Continuous Speech Separation with Recurrent Selective Attention Network.
IEEE International Conference on Acoustics, Speech, and Signal Processing (ICASSP)(2022)
摘要
While permutation invariant training (PIT) based continuous speech separation (CSS) significantly improves the conversation transcription accuracy, it often suffers from speech leakages and failures in separation at "hot spot" regions because it has a fixed number of output channels. In this paper, we propose to apply recurrent selective attention network (RSAN) to CSS, which generates a variable number of output channels based on active speaker counting. In addition, we propose a novel block-wise dependency extension of RSAN by introducing dependencies between adjacent processing blocks in the CSS framework. It enables the network to utilize the separation results from the previous blocks to facilitate the current block processing. Experimental results on the LibriCSS dataset show that the RSAN-based CSS (RSAN-CSS) network consistently improves the speech recognition accuracy over PIT-based models. The proposed block-wise dependency modeling further boosts the performance of RSAN-CSS.
更多查看译文
关键词
Continuous speech separation,recurrent selective attention network,meeting transcription
AI 理解论文
溯源树
样例
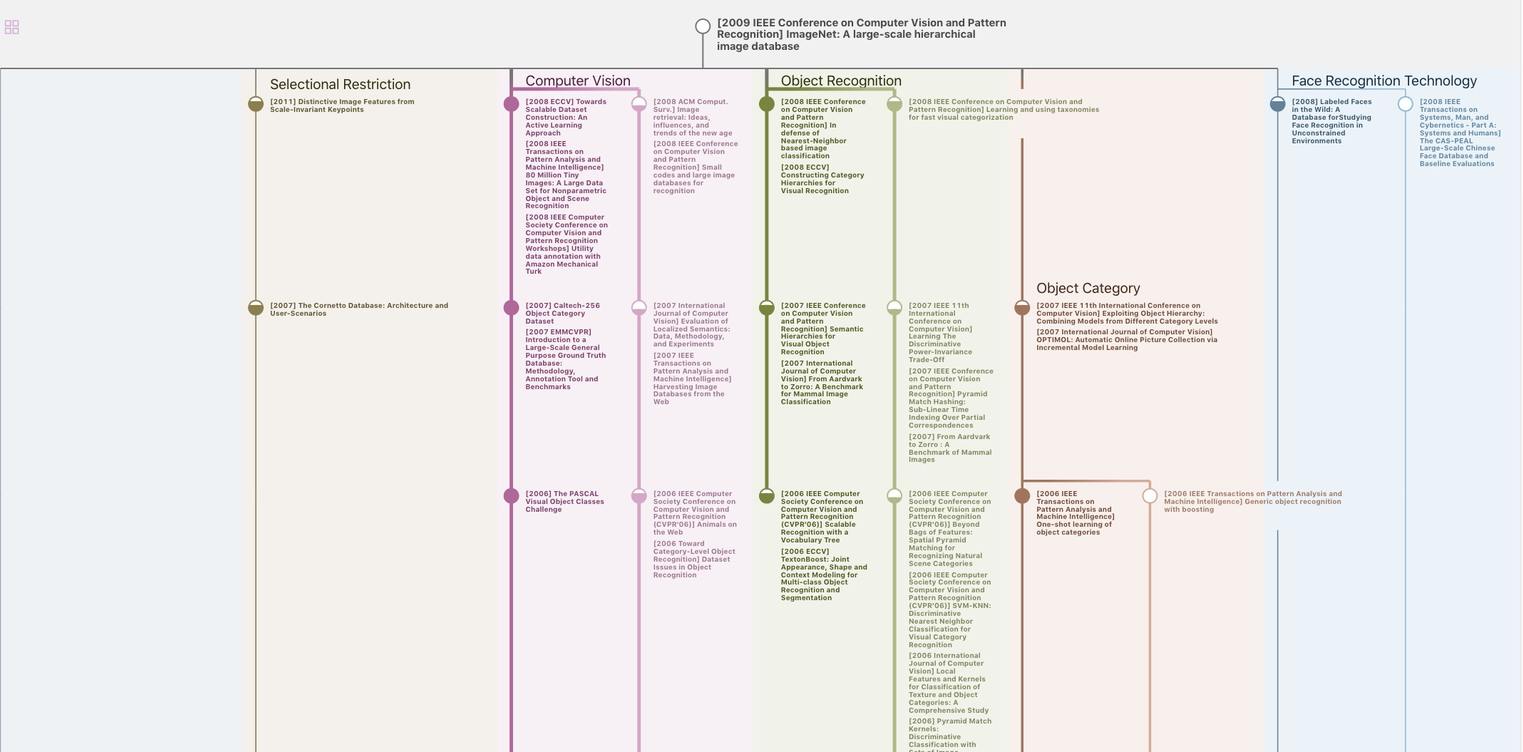
生成溯源树,研究论文发展脉络
Chat Paper
正在生成论文摘要