Vertebrae localization, segmentation and identification using a graph optimization and an anatomic consistency cycle
arxiv(2022)
摘要
Vertebrae localization, segmentation and identification in CT images is key to numerous clinical applications. While deep learning strategies have brought to this field significant improvements over recent years, transitional and pathological vertebrae are still plaguing most existing approaches as a consequence of their poor representation in training datasets. Alternatively, proposed non-learning based methods take benefit of prior knowledge to handle such particular cases. In this work we propose to combine both strategies. To this purpose we introduce an iterative cycle in which individual vertebrae are recursively localized, segmented and identified using deep-networks, while anatomic consistency is enforced using statistical priors. In this strategy, the transitional vertebrae identification is handled by encoding their configurations in a graphical model that aggregates local deep-network predictions into an anatomically consistent final result. Our approach achieves state-of-the-art results on the VerSe20 challenge benchmark, and outperforms all methods on transitional vertebrae as well as the generalization to the VerSe19 challenge benchmark. Furthermore, our method can detect and report inconsistent spine regions that do not satisfy the anatomic consistency priors. Our code and model are openly available for research purposes.
更多查看译文
关键词
Spine,Vertebrae segmentation,Vertebrae labelling,Transitional vertebrae
AI 理解论文
溯源树
样例
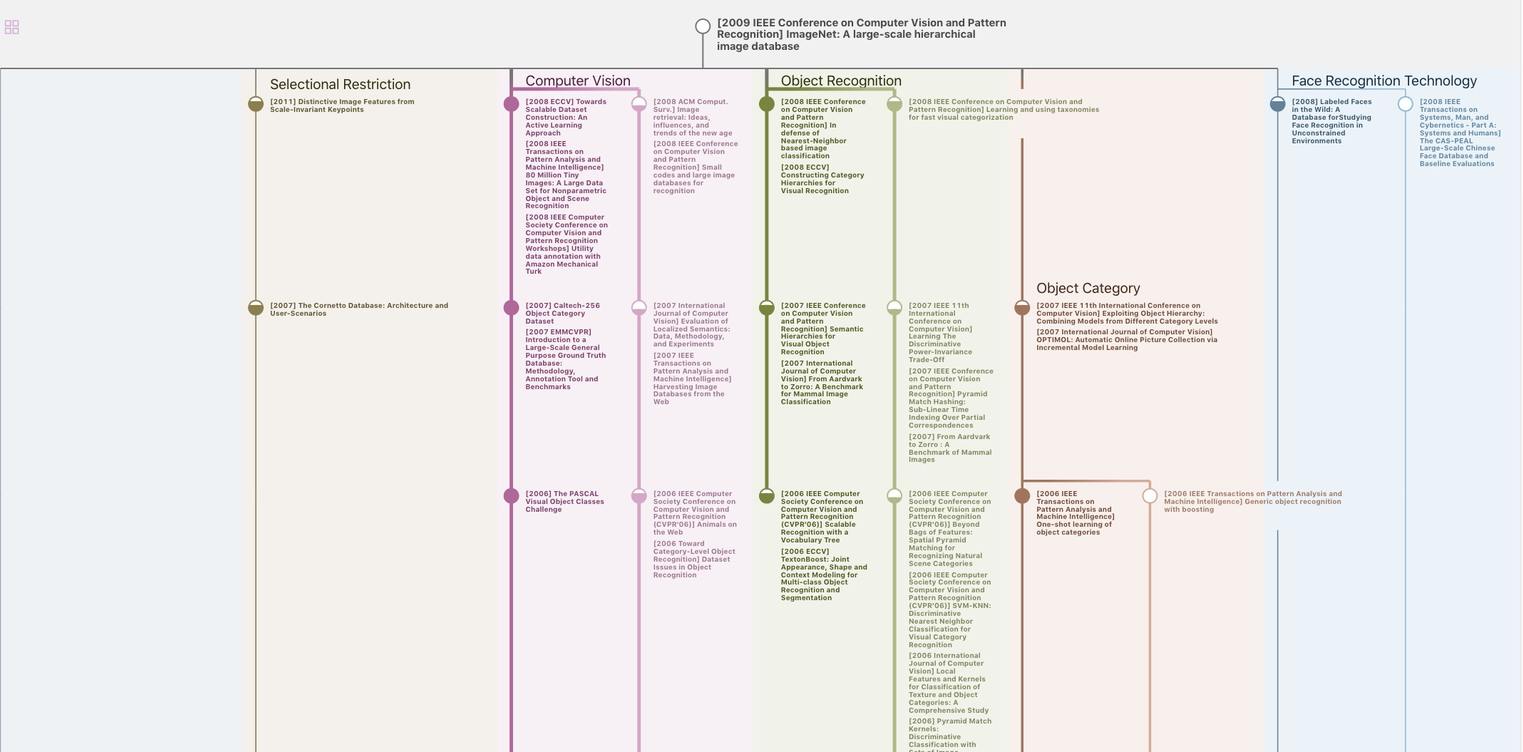
生成溯源树,研究论文发展脉络
Chat Paper
正在生成论文摘要