Exploring Non-Autoregressive End-to-End Neural Modeling for English Mispronunciation Detection and Diagnosis.
IEEE International Conference on Acoustics, Speech, and Signal Processing (ICASSP)(2022)
摘要
End-to-end (E2E) neural modeling has emerged as one predominant school of thought to develop computer-assisted language training (CAPT) systems, showing competitive performance to conventional pronunciation-scoring based methods. However, current E2E neural methods for CAPT are faced with at least two pivotal challenges. On one hand, most of the E2E methods operate in an autoregressive manner with left-to-right beam search to dictate the pronunciations of an L2 learners. This however leads to very slow inference speed, which inevitably hinders their practical use. On the other hand, E2E neural methods are normally data greedy and meanwhile an insufficient amount of nonnative training data would often reduce their efficacy on mispronunciation detection and diagnosis (MD&D). In response, we put forward a novel MD&D method that leverages non-autoregressive (NAR) E2E neural modeling to dramatically speed up the inference time while maintaining performance in line with the conventional E2E neural methods. In addition, we design and develop a pronunciation modeling network stacked on top of the NAR E2E models of our method to further boost the effectiveness of MD&D. Empirical experiments conducted on the L2-ARCTIC English dataset seems to validate the feasibility of our method, in comparison to some top-of-the-line E2E models and an iconic pronunciation-scoring based method built on a DNN-HMM acoustic model.
更多查看译文
关键词
Computer-assisted language training,mispronunciation detection and diagnosis,non-autoregressive,pronunciation modeling
AI 理解论文
溯源树
样例
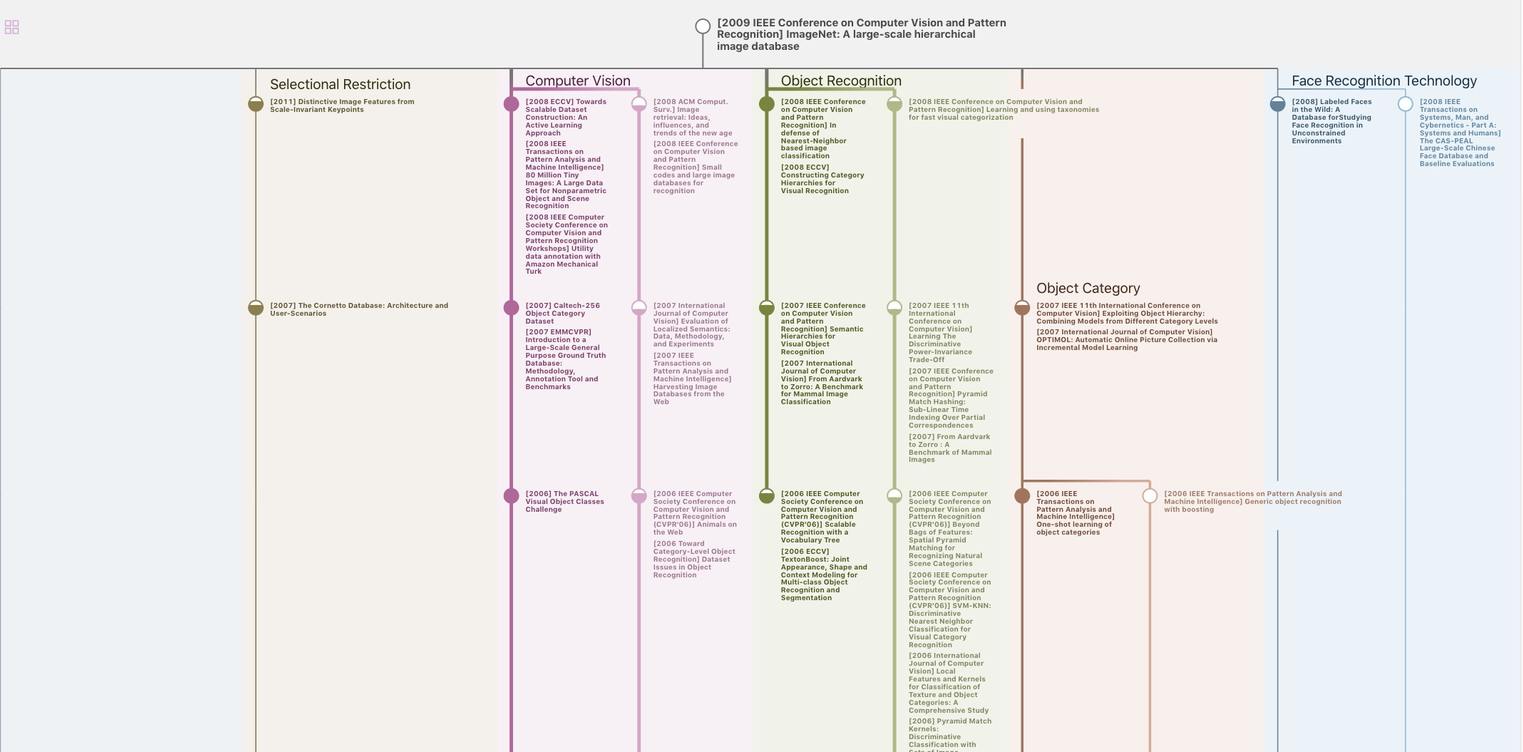
生成溯源树,研究论文发展脉络
Chat Paper
正在生成论文摘要