Nested Multiple Instance Learning with Attention Mechanisms
arxiv(2021)
摘要
Multiple instance learning (MIL) is a type of weakly supervised learning where multiple instances of data with unknown labels are sorted into bags. Since knowledge about the individual instances is incomplete, labels are assigned to the bags containing the instances. While this method fits diverse applications were labelled data is scarce, it lacks depth for solving more complex scenarios where associations between sets of instances have to be made, like finding relevant regions of interest in an image or detecting events in a set of time-series signals. Nested MIL considers labelled bags within bags, where only the outermost bag is labelled and inner-bags and instances are represented as latent labels. In addition, we propose using an attention mechanism to add interpretability, providing awareness into the impact of each instance to the weak bag label. Experiments in classical image datasets show that our proposed model provides high accuracy performance as well as spotting relevant instances on image regions.
更多查看译文
关键词
Weakly supervised learning,multiple instance learning,attention mechanism,interpretability
AI 理解论文
溯源树
样例
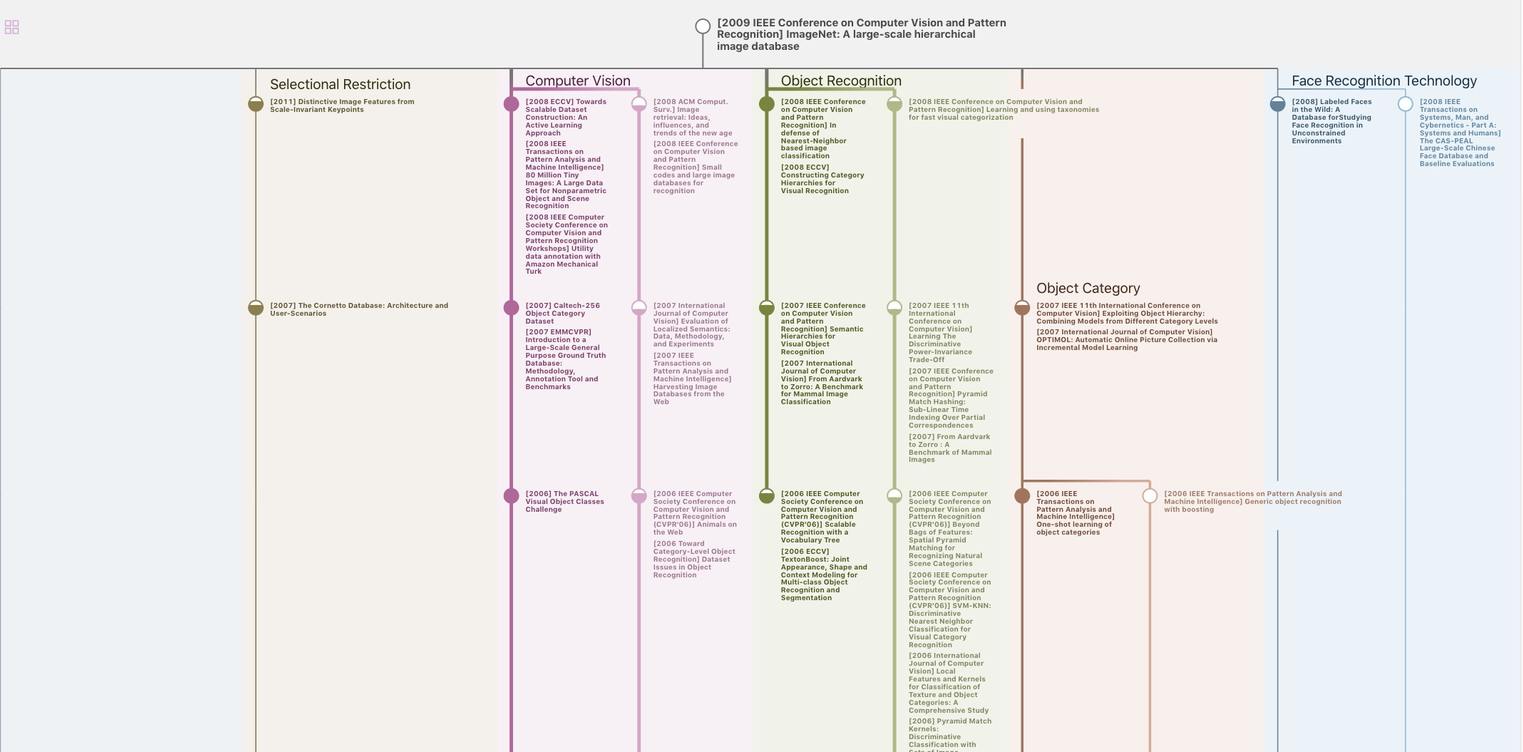
生成溯源树,研究论文发展脉络
Chat Paper
正在生成论文摘要