Electromagnetic shower reconstruction and energy validation with Michel electrons and pi(0) samples for the deep-learning-based analyses in MicroBooNE
JOURNAL OF INSTRUMENTATION(2021)
摘要
This article presents the reconstruction of the electromagnetic activity from electrons and photons (showers) used in the MicroBooNE deep learning-based low energy electron search. The reconstruction algorithm uses a combination of traditional and deep learning-based techniques to estimate shower energies. We validate these predictions using two nu(mu)-sourced data samples: charged/neutral current interactions with final state neutral pions and charged current interactions in which the muon stops and decays within the detector producing a Michel electron. Both the neutral pion sample and Michel electron sample demonstrate agreement between data and simulation. Further, the absolute shower energy scale is shown to be consistent with the relevant physical constant of each sample: the neutral pion mass peak and the Michel energy cutoff.
更多查看译文
关键词
Neutrino detectors, Noble liquid detectors (scintillation, ionization, double-phase), Pattern recognition, cluster finding, calibration and fitting methods, Time projection Chambers (TPC)
AI 理解论文
溯源树
样例
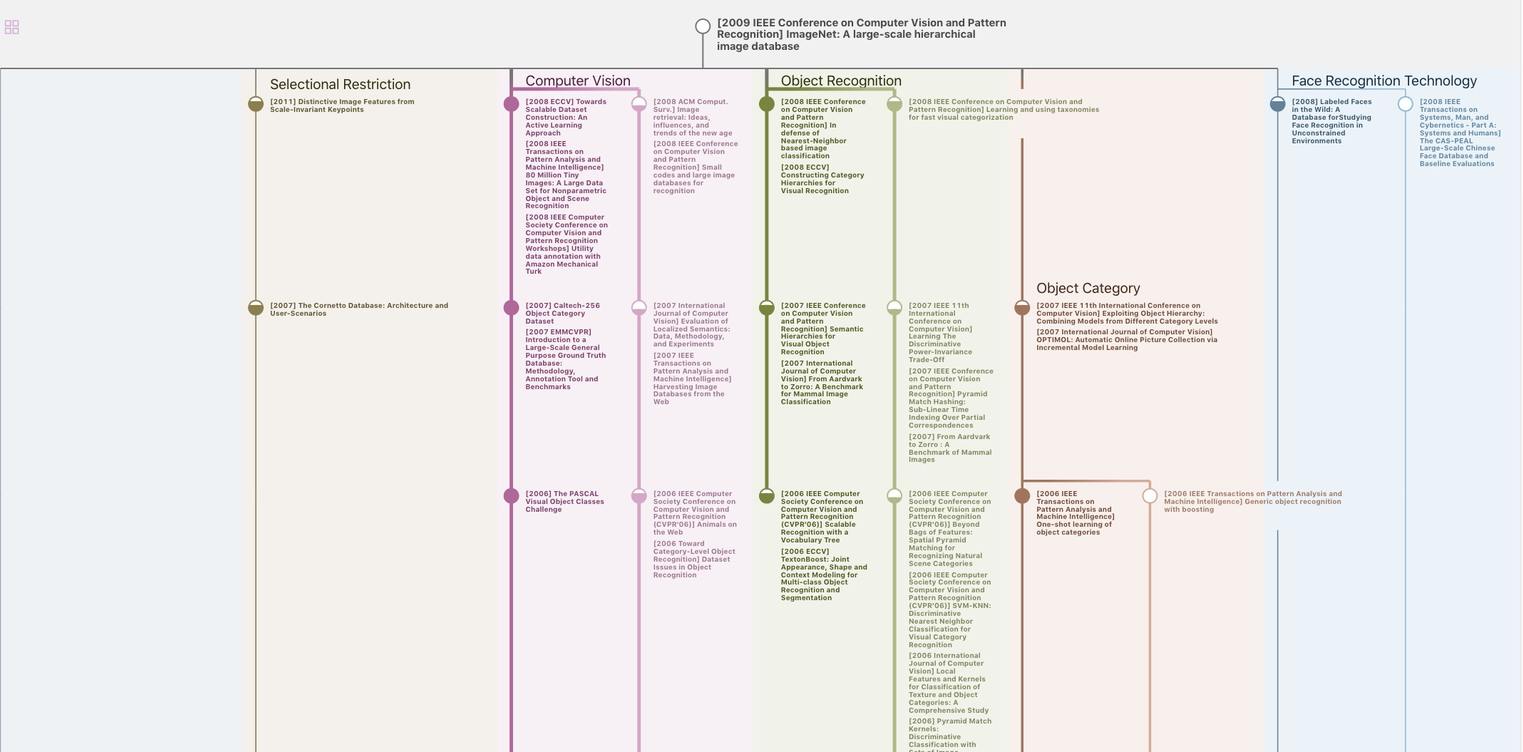
生成溯源树,研究论文发展脉络
Chat Paper
正在生成论文摘要