YOLO-ReT: Towards High Accuracy Real-time Object Detection on Edge GPUs
2022 IEEE WINTER CONFERENCE ON APPLICATIONS OF COMPUTER VISION (WACV 2022)(2022)
摘要
Performance of object detection models has been growing rapidly on two major fronts, model accuracy and efficiency. However, in order to map deep neural network (DNN) based object detection models to edge devices, one typically needs to compress such models significantly, thus compromising the model accuracy. In this paper, we propose a novel edge GPU friendly module for multi-scale feature interaction by exploiting missing combinatorial connections between various feature scales in existing state-of-the-art methods. Additionally, we propose a novel transfer learning backbone adoption inspired by the changing translational information flow across various tasks, designed to complement our feature interaction module and together improve both accuracy as well as execution speed on various edge GPU devices available in the market. For instance, YOLO-ReT with MobileNetV2x0.75 backbone runs real-time on Jetson Nano, and achieves 68.75 mAP on Pascal VOC and 34.91 mAP on COCO, beating its peers by 3.05 mAP and 0.91 mAP respectively, while executing faster by 3.05 FPS. Furthermore, introducing our multi-scale feature interaction module in YOLOv4-tiny and YOLOv4-tiny (3l) improves their performance to 41.5 and 48.1 mAP respectively on COCO, outperforming the original versions by 1.3 and 0.9 mAP.
更多查看译文
关键词
detection,high accuracy,real-time real-time,yolo-ret
AI 理解论文
溯源树
样例
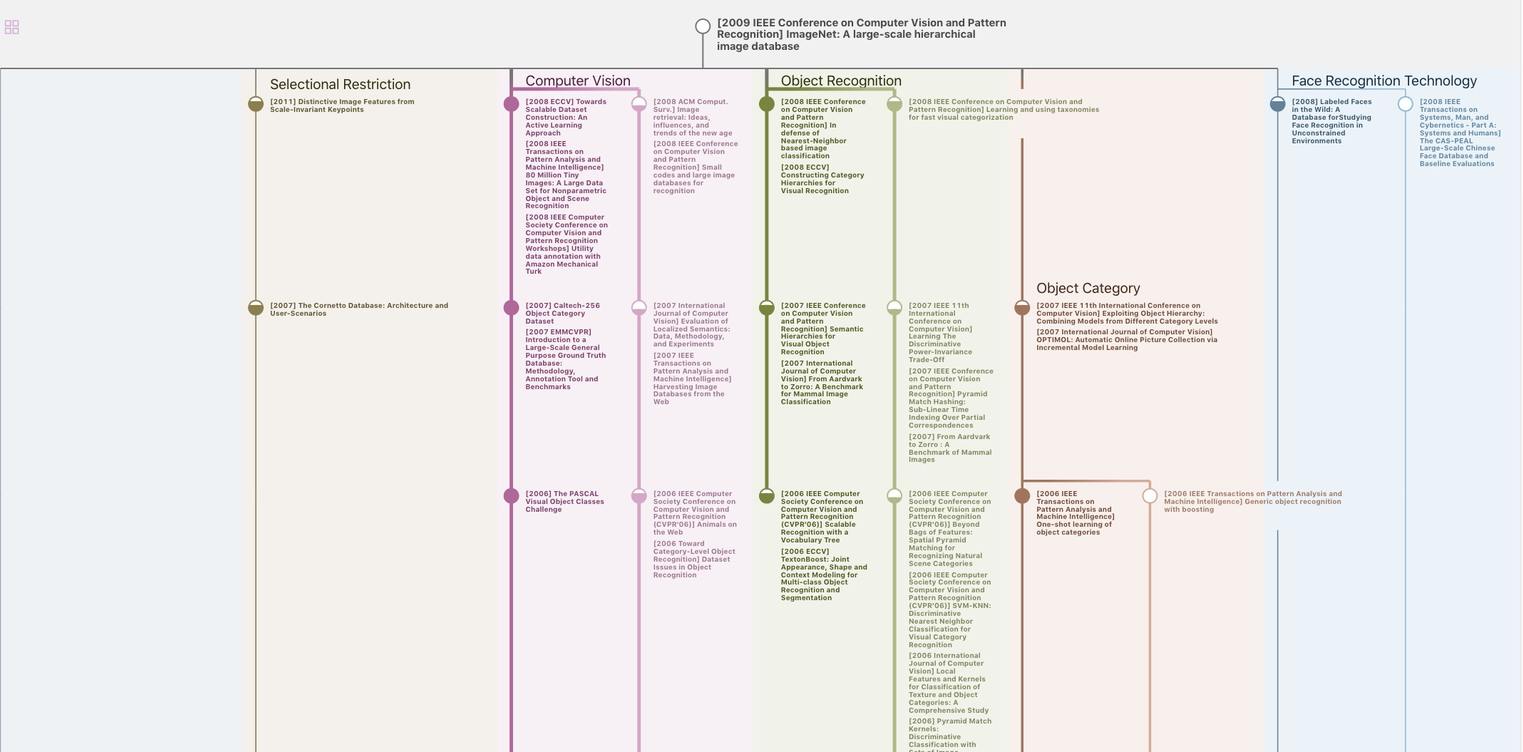
生成溯源树,研究论文发展脉络
Chat Paper
正在生成论文摘要