Transformers in computational visual media: A survey
Computational Visual Media(2022)
Abstract
Transformers, the dominant architecture for natural language processing, have also recently attracted much attention from computational visual media researchers due to their capacity for long-range representation and high performance. Transformers are sequence-to-sequence models, which use a self-attention mechanism rather than the RNN sequential structure. Thus, such models can be trained in parallel and can represent global information. This study comprehensively surveys recent visual transformer works. We categorize them according to task scenario: backbone design, high-level vision, low-level vision and generation, and multimodal learning. Their key ideas are also analyzed. Differing from previous surveys, we mainly focus on visual transformer methods in low-level vision and generation. The latest works on backbone design are also reviewed in detail. For ease of understanding, we precisely describe the main contributions of the latest works in the form of tables. As well as giving quantitative comparisons, we also present image results for low-level vision and generation tasks. Computational costs and source code links for various important works are also given in this survey to assist further development.
MoreTranslated text
Key words
visual transformer,computational visual media (CVM),high-level vision,low-level vision,image generation,multi-modal learning
AI Read Science
Must-Reading Tree
Example
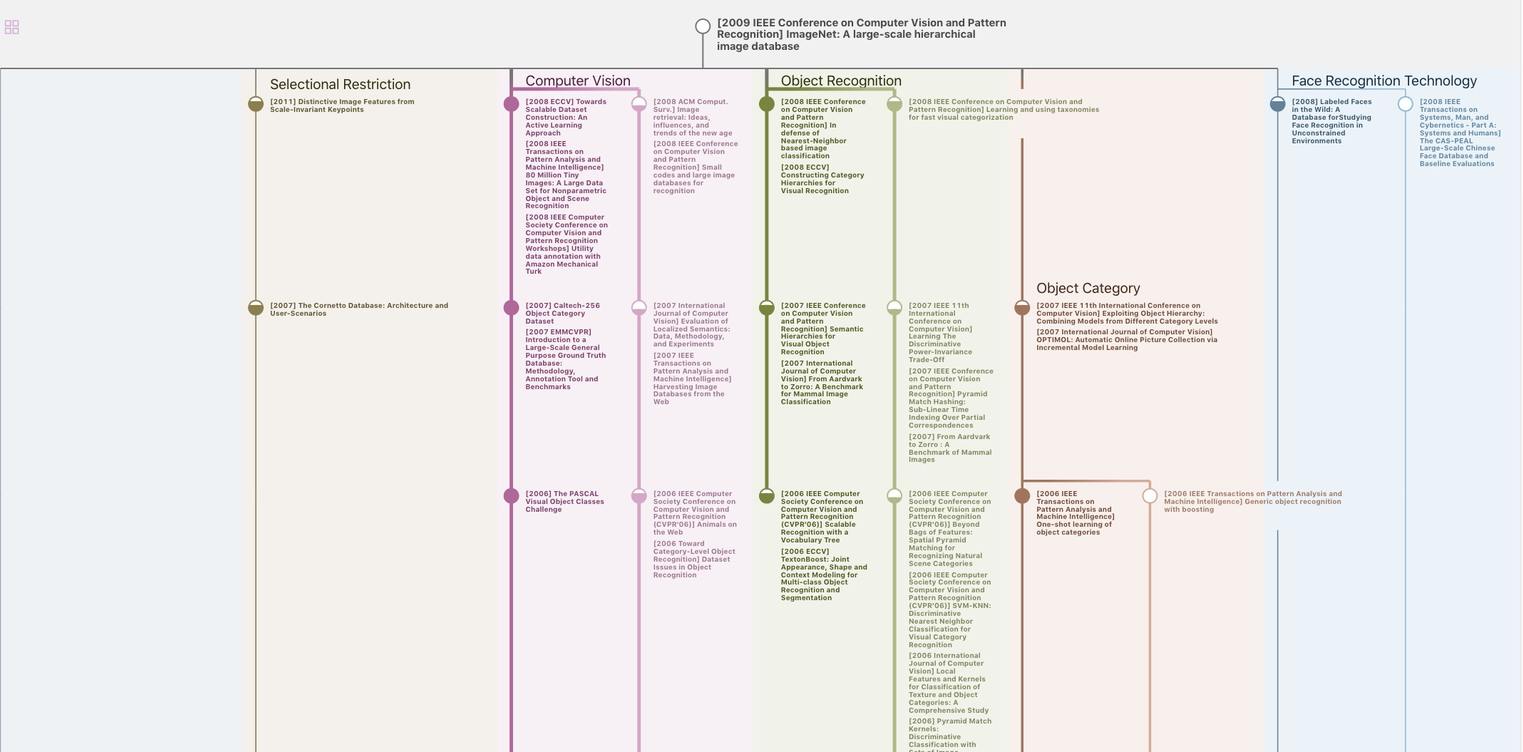
Generate MRT to find the research sequence of this paper
Chat Paper
Summary is being generated by the instructions you defined