DFL: High-Performance Blockchain-Based Federated Learning
Distributed Ledger Technol Res Pract(2023)
摘要
Many researchers have proposed replacing the aggregation server in federated learning with a blockchain system to improve privacy, robustness, and scalability. In this approach, clients would upload their updated models to the blockchain ledger and use a smart contract to perform model averaging. However, the significant delay and limited computational capabilities of blockchain systems make it inefficient to support machine learning applications on the blockchain. In this paper, we propose a new public blockchain architecture called DFL, which is specially optimized for distributed federated machine learning. Our architecture inherits the merits of traditional blockchain systems while achieving low latency and low resource consumption by waiving global consensus. To evaluate the performance and robustness of our architecture, we implemented a prototype and tested it on a physical four-node network, and also developed a simulator to simulate larger networks and more complex situations. Our experiments show that the DFL architecture can reach over 90\% accuracy for non-I.I.D. datasets, even in the presence of model poisoning attacks, while ensuring that the blockchain part consumes less than 5\% of hardware resources.
更多查看译文
关键词
learning,high-performance,blockchain-based
AI 理解论文
溯源树
样例
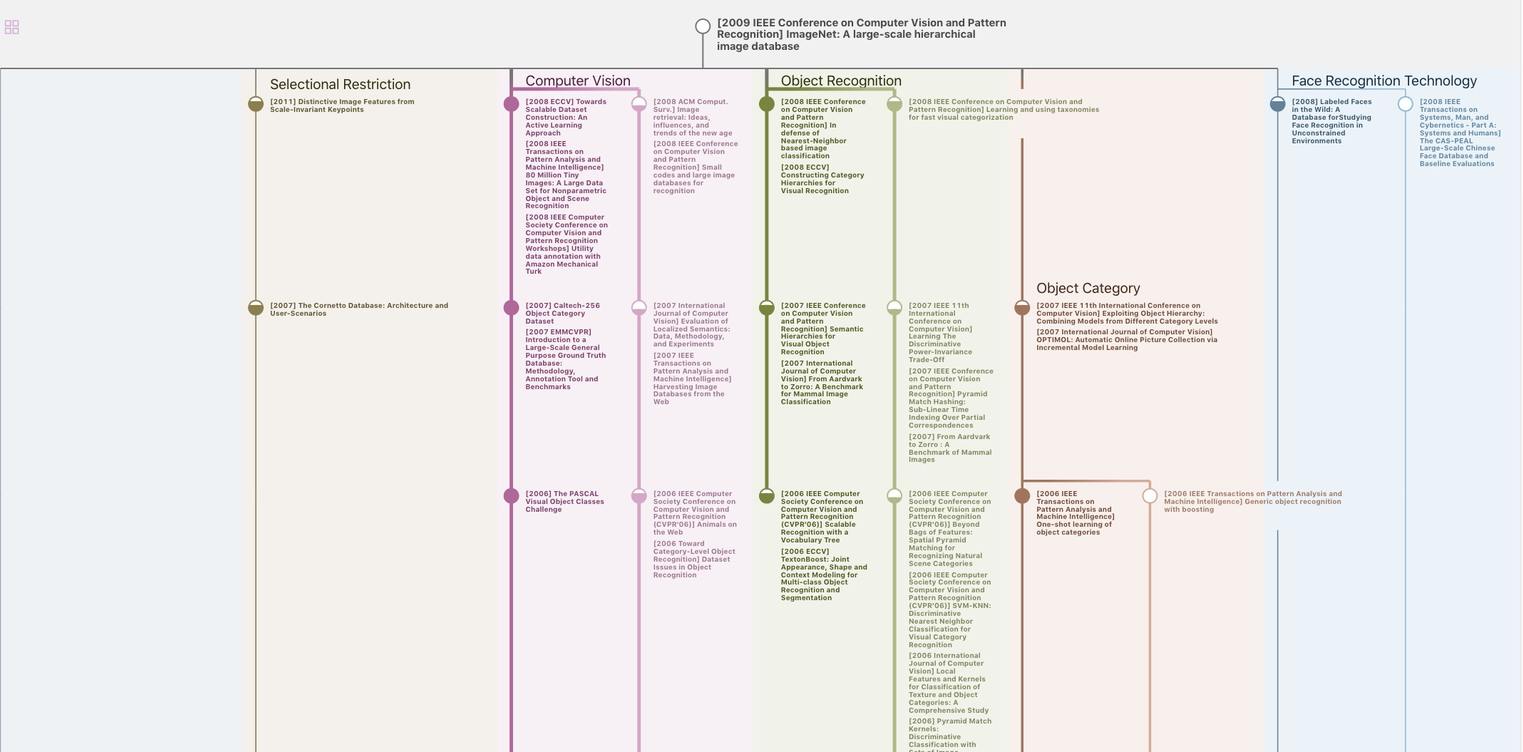
生成溯源树,研究论文发展脉络
Chat Paper
正在生成论文摘要