Point Cloud Segmentation via Edge-fused Local Graph Learning
2021 IEEE INTERNATIONAL CONFERENCE ON ROBOTICS AND AUTOMATION (ICRA 2021)(2021)
Abstract
Traditional convolution for capturing local structures and relationships remains a key technical limit in 3D semantic segmentation, which neglects the certain influence of the adjacent points on the central point in the disordered local point clouds. In this paper, we propose a novel joint-edge graph convolution neural network (JEGCN), which can extract the dynamic features of each local area and transfer the edge information between the vertex pairs to the adjacent vertices. In the proposed graph convolution module, the adjacent vertices are selected with high classification confidence which can guide the central vertex, and then reweight these vertices. Considering the lack of texture features in 3D point clouds, we incorporate 2D image features to adjacent feature propagation to effectively extract the local and global features of point clouds. The experimental results based on ScanNet and S3DIS datasets demonstrate the effectiveness of the proposed method.
MoreTranslated text
Key words
disordered local point clouds,novel joint-edge graph convolution neural network,dynamic features,local area,edge information,vertex pairs,adjacent vertices,graph convolution module,high classification confidence,central vertex,texture features,2D image features,adjacent feature propagation,local features,global features,point cloud segmentation,edge-fused local graph learning,traditional convolution,local structures,key technical limit,3D semantic segmentation,adjacent points,central point
AI Read Science
Must-Reading Tree
Example
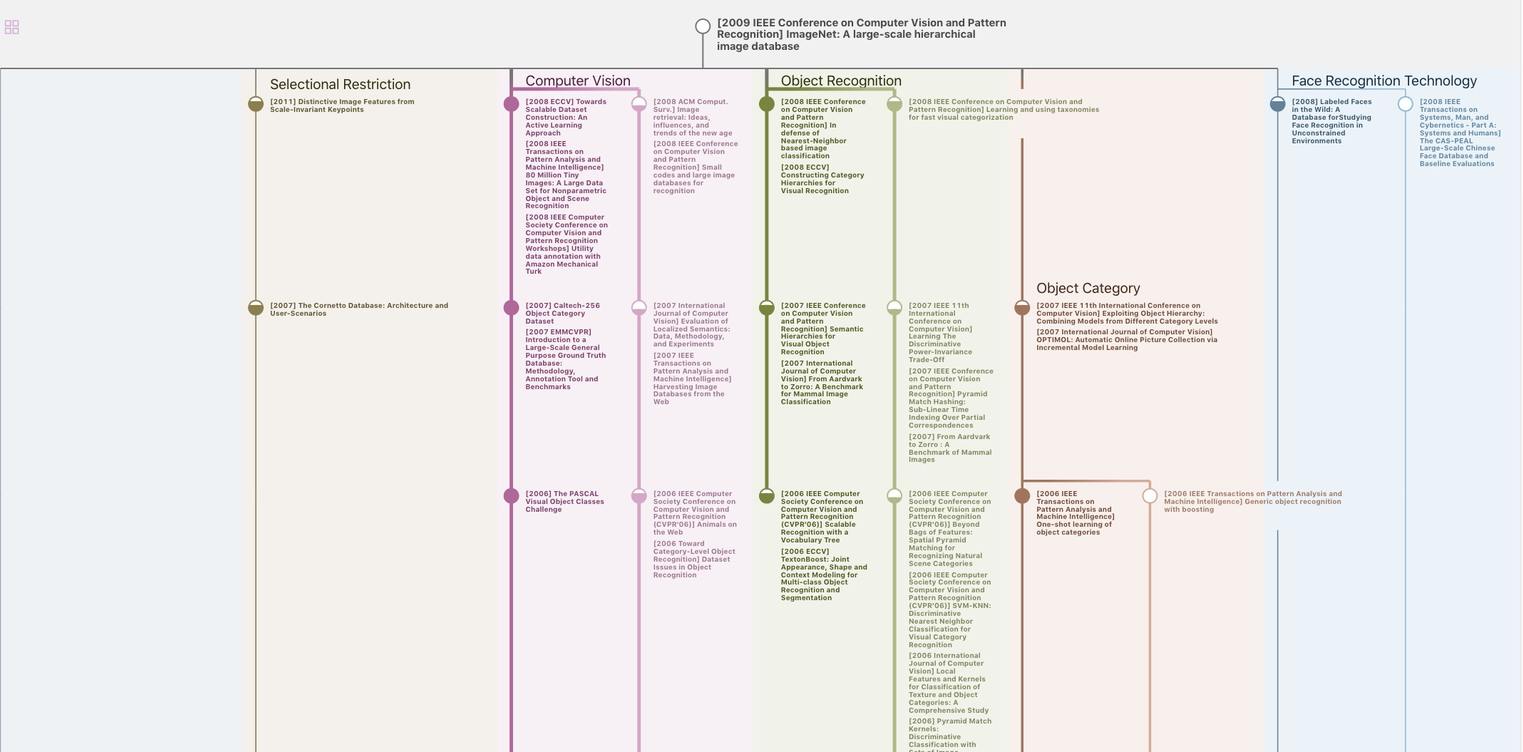
Generate MRT to find the research sequence of this paper
Chat Paper
Summary is being generated by the instructions you defined