A multimodal approach for regional GDP prediction using social media activity and historical information
APPLIED SOFT COMPUTING(2021)
摘要
This work proposes a multimodal approach with which to predict the regional Gross Domestic Product (GDP) by combining historical GDP values with the embodied information in Twitter messages concerning the current economic condition. This proposal is of great interest, since it delivers forecasts at higher frequencies than both the official statistics (published only annually at the regional level in Spain) and the existing unofficial quarterly predictions (which rely on economic indicators that are available only after months of delay). The proposed method is based on a two-stage architecture. In the first stage, a multi-task autoencoder is initially used to obtain a GDP-related representation of tweets, which are then filtered to remove outliers and to obtain the GDP prediction from the consensus of opinions. In a second stage, this result is combined with the historical GDP values of the region using a multimodal network. The method is evaluated in four different regions of Spain using the tweets written by the most relevant economists, politicians, newspapers and institutions in each one. The results show that our approach successfully learns the evolution of the GDP using only historical information and tweets, thus making it possible to provide earlier forecasts about the regional GDP. This method also makes it possible to establish which the most or least influential opinions regarding this prediction are. As an additional exercise, we have assessed how well our method predicted the effect of the COVID-19 pandemic. (C) 2021 Elsevier B.V. All rights reserved.
更多查看译文
关键词
Machine learning, Neural networks, Gross Domestic Product (GDP), Knowledge based economy
AI 理解论文
溯源树
样例
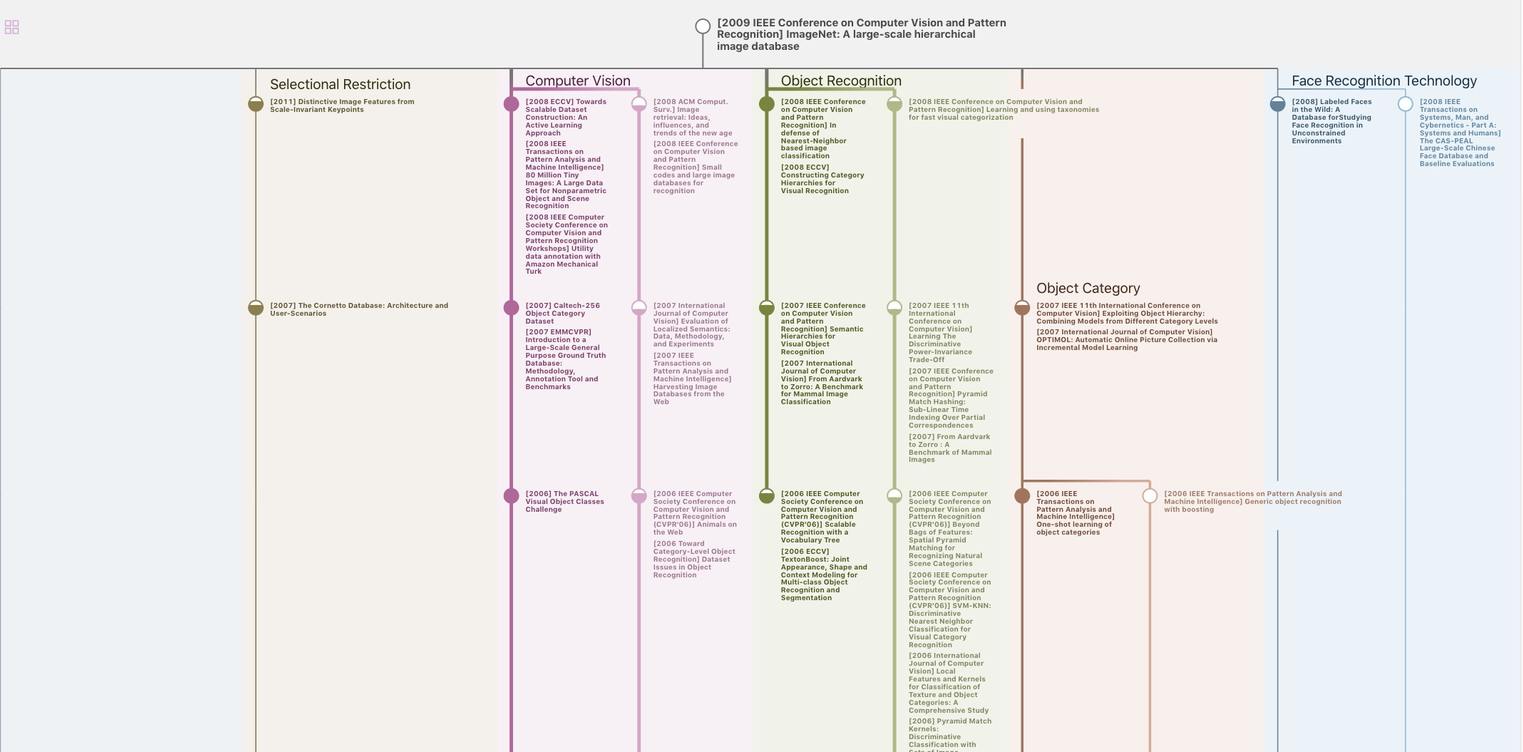
生成溯源树,研究论文发展脉络
Chat Paper
正在生成论文摘要