Learning to Assimilate in Chaotic Dynamical Systems.
Annual Conference on Neural Information Processing Systems(2021)
摘要
The accuracy of simulation-based forecasting in chaotic systems is heavily dependent on high-quality estimates of the system state at the beginning of the forecast. Data assimilation methods are used to infer these initial conditions by systematically combining noisy, incomplete observations and numerical models of system dynamics to produce highly effective estimation schemes. We introduce a self-supervised framework, which we call \textit{amortized assimilation}, for learning to assimilate in dynamical systems. Amortized assimilation combines deep learning-based denoising with differentiable simulation, using independent neural networks to assimilate specific observation types while connecting the gradient flow between these sub-tasks with differentiable simulation and shared recurrent memory. This hybrid architecture admits a self-supervised training objective which is minimized by an unbiased estimator of the true system state even in the presence of only noisy training data. Numerical experiments across several chaotic benchmark systems highlight the improved effectiveness of our approach compared to widely-used data assimilation methods.
更多查看译文
关键词
chaotic dynamical systems,learning,assimilate
AI 理解论文
溯源树
样例
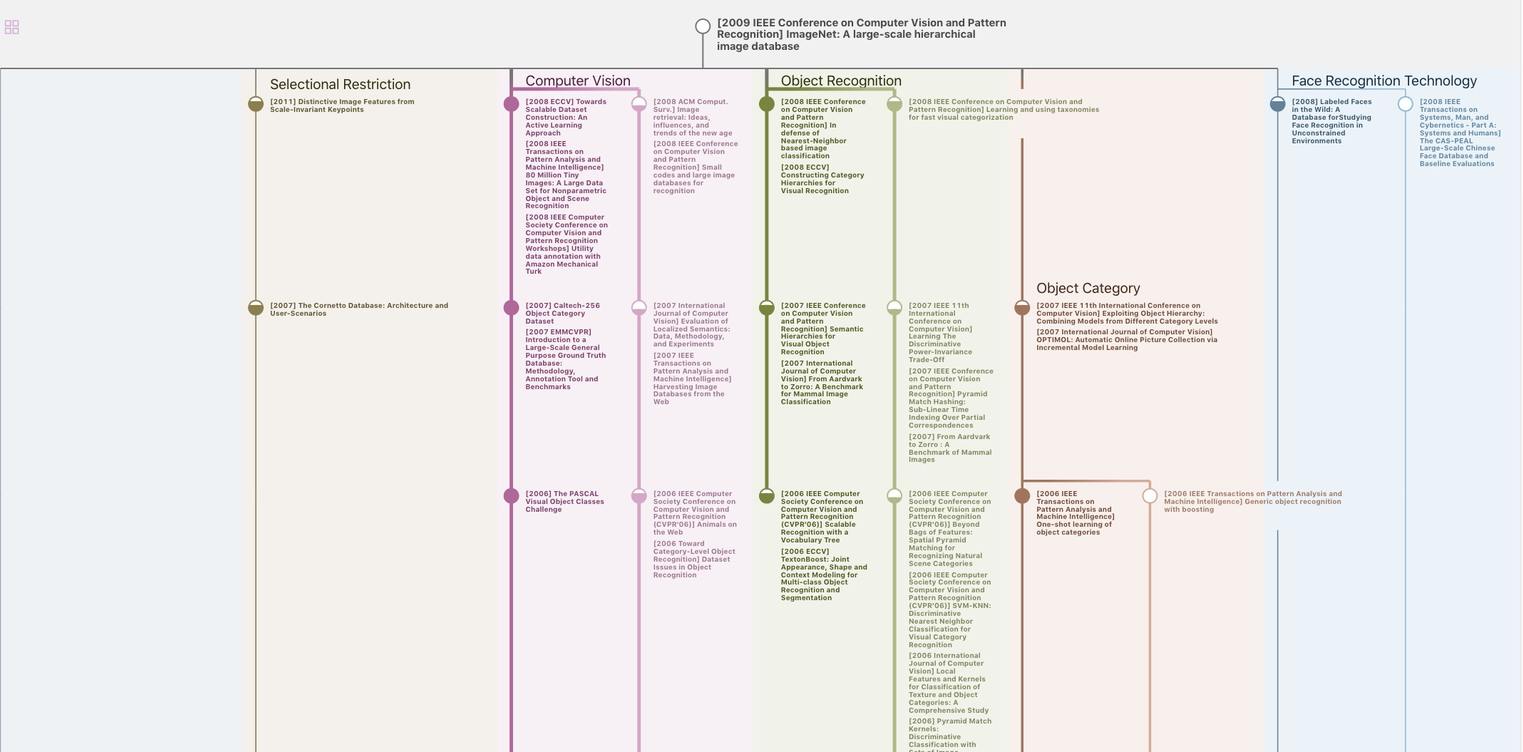
生成溯源树,研究论文发展脉络
Chat Paper
正在生成论文摘要