A Framework for Transforming Specifications in Reinforcement Learning
arxiv(2021)
摘要
Reactive synthesis algorithms allow automatic construction of policies to control an environment modeled as a Markov Decision Process (MDP) that are optimal with respect to high-level temporal logic specifications assuming the MDP model is known a priori. Reinforcement learning algorithms, in contrast, are designed to learn an optimal policy when the transition probabilities of the MDP are unknown, but require the user to associate local rewards with transitions. The appeal of high-level temporal logic specifications has motivated research to develop RL algorithms for synthesis of policies from specifications. To understand the techniques, and nuanced variations in their theoretical guarantees, in the growing body of resulting literature, we develop a formal framework for defining transformations among RL tasks with different forms of objectives. We define the notion of sampling-based reduction to relate two MDPs whose transition probabilities can be learnt by sampling, followed by formalization of preservation of optimal policies, convergence, and robustness. We then use our framework to restate known results, establish new results to fill in some gaps, and identify open problems.
更多查看译文
关键词
transforming specifications,reinforcement learning
AI 理解论文
溯源树
样例
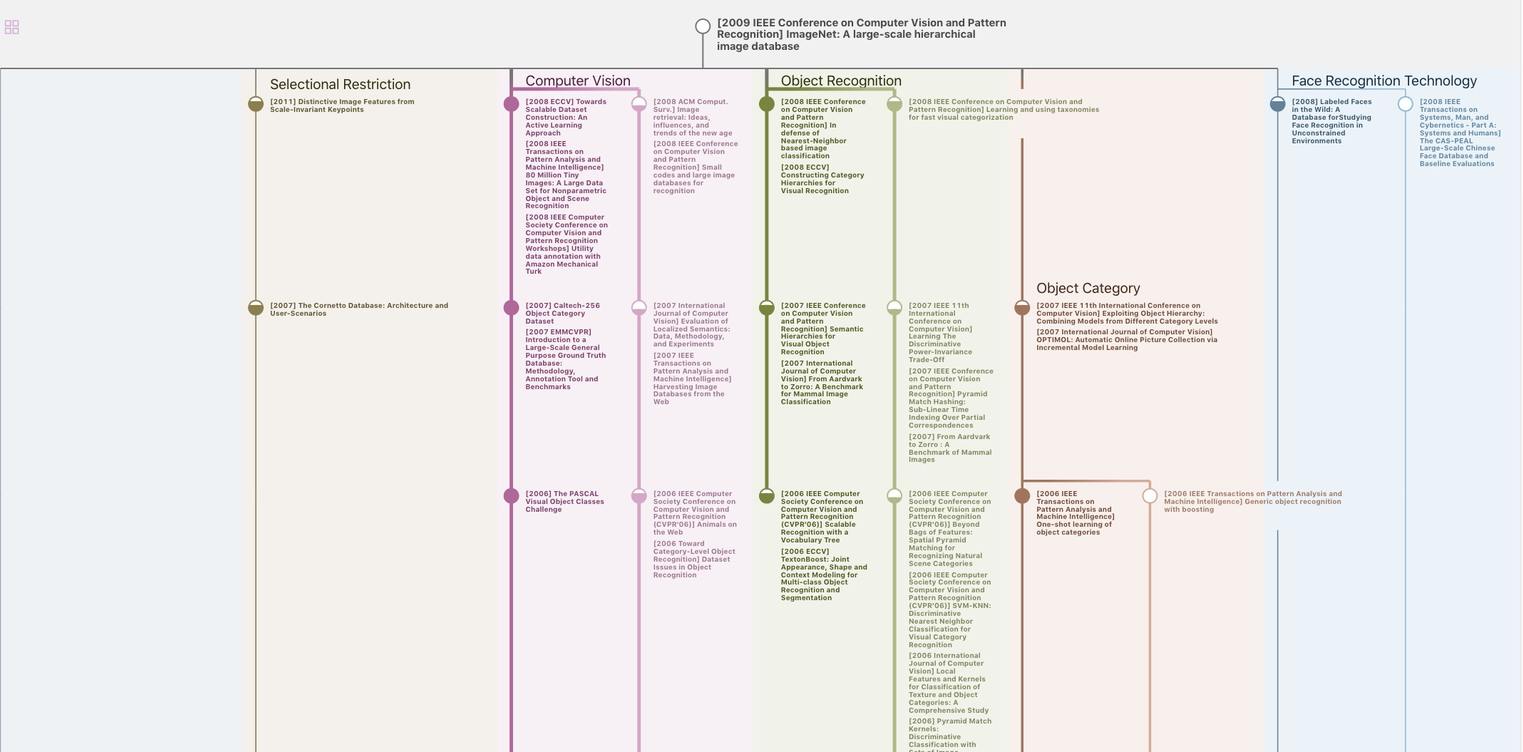
生成溯源树,研究论文发展脉络
Chat Paper
正在生成论文摘要