Adaptive Prediction of Hip Joint Center from X-ray Images Using Generalized Regularized Extreme Learning Machine and Globalized Bounded Nelder-Mead Strategy.
PRICAI(2021)
Abstract
The prediction of hip joint center (HJC) is an important step in hip dysplasia screening. Existing state-of-the-art identification methods focus on the development of Mose circle, functional and predictive methods. Those approaches extract few factors and ignore the adaptive HJC prediction, and their applications are not universally applicable. This paper proposes an adaptive HJC prediction model from X-ray images. The proposed network is based on generalized regularized extreme learning machine (GRELM) with three improvements: a multivariable feature extraction module, obtaining comprehensive predictive factors; an attribute optimization module based on Pearson correlation method and entropy weights, guiding the network to focus on useful information at variables; And appending a globalized bounded Nelder-Mead (GBNM) strategy to the framework to automatically and efficiently determine optimal model parameters. By integrating the above improvements in series, the models’ performances are gradually enhanced. Experimental results demonstrate the effectiveness of our method. Our method can be easily connected in series with an automatic landmark detection module, and the HJC can be quickly determined based on these anatomical landmarks using the proposed model.
MoreTranslated text
Key words
hip joint center,prediction,x-ray,nelder-mead
AI Read Science
Must-Reading Tree
Example
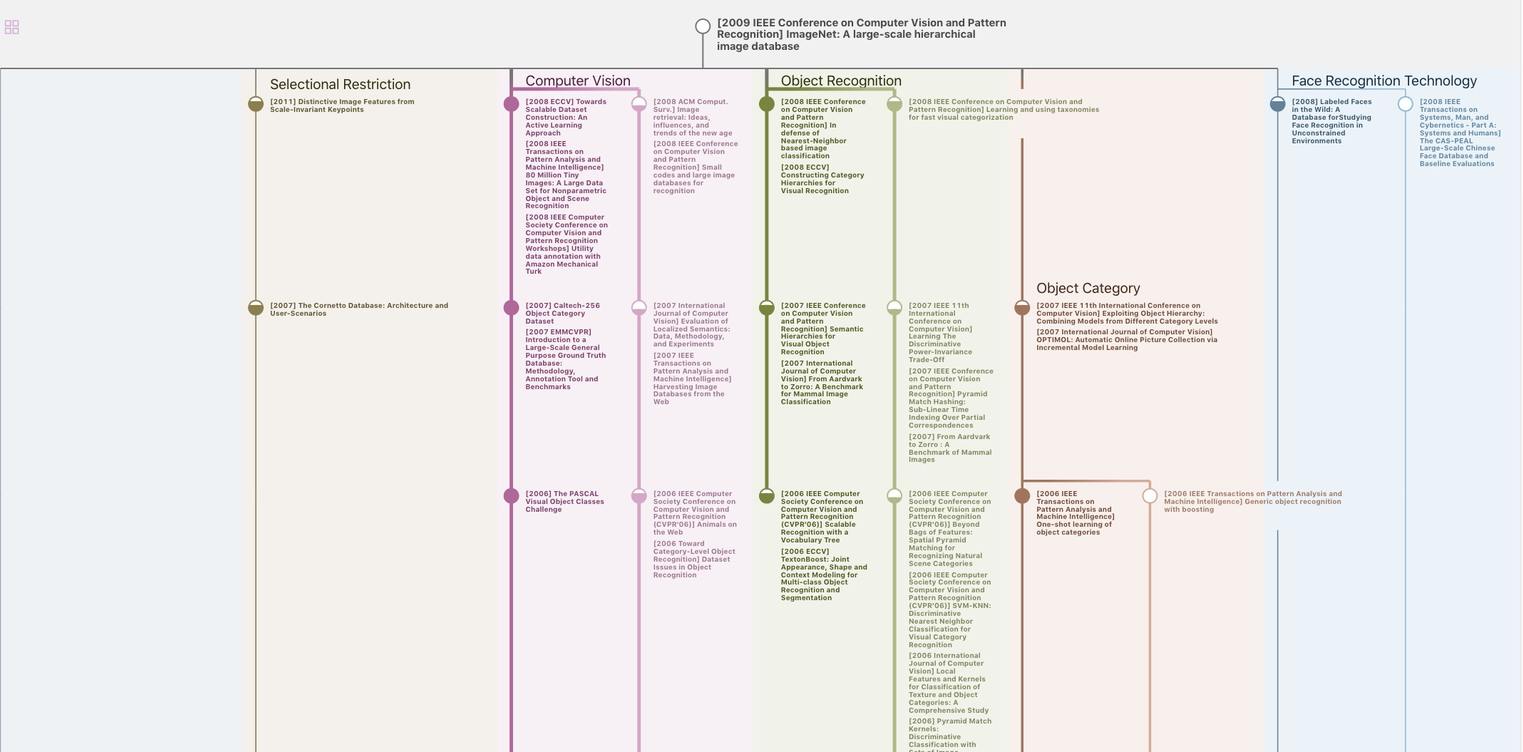
Generate MRT to find the research sequence of this paper
Chat Paper
Summary is being generated by the instructions you defined