Incremental Adaptive Dynamic Programming for Approximate Optimal Tracking Control: a Decoupled and Model-Free Approach
arxiv(2021)
摘要
This paper proposes a new formulation for the optimal tracking control problem (OTCP) of Euler-Lagrange systems. This formulation extends the incremental adaptive dynamic programming (IADP) technique, a reinforcement learning based method for solving the robust optimal regulation control problem (RORCP), to learn the approximate solution to the OTCP. Departing from available solutions to the OTCP, our developed tracking control scheme settles the curse of complexity problem in value function approximation from a decoupled way, circumvents the learning inefficiency regarding varying desired trajectories by avoiding introducing a reference trajectory dynamics into the learning process, and requires neither an accurate nor identified dynamics through the time delay estimation technique. Specifically, we first convert the intractable OTCP of a high-dimensional uncertain system into multiple manageable sub-RORCPs of low-dimensional incremental error subsystems. Then, the resulting sub-RORCPs are approximately solved by IADP implemented as a parallel critic learning structure. The proposed tracking control scheme is developed with rigorous theoretical analysis of system stability and weight convergence, and validated numerically on a 6-DoF quadrotor and experimentally on a 3-DoF robot manipulator.
更多查看译文
AI 理解论文
溯源树
样例
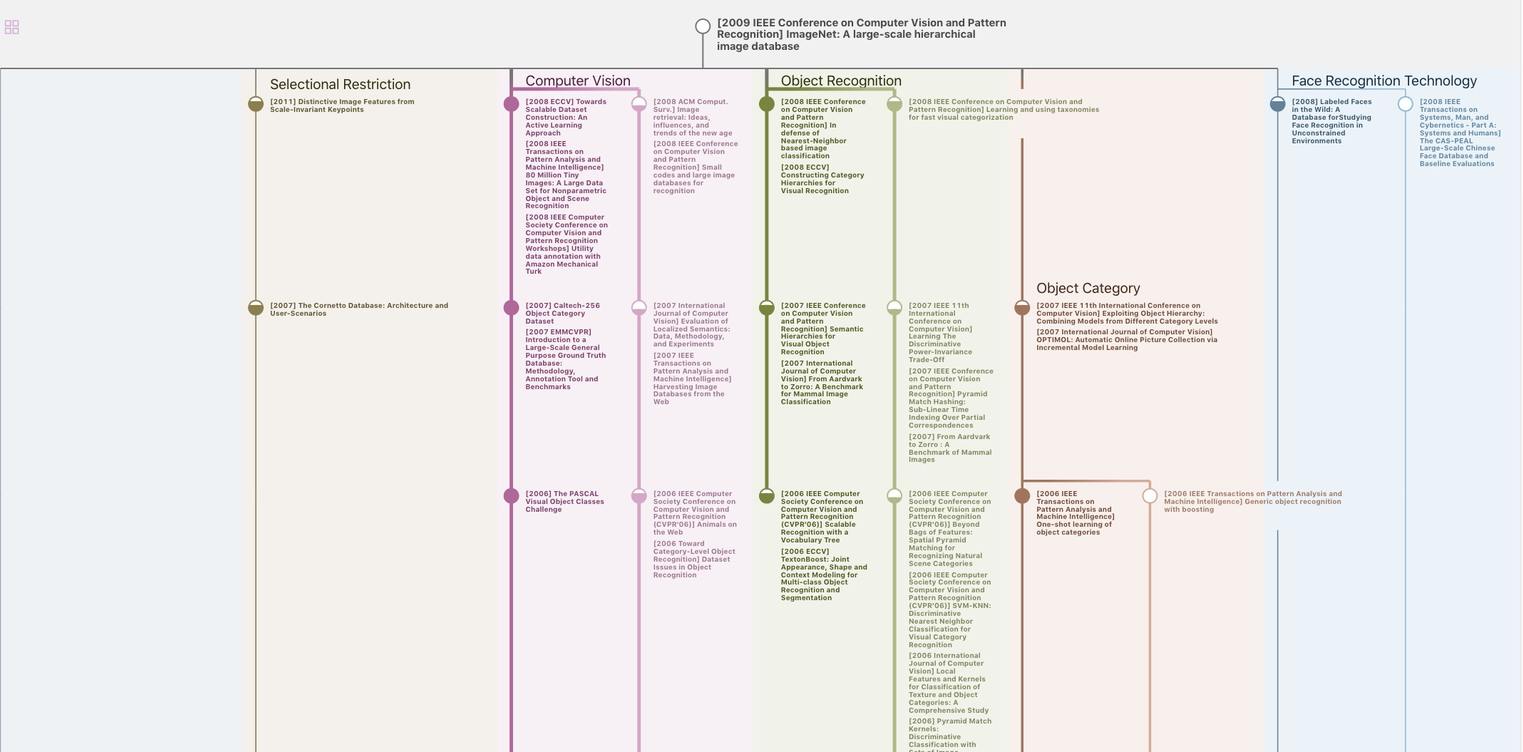
生成溯源树,研究论文发展脉络
Chat Paper
正在生成论文摘要