GenURL: A General Framework for Unsupervised Representation Learning
IEEE TRANSACTIONS ON NEURAL NETWORKS AND LEARNING SYSTEMS(2024)
摘要
Unsupervised representation learning (URL) that learns compact embeddings of high-dimensional data without supervision has achieved remarkable progress recently. However, the development of URLs for different requirements is independent, which limits the generalization of the algorithms, especially prohibitive as the number of tasks grows. For example, dimension reduction (DR) methods, t-SNE and UMAP, optimize pairwise data relationships by preserving the global geometric structure, while self-supervised learning, SimCLR and BYOL, focuses on mining the local statistics of instances under specific augmentations. To address this dilemma, we summarize and propose a unified similarity-based URL framework, GenURL, which can adapt to various URL tasks smoothly. In this article, we regard URL tasks as different implicit constraints on the data geometric structure that help to seek optimal low-dimensional representations that boil down to data structural modeling (DSM) and low-dimensional transformation (LDT). Specifically, DSM provides a structure-based submodule to describe the global structures, and LDT learns compact low-dimensional embeddings with given pretext tasks. Moreover, an objective function, general Kullback-Leibler (GKL) divergence, is proposed to connect DSM and LDT naturally. Comprehensive experiments demonstrate that GenURL achieves consistent state-of-the-art performance in self-supervised visual learning, unsupervised knowledge distillation (KD), graph embeddings (GEs), and DR.
更多查看译文
关键词
Contrastive learning (CL),dimension reduction (DR),graph embedding (GE),knowledge distillation (KD),self-supervised learning
AI 理解论文
溯源树
样例
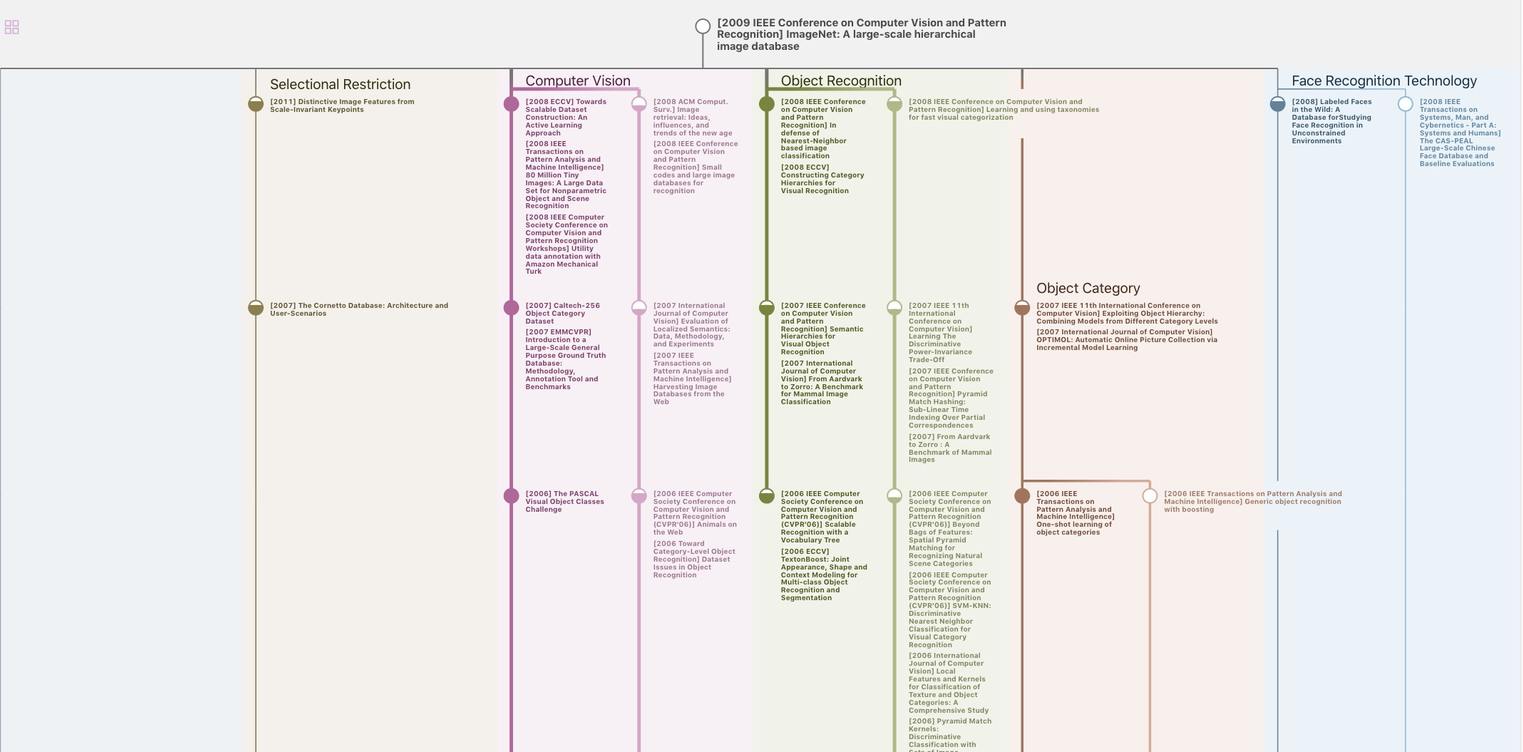
生成溯源树,研究论文发展脉络
Chat Paper
正在生成论文摘要