Real-Time Trajectory Adaptation for Quadrupedal Locomotion using Deep Reinforcement Learning
2021 IEEE INTERNATIONAL CONFERENCE ON ROBOTICS AND AUTOMATION (ICRA 2021)(2021)
摘要
We present a control architecture for real-time adaptation and tracking of trajectories generated using a terrain-aware trajectory optimization solver. This approach enables us to circumvent the computationally exhaustive task of online trajectory optimization, and further introduces a control solution robust to systems modeled with approximated dynamics. We train a policy using deep reinforcement learning (RL) to introduce additive deviations to a reference trajectory in order to generate a feedback-based trajectory tracking system for a quadrupedal robot. We train this policy across a multitude of simulated terrains and ensure its generality by introducing training methods that avoid overfilling and convergence towards local optima. Additionally, in order to capture terrain information, we include a latent representation of the height maps in the observation space of the RL environment as a form of exteroceptive feedback. We test the performance of our trained policy by tracking the corrected set points using a model-based whole-body controller and compare it with the tracking behavior obtained without the corrective feedback in several simulation environments, and show that introducing the corrective feedback results in increase of the success rate from 72.7% to 92.4% for tracking precomputed dynamic long horizon trajectories on flat terrain and from 47.5% to 803% on a complex modular uneven terrain. We also show successful transfer of our training approach to the real physical system and further present cogent arguments in support of our framework.
更多查看译文
关键词
time trajectory adaptation,quadrupedal locomotion,deep reinforcement learning,control architecture,real-time adaptation,terrain-aware trajectory optimization solver,computationally exhaustive task,online trajectory optimization,approximated dynamics,additive deviations,reference trajectory,feedback-based trajectory tracking system,quadrupedal robot,simulated terrains,training methods,terrain information,RL environment,exteroceptive feedback,trained policy,corrected set points,model-based whole-body controller,tracking behavior,simulation environments,corrective feedback,precomputed dynamic long horizon trajectories,flat terrain,complex modular uneven terrain
AI 理解论文
溯源树
样例
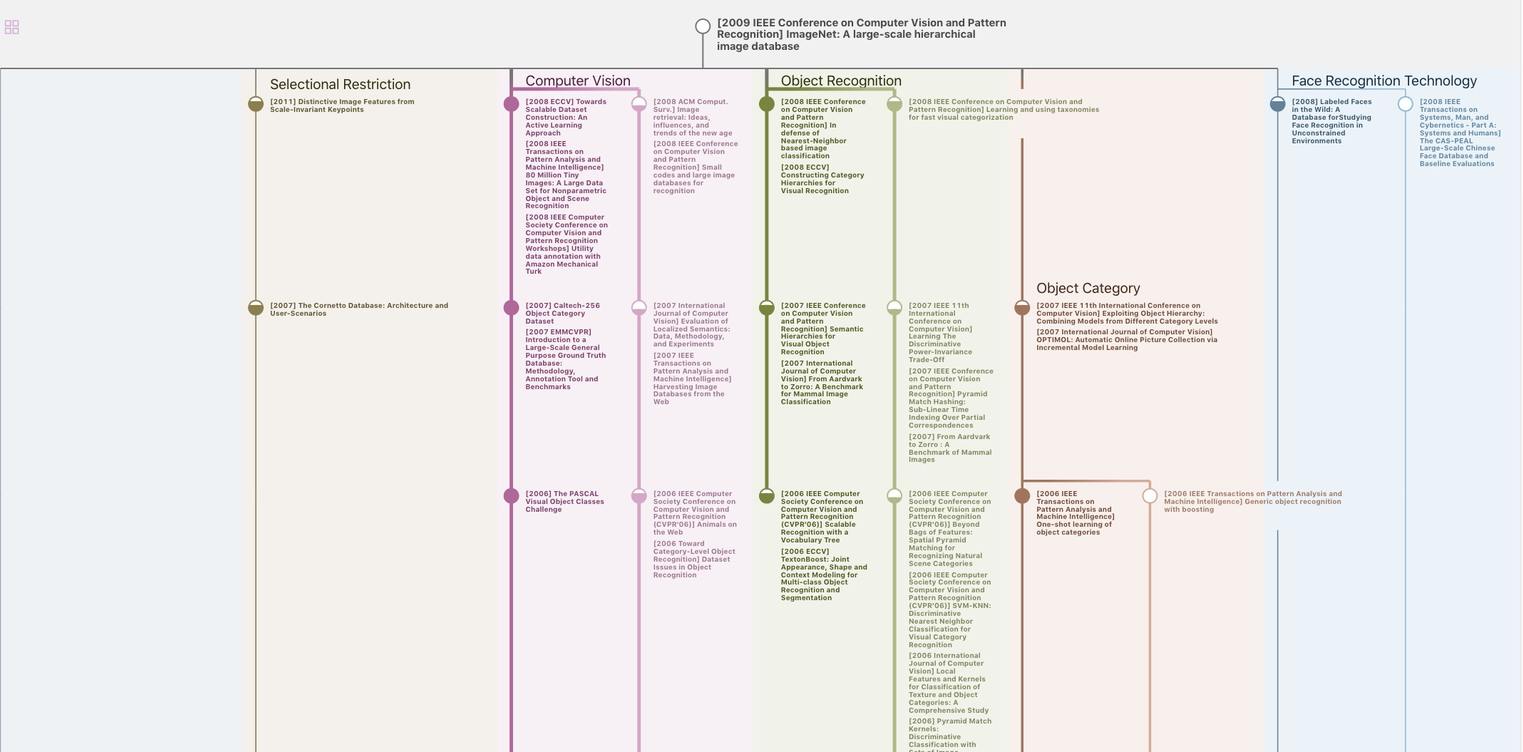
生成溯源树,研究论文发展脉络
Chat Paper
正在生成论文摘要