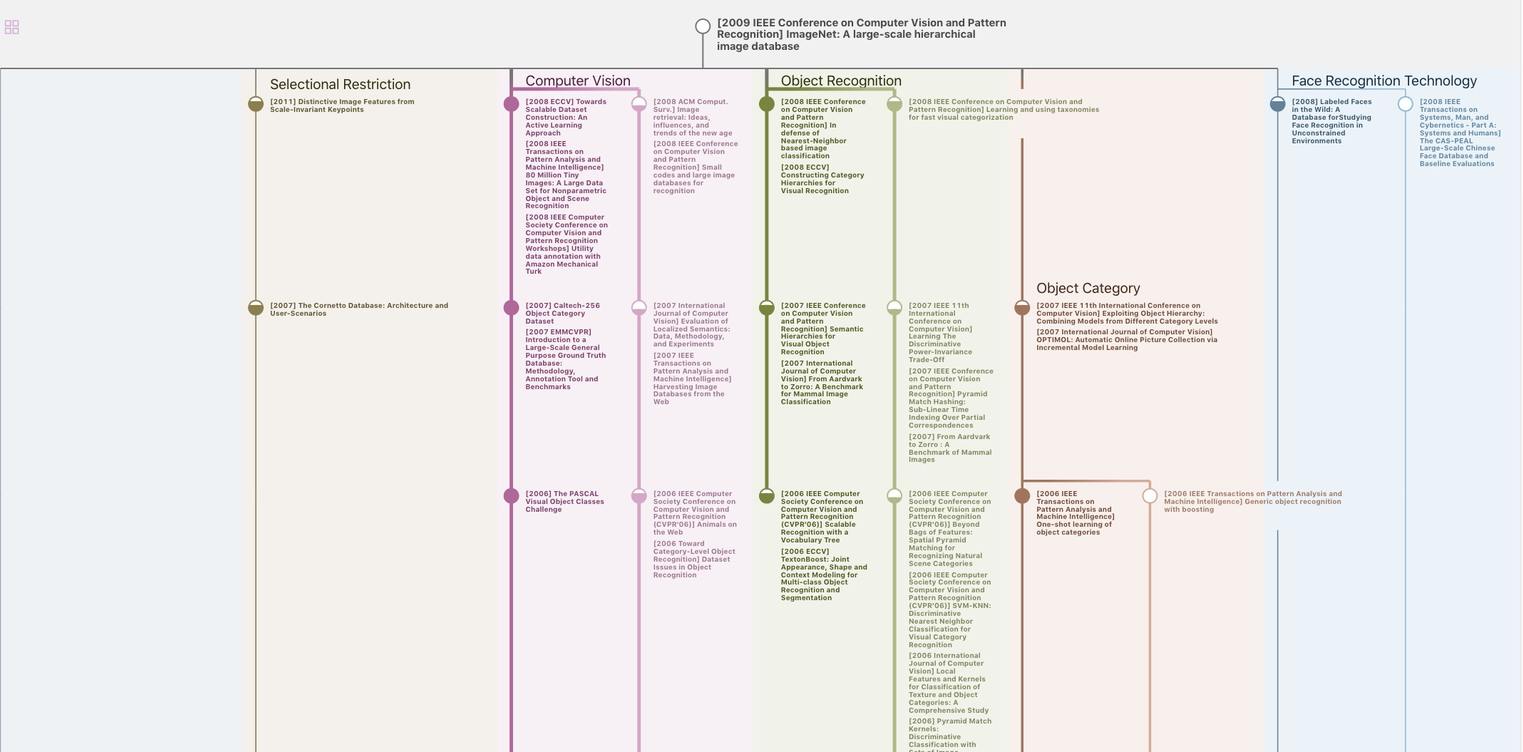
生成溯源树,研究论文发展脉络
Chat Paper
正在生成论文摘要