Predicting origin for bone metastatic cancer using deep learning-based pathology.
EBioMedicine(2023)
摘要
Metastasis is a common event in cancer progression. Rich in blood supply and red marrow, the skeletal system is one of the most common sites for malignant tumor metastasis.1Coleman R. Hadji P. Body J.J. et al.Bone health in cancer: ESMO clinical practice guidelines.Ann Oncol. 2020; 31: 1650-1663Summary Full Text Full Text PDF PubMed Scopus (162) Google Scholar Bone metastatic cancer is the most common malignant bone tumor. After bone metastases, a range of bone-related complications can occur, such as pathological fractures, spinal compression, bone pain, etc., which can greatly affect the living quality and prognosis of patients.2D'Oronzo S. Coleman R. Brown J. et al.Metastatic bone disease: pathogenesis and therapeutic options: up-date on bone metastasis management.J Bone Oncol. 2019; 15100205Crossref PubMed Scopus (149) Google Scholar Currently, the treatment of bone metastases is usually drug therapy, radionuclide therapy, local radiotherapy, and surgery. Treatment options for bone metastatic cancer include drug therapy, radionuclide therapy, local radiotherapy, and surgery. The specific treatment depends on the primary site. At present, primary tumor biomarkers, whole body imaging examination, biopsy immunohistochemistry test and so on are used to infer the origin for bone metastatic cancer (OBMC). However, these methods are usually time-consuming, laborious, lack of accuracy, and lack of comprehensive diagnostic rules. Therefore, it is urgent to develop a new method to predict OBMC, improve the efficiency and accuracy of diagnosis, and assist treatment decision-making. To solve the above problems, in a recent issue of eBioMedicine, the researchers proposed a deep learning-based OBMC prediction algorithm, which could extract key information of bone metastatic cancer from hematoxylin-eosin (HE) staining slides of biopsy tissues.3Zhu L. Shi H. Wei H. et al.An accurate prediction of the origin for bone metastatic cancer using deep learning on digital pathological images.eBioMedicine. 2022; https://doi.org/10.1016/j.ebiom.2022.104426Summary Full Text Full Text PDF Scopus (1) Google Scholar The input to their prediction model was the tumor region segmented by pathologists on HE slices. By modifying several state-of-the-art deep learning feature extractors, the researchers converted small patches in each region into structured feature vectors. They then fused the feature vectors using an attention mechanism to keep information flow highly representative and informative to the tumor region, and achieved region-level prediction. Finally, the patient-level prediction was obtained by averaging the results in each region. The authors conducted a comprehensive and in-depth validation of their proposed model. They not only used an external dataset to test the robustness of the model to different imaging devices, but also evaluated its effect on improving the diagnosis process in a clinical scene based on a group of patients with unknown primary tumor sites. Since the digital HE slices are readily available in routine clinical approaches, yielding a high prediction accuracy for OBMC, the proposed model is expected to provide significant auxiliary effects for the diagnosis of bone metastatic cancer. In recent years, artificial intelligence (AI) has made great progress in disease diagnosis,4Hunter B. Chen M. Ratnakumar P. et al.A radiomics-based decision support tool improves lung cancer diagnosis in combination with the Herder score in large lung nodules.eBioMedicine. 