Calibrating DFT Formation Enthalpy Calculations by Multifidelity Machine Learning.
JACS Au(2022)
摘要
The application of machine learning to predict materials properties measured by experiments are valuable yet difficult due to the limited amount of experimental data. In this work, we use a multifidelity random forest model to learn the experimental formation enthalpy of materials with prediction accuracy higher than the Perdew-Burke-Ernzerhof (PBE) functional with linear correction, PBEsol, and meta-generalized gradient approximation (meta-GGA) functionals (SCAN and rSCAN), and it outperforms the hotly studied deep neural network-based representation learning and transfer learning. We then use the model to calibrate the DFT formation enthalpy in the Materials Project database and discover materials with underestimated stability. The multifidelity model is also used as a data-mining approach to find how DFT deviates from experiments by explaining the model output.
更多查看译文
关键词
formation enthalpy,stability,machine learning,DFT,multifidelity,transfer learning
AI 理解论文
溯源树
样例
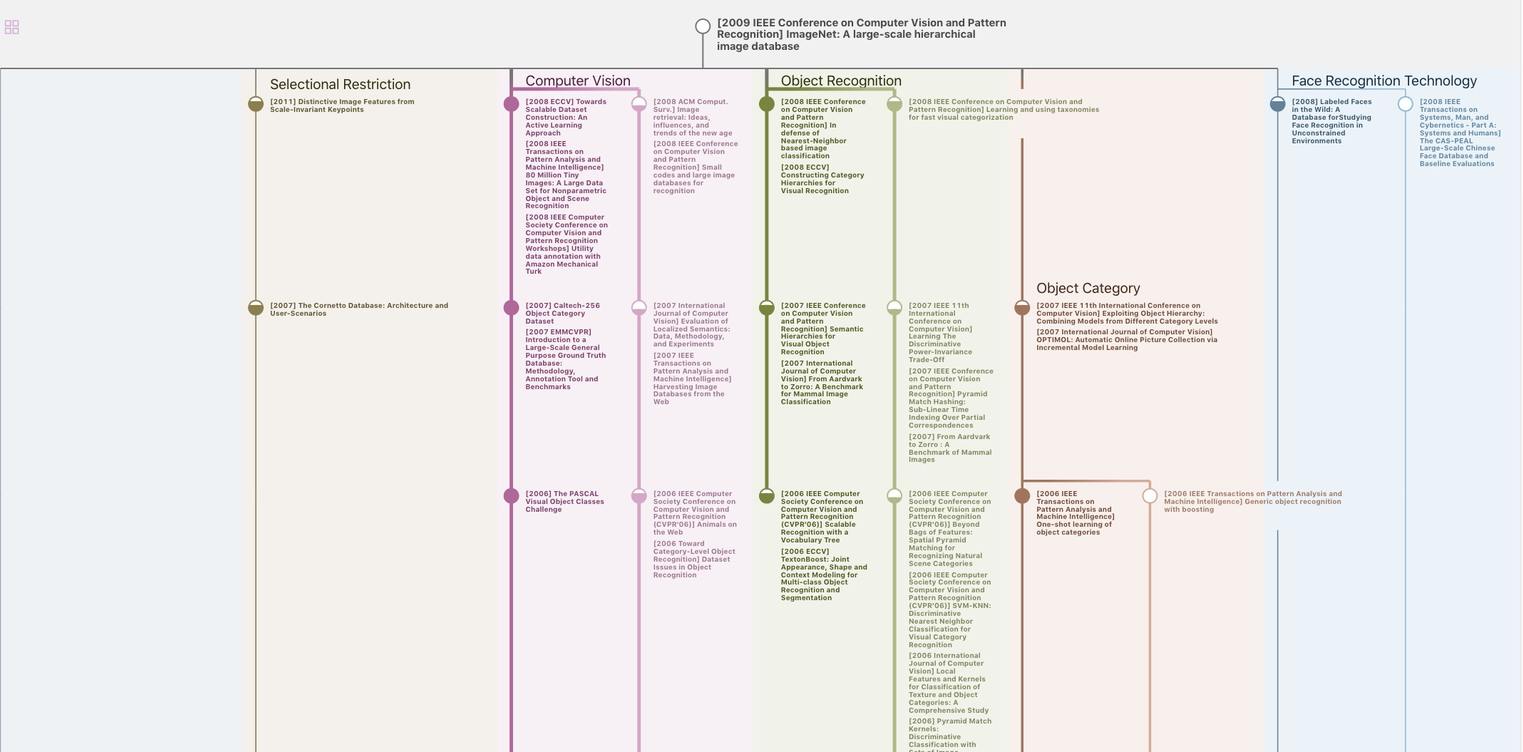
生成溯源树,研究论文发展脉络
Chat Paper
正在生成论文摘要