SoftMP: Attentive feature pooling for joint local feature detection and description for place recognition in changing environments
2021 IEEE INTERNATIONAL CONFERENCE ON ROBOTICS AND AUTOMATION (ICRA 2021)(2021)
摘要
Visual place recognition is the task of finding matchings of images that show the same place in the world. Combinations of appearance changes (e.g. changing illumination or weather) and geometric changes (e.g. viewpoint changes or occlusions) challenge existing approaches. Learning-based local image feature pipelines are a promising approach to this type of problem. We present a novel attentive feature pooling method that can be used to train a CNN to jointly detect and describe local image features. It can be trained on small or moderately sized datasets with weak supervision in a classification training setup (e.g. we use a set of 24k images of publicly available web-camera images in our experiments). We propose to use a joint loss function that combines the cross-entropy loss for the classification task with a mean squared error in order to increase the repeatability of feature detections. We show how the approach can be integrated in a place recognition pipeline and run experiments on several standard place recognition datasets. Despite the small training dataset, we demonstrate a 15% improvement in the average performance compared to the best of a number of compared state-of-the-art approaches, and, probably more importantly, a 3x improvement in the worst-case performance. Open source code is available.
更多查看译文
关键词
feature detections,place recognition pipeline,standard place recognition datasets,training dataset,compared state-of-the-art approaches,joint local feature detection,visual place recognition,appearance changes,changing illumination,geometric changes,viewpoint changes,learning-based local image feature pipelines,novel attentive feature pooling method,local image features,moderately sized datasets,classification training setup,joint loss function,cross-entropy loss,classification task,temperature 24.0 K
AI 理解论文
溯源树
样例
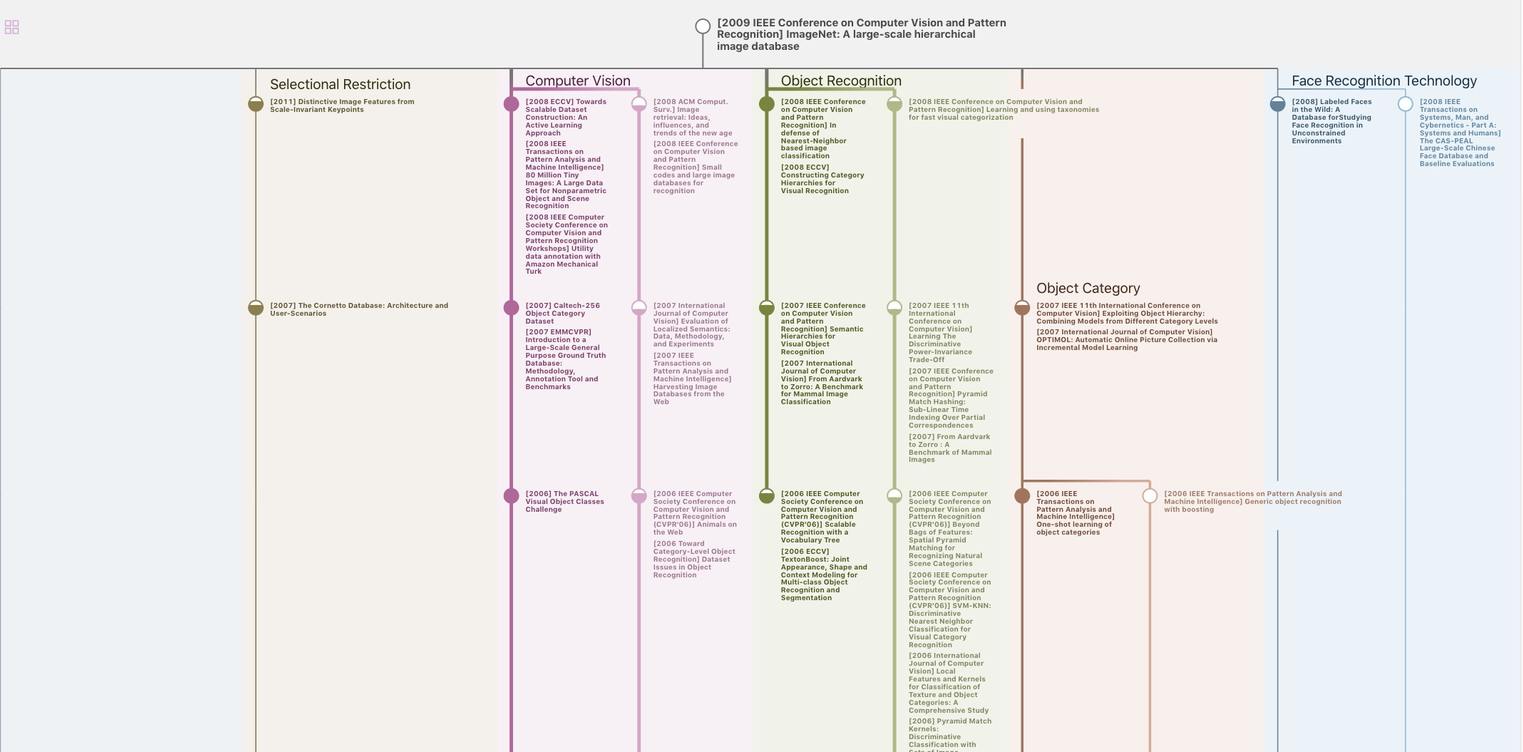
生成溯源树,研究论文发展脉络
Chat Paper
正在生成论文摘要