2022; 86104344Summary Full Text Full Text PDF PubMed Scopus (3) Google Scholar staging,5Dong D. Fang M.J. Tang L. et al.Deep learning radiomic nomogram can predict the number of lymph node metastasis in locally advanced gastric cancer: an international multicenter study.Ann Oncol. 2020; 31: 912-920Summary Full Text Full Text PDF PubMed Scopus (138) Google Scholar prognosis prediction,6Zhang W. Fang M. Dong D. et al.Development and validation of a CT-based radiomic nomogram for preoperative prediction of early recurrence in advanced gastric cancer.Radiother Oncol. 2020; 145: 13-20Summary Full Text Full Text PDF PubMed Scopus (64) Google Scholar efficacy evaluation,7Liu Y. Wang Y. Wang Y. et al.Early prediction of treatment response to neoadjuvant chemotherapy based on longitudinal ultrasound images of HER2-positive breast cancer patients by Siamese multi-task network: a multicentre, retrospective cohort study.eClinicalMedicine. 2022; 52101562Summary Full Text Full Text PDF Scopus (4) Google Scholar etc. It has the potential to reshape medicine and improve clinical outcomes by integrating AI deeply into critical clinical tasks and creating collaborative effects in the workflow of clinicians. The fusion of digital pathology images and AI has resulted in the novel pathology intelligence analysis methods. This technique is able to extract deep-level features of tumor pathology images and overcome the difficulties of quantitatively measuring cell morphology and intra-regional heterogeneity. It has developed into an auxiliary diagnostic method with remarkable accuracy, stability, and automation, which has broad clinical application prospects.8Kers J. Bülow R.D. Klinkhammer B.M. et al.Deep learning-based classification of kidney transplant pathology: a retrospective, multicentre, proof-of-concept study.Lancet Digit Health. 2022; 4: e18-e26Summary Full Text Full Text PDF PubMed Scopus (16) Google Scholar A large number of novel AI frameworks with outstanding performances provide a broad reference for the design of intelligent cores in medical image analysis. In this study, the researchers tested the feature extraction capabilities of SwinTransformer and ConvNeXt. Transformer is a deep learning architecture based on multi-head self-attention mechanism and forward full-connected network. It discards the traditional convolutional structure and is particularly suitable for processing sequence-to-sequence transformations.9Gong L. Wang M. Shu L. et al.Automatic captioning of early gastric cancer using magnification endoscopy with narrow-band imaging.Gastrointest Endosc. 