Model-based Reinforcement Learning with Provable Safety Guarantees via Control Barrier Functions
2021 IEEE INTERNATIONAL CONFERENCE ON ROBOTICS AND AUTOMATION (ICRA 2021)(2021)
摘要
Safety is a critical property in applications including robotics, transportation, and energy. Safety is especially challenging in reinforcement learning (RL) settings, in which uncertainty of the system dynamics may cause safety violations during exploration. Control Barrier Functions (CBFs), which enforce safety by constraining the control actions at each time step, are a promising approach for safety-critical control. This technique has been applied to ensure the safety of model-free RL, however, it has not been integrated into model-based RL. In this paper, we propose Uncertainty-Tolerant Control Barrier Functions (UTCBFs), a new class of CBFs to incorporate model uncertainty and provide provable safety guarantees with desired probability. Furthermore, we introduce an algorithm for model-based RL to guarantee safety by integrating CBFs with gradient-based policy search. Our approach is verified through a numerical study of a cart-pole system and an inverted pendulum system with comparison to state-of-the-art RL algorithms.
更多查看译文
关键词
model-based reinforcement learning,provable safety guarantees,critical property,reinforcement learning settings,safety violations,CBFs,control actions,safety-critical control,model-free RL,model-based RL,Uncertainty-Tolerant Control Barrier Functions,model uncertainty,gradient-based policy search,state-of-the-art RL algorithms
AI 理解论文
溯源树
样例
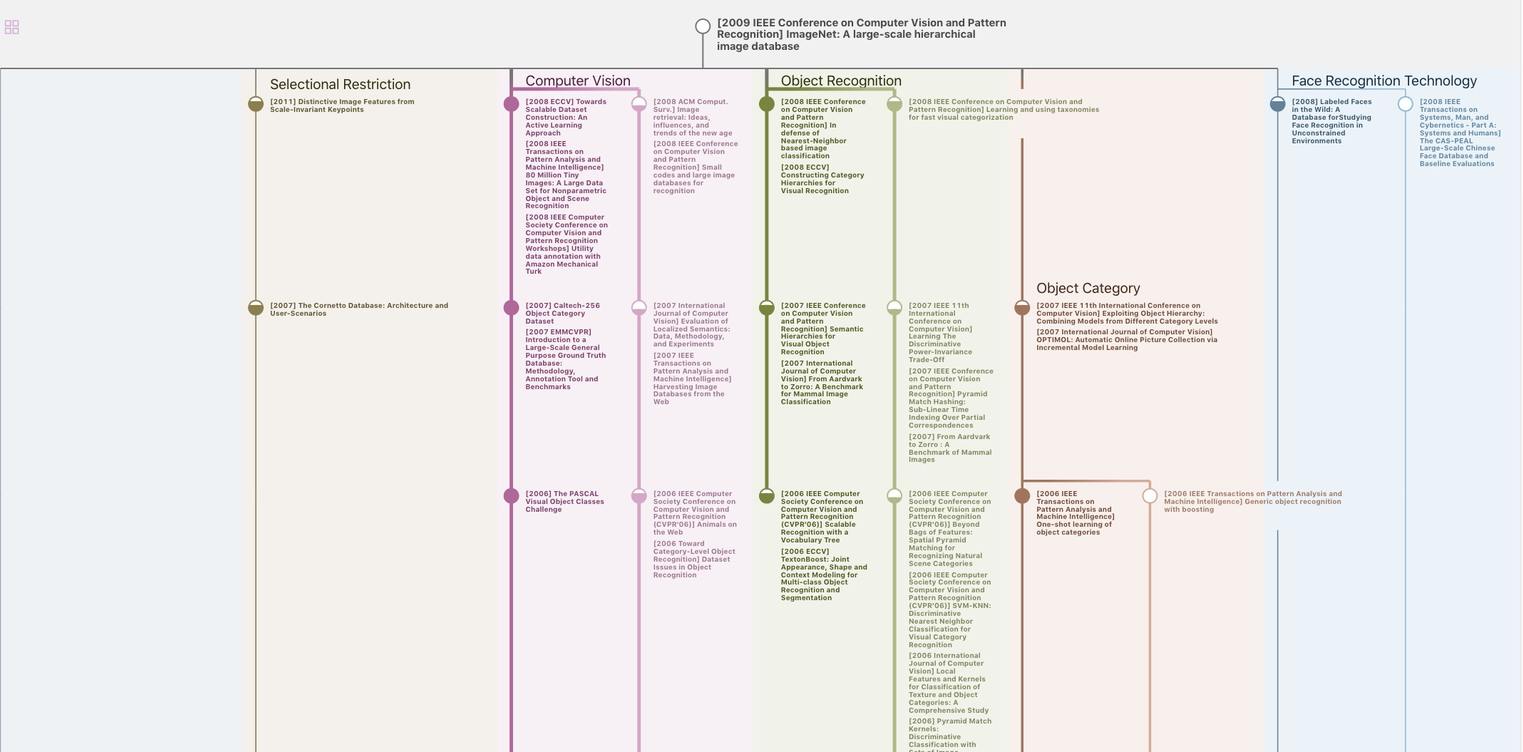
生成溯源树,研究论文发展脉络
Chat Paper
正在生成论文摘要