Reaching Pruning Locations in a Vine Using a Deep Reinforcement Learning Policy
2021 IEEE INTERNATIONAL CONFERENCE ON ROBOTICS AND AUTOMATION (ICRA 2021)(2021)
摘要
We outline a neural network-based pipeline for perception, control and planning of a 7 DoF robot for tasks that involve reaching into a dormant grapevine canopy. The proposed system consists of a 6 DoF industrial robot arm and a linear slider that can actuate on an entire grape vine. Our approach uses Convolutional Neural Networks to detect buds in dormant grape vines and a Reinforcement Learning based control strategy to reach desired cut-point locations for pruning tasks. Within this framework, three methodologies are developed and compared to reach the desired locations: the learned policy-based approach (RL), a hybrid method that uses the learned policy and an inverse kinematics solver (RL+IK), and lastly a classical approach commonly used in robotics. We first tested and validated the suitability of the proposed learning methodology in a simulated environment that resembled laboratory conditions. A reaching accuracy of up to 61.90% and 85.71% for the RL and RL+IK approaches respectively was obtained for a vine that the agent observed while learning. When testing in a new vine, the accuracy was up to 66.66% and 76.19% for RL and RL+IK, respectively. The same methods were then deployed on a real system in an end to end procedure: autonomously scan the vine using a vision system, create its model and finally use the learned policy to reach cutting points. The reaching accuracy obtained in these tests was 73.08%.
更多查看译文
关键词
cut-point locations,reaching accuracy,pruning locations,deep reinforcement learning policy,7 DoF robot,grapevine canopy,6 DoF industrial robot arm,grape vine,convolutional neural networks,inverse kinematics solver,vision system,agricultural manipulation
AI 理解论文
溯源树
样例
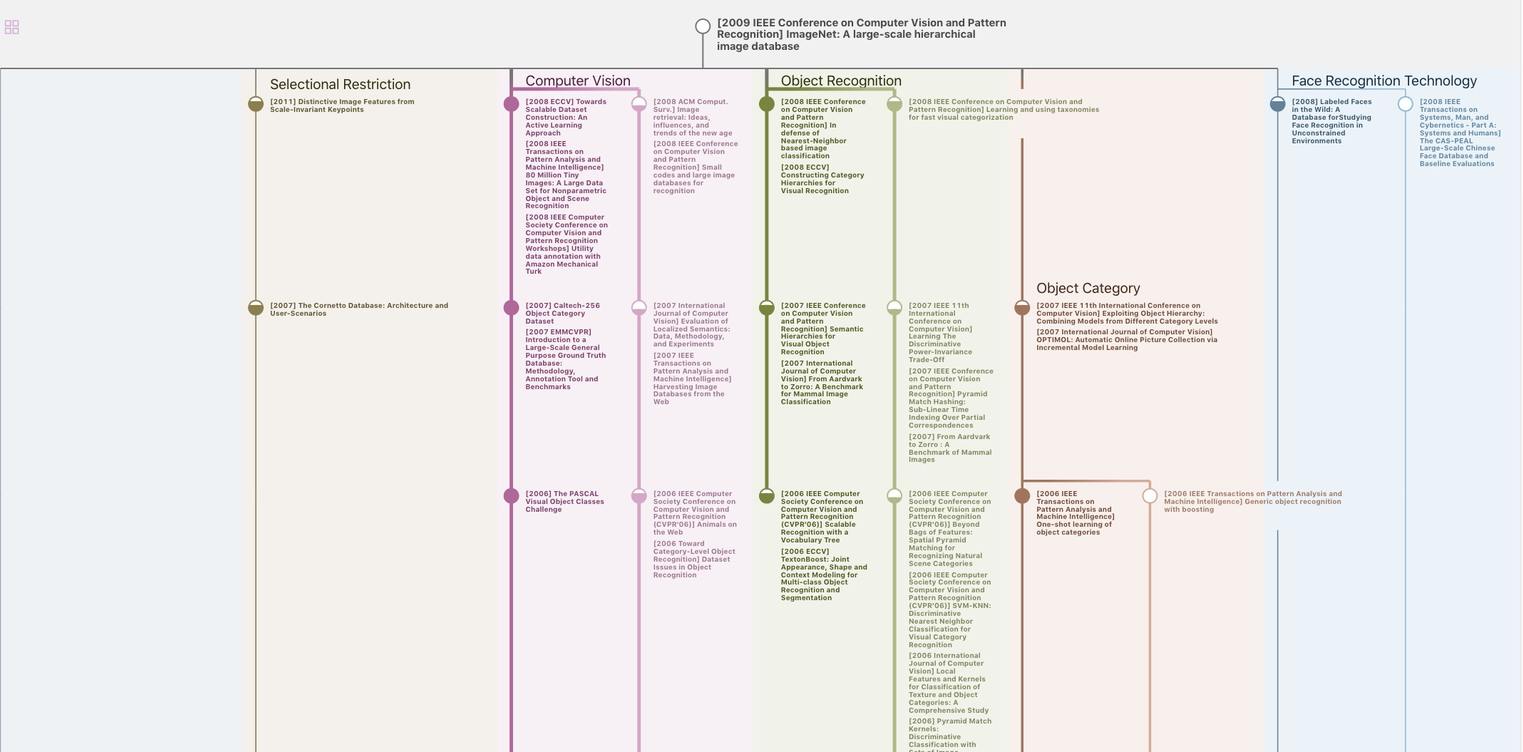
生成溯源树,研究论文发展脉络
Chat Paper
正在生成论文摘要