2022; 96: 929-942.e6Summary Full Text Full Text PDF PubMed Scopus (3) Google Scholar Although transformer was originally developed for natural language processing, various improved models have been derived for image-related tasks. In particular, SwinTransformer has some of the advantages of ResNet through sliding window operation and gradually expanding receptive field, and achieves remarkable image analysis performance. Correspondingly, ConvNeXt maintains the simple and efficient structure of convolutional networks while fully borrowing the advanced design of transformer. It successfully improves the performance of convolutional networks. How to embed AI models into clinical processes is a critical issue in the application of pathology intelligence analysis. In this study, researchers explored the added value of the model in clinical diagnosis when multiple candidate primary tumor sites were provided. Experimental results showed that their model achieved a top-3 accuracy of about 95%, and thus could be considered capable of significantly reducing clinical workload and diagnostic difficulty. Multiple highly informative candidates may be more acceptable to clinicians than providing only the result with the highest probability. This study directly analyzed the characteristics of tumor cells at the microscopic level based on routine HE slices. It is worth noting that multi-modality images tend to be complementary.10Bhattacharya I. Seetharaman A. Kunder C. et al.Selective identification and localization of indolent and aggressive prostate cancers via CorrSigNIA: an MRI-pathology correlation and deep learning framework.Med Image Anal. 2022; 75102288Summary Full Text Full Text PDF PubMed Scopus (12) Google Scholar For example, X-ray computed tomography can display anatomic information of lesions with high resolution, radionuclide imaging can specifically quantify metabolic function, and magnetic resonance imaging can detect fat and metastasis in bone marrow with high water content. Designing feature fusion algorithms for multi-modality images to leverage multi-source heterogeneous information is a valuable research direction. All authors contributed to conceptualization, writing, reviewing, editing and have read and agreed to the published version of the manuscript. The authors declare no conflict of interest. This commentary was supported by National Natural Science Foundation of China (82022036, 91959130, 81971776, 62027901, 91959205, 81771924, 92059203), Beijing Natural Science Foundation (L182061, Z20J00105) and China Postdoctoral Science Foundation (2022M720357). The funders had no role in the writing or editing of the manuscript, the decision to submit the manuscript for publication, or any aspect pertinent to the manuscript. All authors accept responsibility to submit for publication. An accurate prediction of the origin for bone metastatic cancer using deep learning on digital pathological imagesOur model demonstrated good performance to predict the OBMC from routine histology and had great potential for assisting pathologists with determining the OBMC accurately. Full-Text PDF Open Access
更多查看译文
AI 理解论文
溯源树
样例
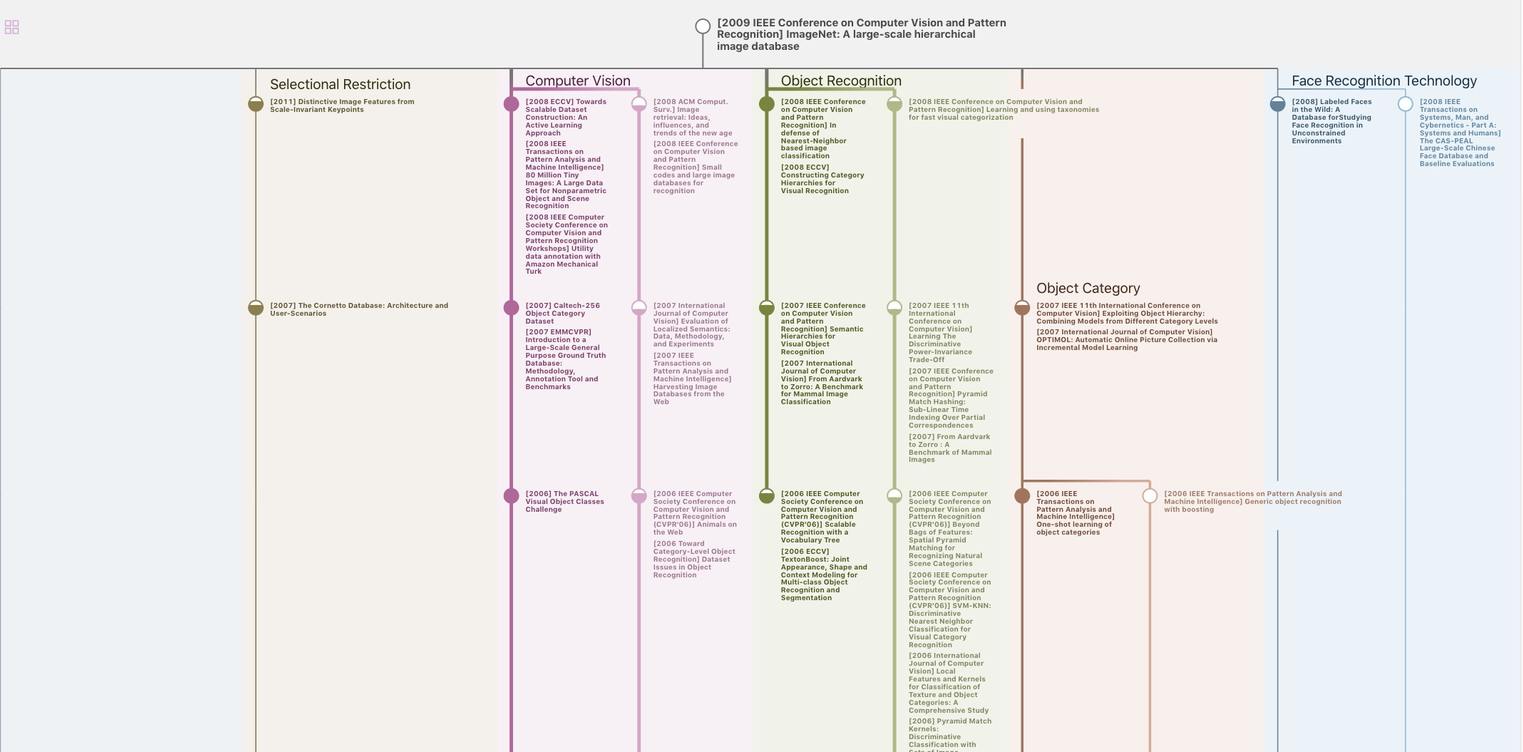
生成溯源树,研究论文发展脉络
Chat Paper
正在生成论文摘要