Distributing Deep Learning Hyperparameter Tuning for 3D Medical Image Segmentation
2022 IEEE 36TH INTERNATIONAL PARALLEL AND DISTRIBUTED PROCESSING SYMPOSIUM WORKSHOPS (IPDPSW 2022)(2022)
摘要
Most research on novel techniques for 3D Medical Image Segmentation (MIS) is currently done using Deep Learning with GPU accelerators. The principal challenge of such technique is that a single input can easily cope computing resources, and require prohibitive amounts of time to be processed. Distribution of deep learning and scalability over computing devices is an actual need for progressing on such research field. Conventional distribution of neural networks consist in “data parallelism”, where data is scattered over resources (e.g., GPUs) to parallelize the training of the model. However, “experiment parallelism” is also an option, where different training processes (i.e., on a hyper-parameter search) are parallelized across resources. While the first option is much more common on 3D image segmentation, the second provides a pipeline design with less dependence among parallelized processes, allowing overhead reduction and more potential scalability. In this work we present a design for distributed deep learning training pipelines, focusing on multi-node and multi-GPU environments, where the two different distribution approaches are deployed and benchmarked. We take as proof of concept the 3D U-Net architecture, using the MSD Brain Tumor Segmentation dataset, a state-of-art problem in medical image segmentation with high computing and space requirements. Using the BSC MareNostrum supercomputer as benchmarking environment, we use TensorFlow and Ray as neural network training and experiment distribution platforms. We evaluate the experiment speed-up when parallelizing, showing the potential for scaling out on GPUs and nodes. Also comparing the different parallelism techniques, showing how experiment distribution leverages better such resources through scaling, e.g. by a speed-up factor from x12 to x14 using 32 GPUs. Finally, we provide the implementation of the design open to the community, and the non-trivial steps and methodology for adapting and deploying a MIS case as the here presented.
更多查看译文
关键词
Distributed Deep Learning, Distributed Computing, GPU, Parallelism, Scalability
AI 理解论文
溯源树
样例
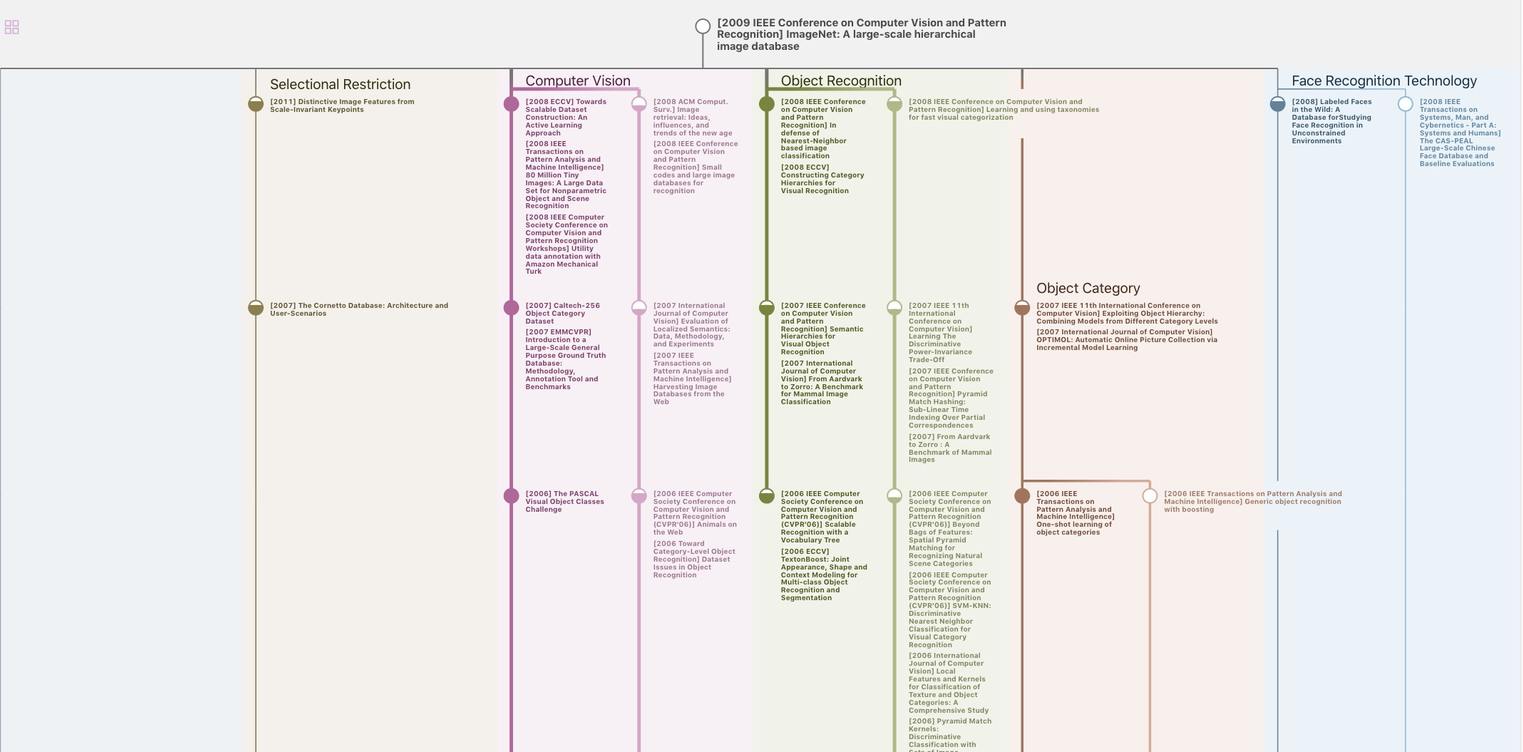
生成溯源树,研究论文发展脉络
Chat Paper
正在生成论文摘要