Adaptive Anomaly Detection Network for Unseen Scene Without Fine-Tuning.
PRCV(2021)
摘要
Anomaly detection in video is a challenging task with great application value. Most existing approaches formulate anomaly detection as a reconstruction/prediction problem established on the encoderdecoder structure. However, they suffer from the poor generalization performance when the model is directly applied to an unseen scene. To solve this problem, in this paper, we propose an Adaptive Anomaly Detection Network (AADNet) to realize few-shot scene-adaptive anomaly detection. Our core idea is to learn an adaptive model, which can identify abnormal events without fine-tuning when transferred to a new scene. To this end, in AADNet, a Segments Similarity Measurement (SSM) module is utilized to calculate the cosine distance of different input video segments, based on which the normal segments will be gathered. Meanwhile, to further exploit the information of normal events, we design a novel Relational Scene Awareness (RSA) module to capture the pixelto-pixel relationship between different segments. By combining the SSM module with RSA, the proposed AADNet becomes much more generative. Extensive experiments on four datasets demonstrate our method can adapt to a new scene effectively without fine-tuning and achieve the state-of-the-art performance.
更多查看译文
关键词
Anomaly detection,Scene-adaptive,Few-shot learning
AI 理解论文
溯源树
样例
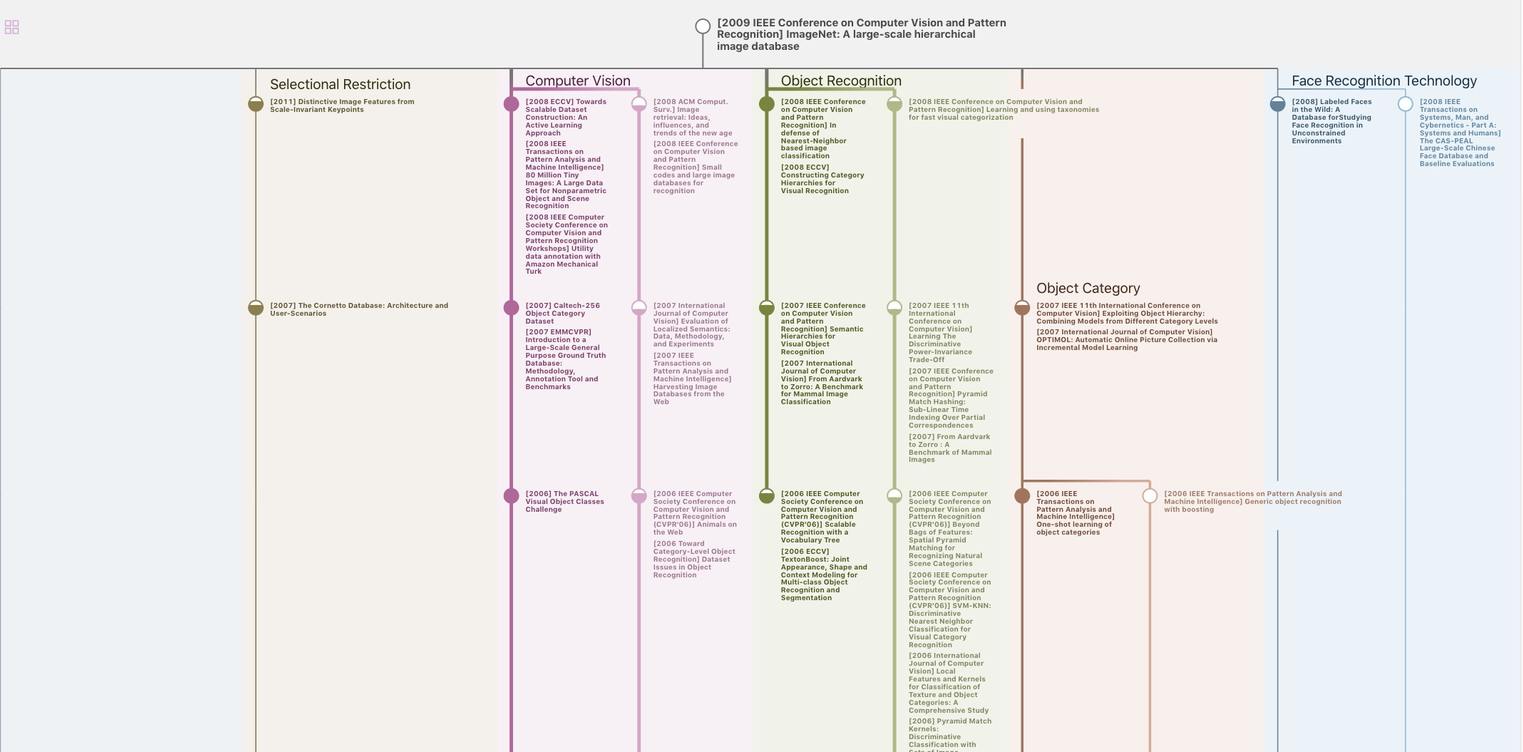
生成溯源树,研究论文发展脉络
Chat Paper
正在生成论文摘要