FlowDriveNet: An End-to-End Network for Learning Driving Policies from Image Optical Flow and LiDAR Point Flow
2021 IEEE INTERNATIONAL CONFERENCE ON ROBOTICS AND AUTOMATION (ICRA 2021)(2021)
摘要
Learning driving policies using an end-to-end network has been proved a promising solution for autonomous driving. Due to the lack of a benchmark driver behavior dataset that contains both the visual and the LiDAR data, existing works solely focus on learning driving from visual sensors. Besides, most works are limited to predict steering angle yet neglect the more challenging vehicle speed control problem. In this paper, we propose a novel end-to-end network, FlowDriveNet, which takes advantages of sequential visual data and LiDAR data jointly to predict steering angle and vehicle speed. The main challenges of this problem are how to efficiently extract driving-related information from images and point clouds, and how to fuse them effectively. To tackle these challenges, we propose a concept of point flow and declare that image optical flow and LiDAR point flow are significant motion cues for driving policy learning. Specifically, we first create an enhanced dataset that consists of images, point clouds and corresponding human driver behaviors. Then, in FlowDriveNet, a deep but efficient visual feature extraction module and a point feature extraction module are utilized to extract spatial features from optical flow and point flow, respectively. Additionally, a novel temporal fusion and prediction module is designed to fuse temporal information from the extracted spatial feature sequences and predict vehicle driving commands. Finally, a series of ablation experiments verify the importance of optical flow and point flow and comparison experiments show that our flow-based method outperforms the existing image-based approaches on the task of driving policy learning.
更多查看译文
关键词
FlowDriveNet,end-to-end network,image optical flow,LiDAR point flow,autonomous driving,benchmark driver behavior dataset,LiDAR data,steering angle,sequential visual data,point clouds,policy learning,point feature extraction module,vehicle driving commands,flow-based method,driving policy learning,vehicle speed control problem,deep visual feature extraction module
AI 理解论文
溯源树
样例
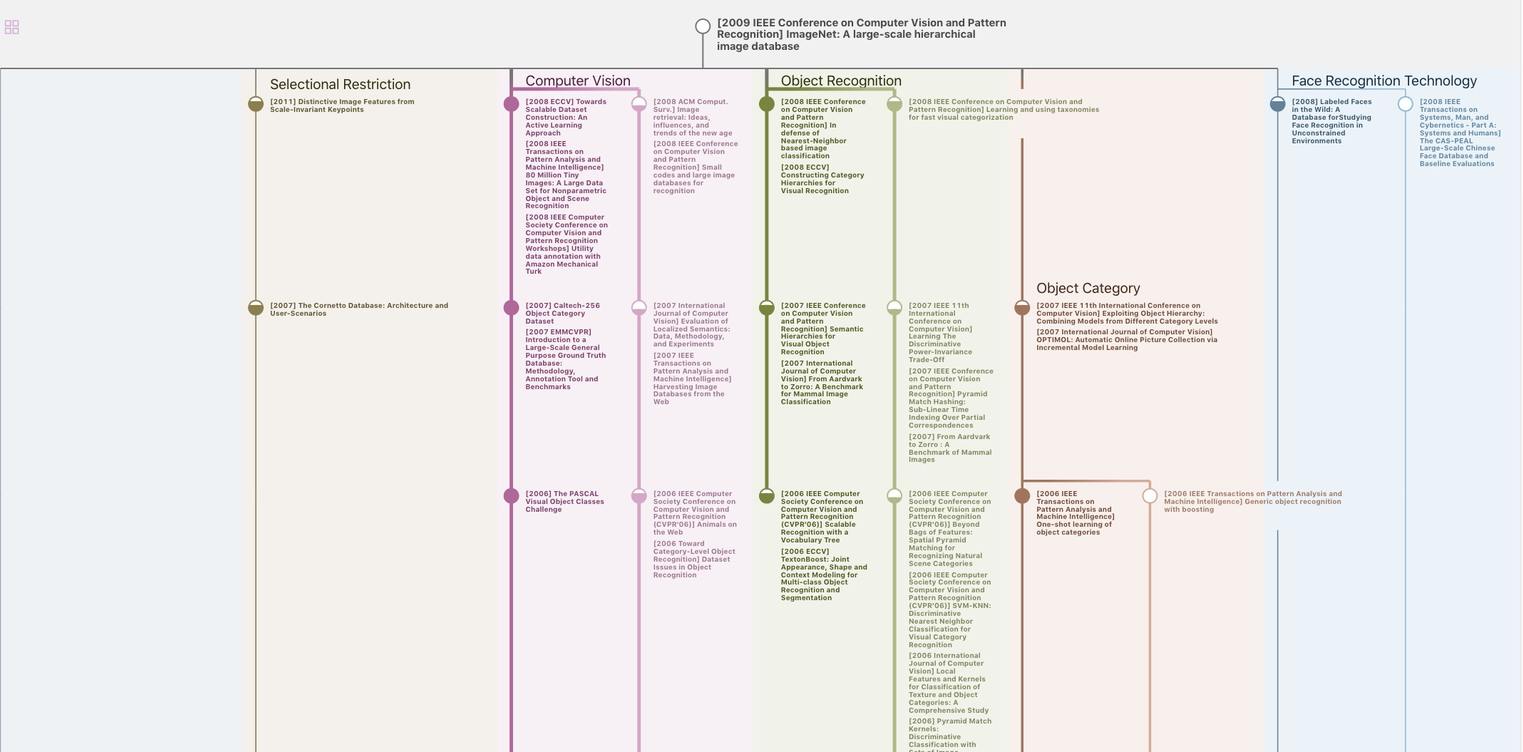
生成溯源树,研究论文发展脉络
Chat Paper
正在生成论文摘要