Extraction of a vascular function for a fully automated dynamic contrast-enhanced magnetic resonance brain image processing pipeline
MAGNETIC RESONANCE IN MEDICINE(2022)
摘要
Purpose To develop a deep-learning model that leverages the spatial and temporal information from dynamic contrast-enhanced magnetic resonance (DCE MR) brain imaging in order to automatically estimate a vascular function (VF) for quantitative pharmacokinetic (PK) modeling. Methods Patients with glioblastoma multiforme were scanned post-resection approximately every 2 months using a high spatial and temporal resolution DCE MR imaging sequence (approximate to 5 s and approximate to 2 cm(3)). A region over the transverse sinus was manually drawn in the dynamic T1-weighted images to provide a ground truth VF. The manual regions and their resulting VF curves were used to train a deep-learning model based on a 3D U-net architecture. The model concurrently utilized the spatial and temporal information in DCE MR images to predict the VF. In order to analyze the contribution of the spatial and temporal terms, different weighted combinations were examined. The manual and deep-learning predicted regions and VF curves were compared. Results Forty-three patients were enrolled in this study and 155 DCE MR scans were processed. The 3D U-net was trained using a loss function that combined the spatial and temporal information with different weightings. The best VF curves were obtained when both spatial and temporal information were considered. The predicted VF curve was similar to the manual ground truth VF curves. Conclusion The use of spatial and temporal information improved VF curve prediction relative to when only the spatial information is used. The method generalized well for unseen data and can be used to automatically estimate a VF curve suitable for quantitative PK modeling. This method allows for a more efficient clinical pipeline and may improve automation of permeability mapping.
更多查看译文
关键词
deep-learning, dynamic contrast enhanced (DCE) imaging, glioblastoma multiforme (GBM), permeability maps, vascular function (VF)
AI 理解论文
溯源树
样例
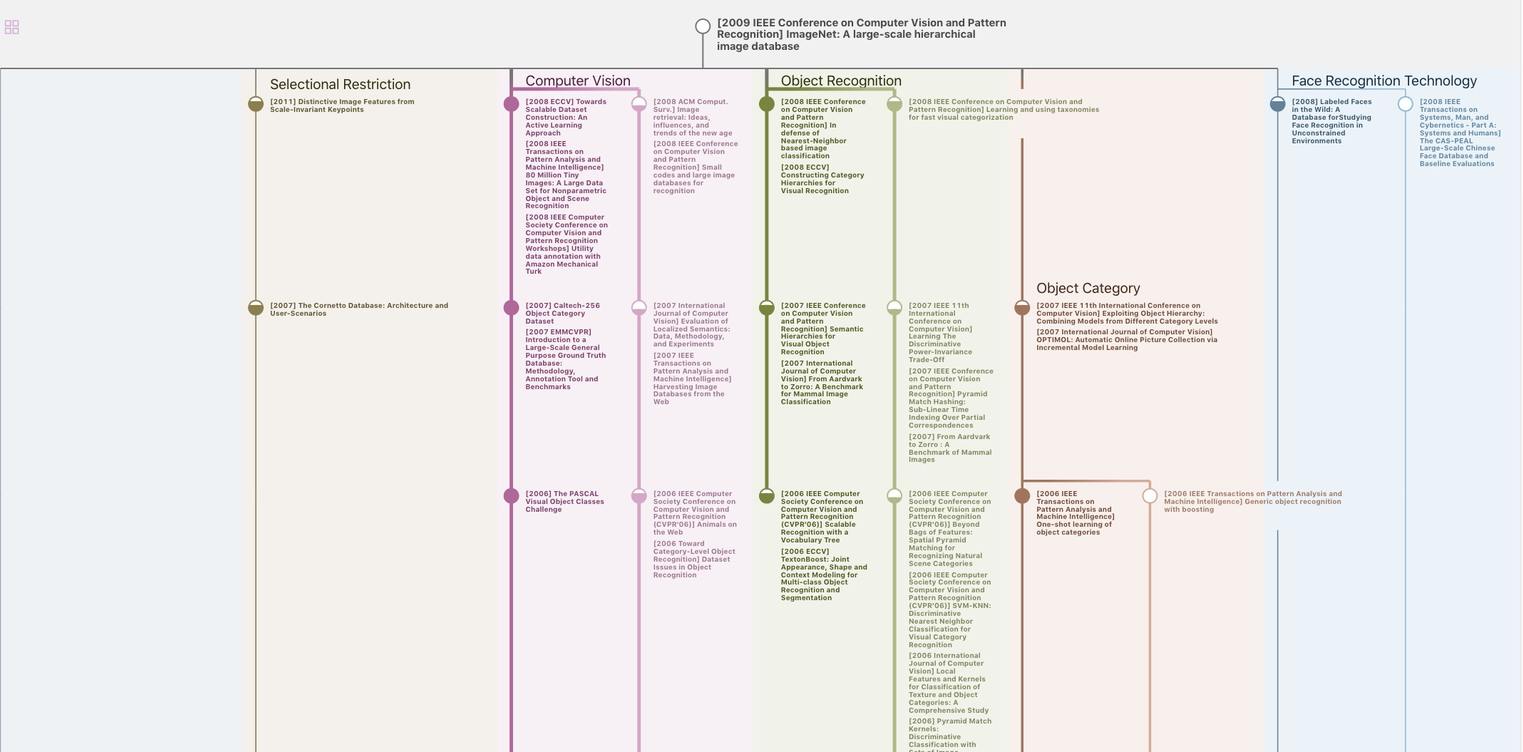
生成溯源树,研究论文发展脉络
Chat Paper
正在生成论文摘要