Post-Regularization Confidence Bands for Ordinary Differential Equations
JOURNAL OF MACHINE LEARNING RESEARCH(2024)
Abstract
Ordinary differential equation (ODE) is an important tool to study a system of biological and physical processes. A central question in ODE modeling is to infer the significance of individual regulatory effect of one signal variable on another. However, building confidence band for ODE with unknown regulatory relations is challenging, and it remains largely an open question. In this article, we construct the post-regularization confidence band for the individual regulatory function in ODE with unknown functionals and noisy data observations. Our proposal is the first of its kind, and is built on two novel ingredients. The first is a new localized kernel learning approach that combines reproducing kernel learning with local Taylor approximation, and the second is a new de-biasing method that tackles infinite -dimensional functionals and additional measurement errors. We show that the constructed confidence band has the desired asymptotic coverage probability, and the recovered regulatory network approaches the truth with probability tending to one. We establish the theoretical properties when the number of variables in the system can be either smaller or larger than the number of sampling time points, and we study the regimeswitching phenomenon. We demonstrate the efficacy of the proposed method through both simulations and illustrations with two data applications.
MoreTranslated text
Key words
De-biasing,Local polynomial approximation,Ordinary differential equations,Reproducing kernel Hilbert space,Smoothing spline analysis of variance,Time series
AI Read Science
Must-Reading Tree
Example
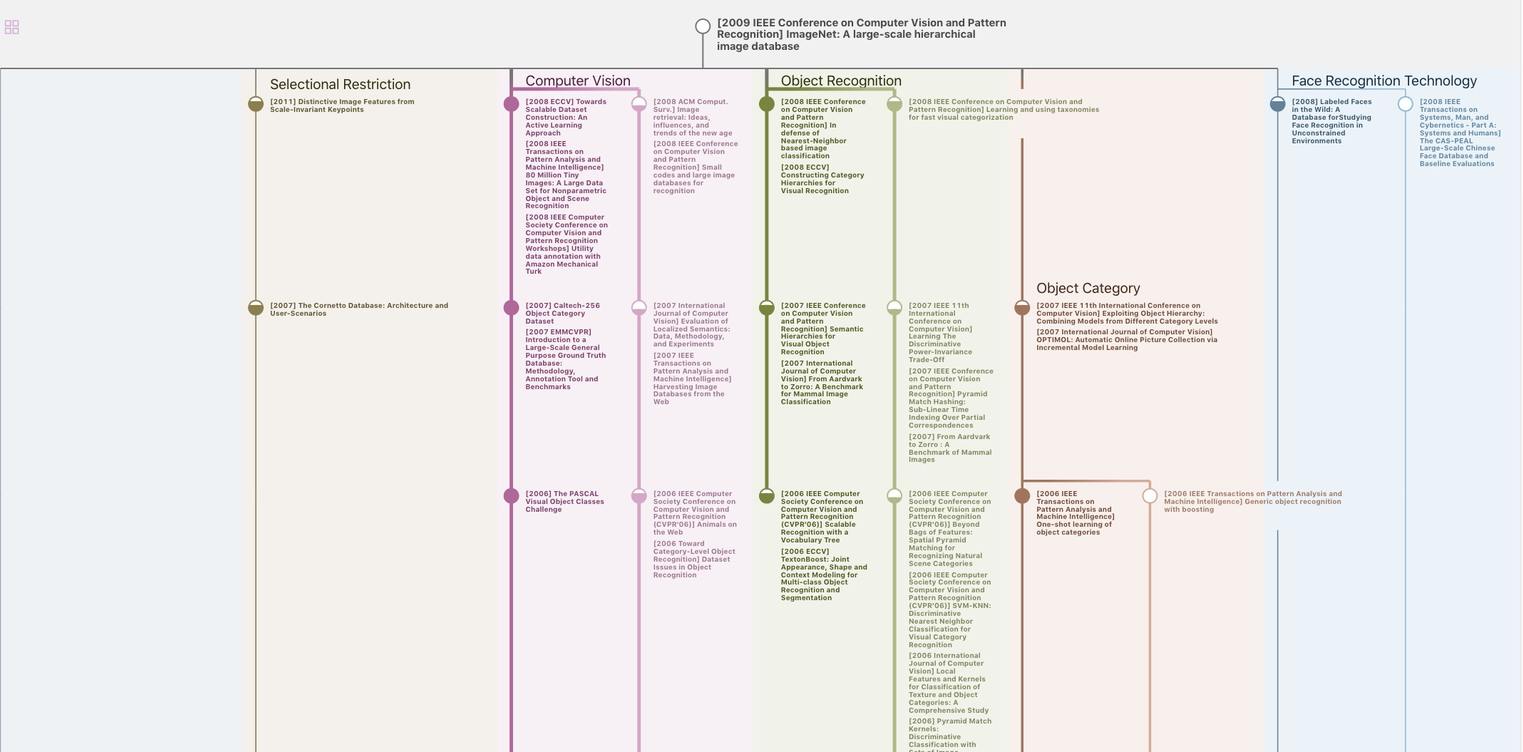
Generate MRT to find the research sequence of this paper
Chat Paper
Summary is being generated by the instructions you defined