A CNN-LSTM-based fault classifier and locator for underground cables
NEURAL COMPUTING & APPLICATIONS(2021)
摘要
This paper presents a data-driven approach to classify and locate the faults occurring in underground distribution cables using a CNN-LSTM-based deep learning architecture. A sliding window method is adopted, using the current and voltage signal patches as inputs. The combination of various system parameters is considered to generalize the performance. Additionally, Gaussian noise is added to resemble and extend the approach to practical scenarios. The trained model is evaluated with the data from a new simulation, and the results confirm the generalizability of the adopted method. A comparison is made with other feasible approaches, which show that the adopted method is preferable to achieve better performance. Further, to ease the extension of the proposed method for different systems with similar configurations, parameters of the trained deep learning networks are exploited with the help of transfer learning.
更多查看译文
关键词
CNN-LSTM, Fault classification, Fault localization, Underground cables
AI 理解论文
溯源树
样例
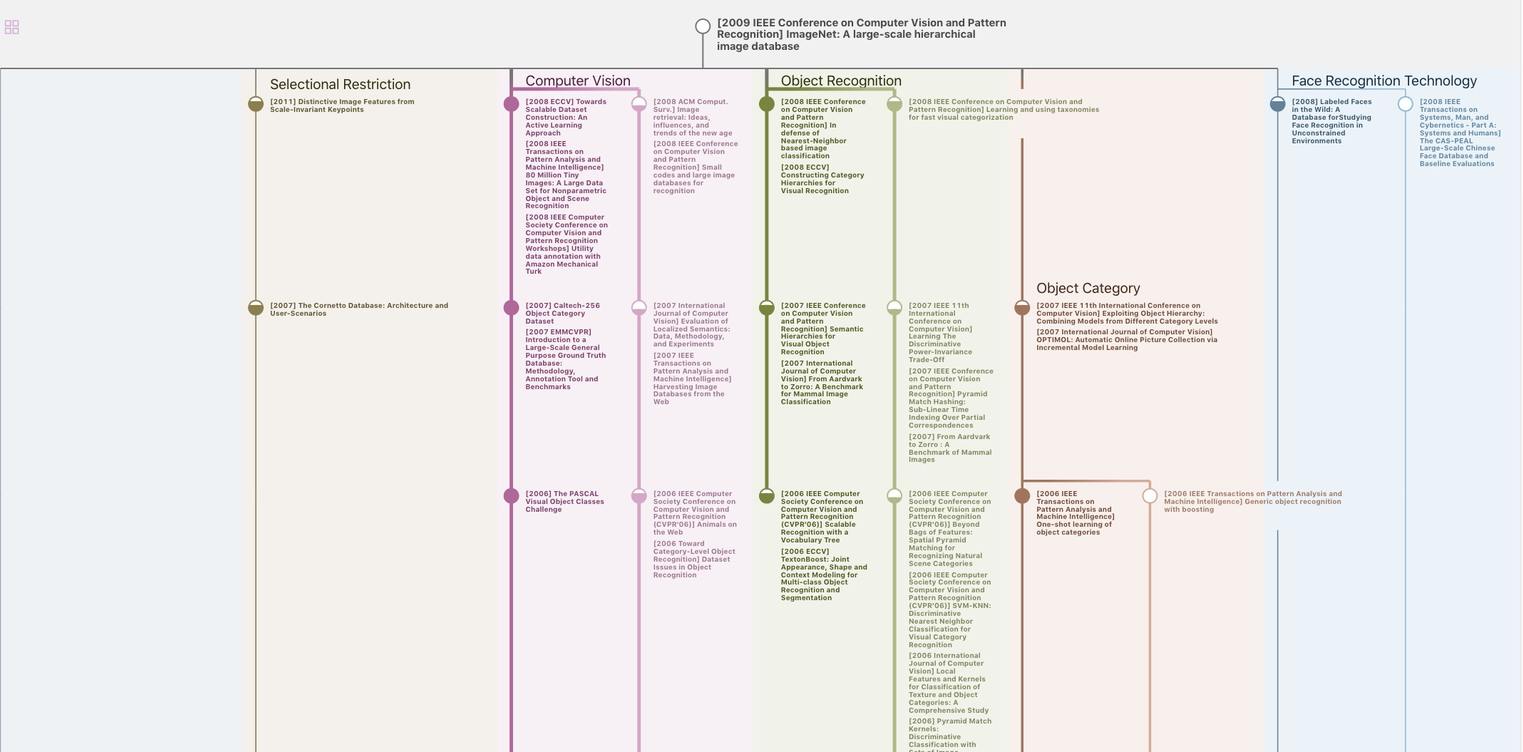
生成溯源树,研究论文发展脉络
Chat Paper
正在生成论文摘要