Few-shot Semantic Segmentation with Self-supervision from Pseudo-classes.
British Machine Vision Conference(2021)
摘要
Despite the success of deep learning methods for semantic segmentation, few-shot semantic segmentation remains a challenging task due to the limited training data and the generalisation requirement for unseen classes. While recent progress has been particularly encouraging, we discover that existing methods tend to have poor performance in terms of meanIoU when query images contain other semantic classes besides the target class. To address this issue, we propose a novel self-supervised task that generates random pseudo-classes in the background of the query images, providing extra training data that would otherwise be unavailable when predicting individual target classes. To that end, we adopted superpixel segmentation for generating the pseudo-classes. With this extra supervision, we improved the meanIoU performance of the state-of-the-art method by 2.5% and 5.1% on the one-shot tasks, as well as 6.7% and 4.4% on the five-shot tasks, on the PASCAL-5i and COCO benchmarks, respectively.
更多查看译文
关键词
few-shot,self-supervision,pseudo-classes
AI 理解论文
溯源树
样例
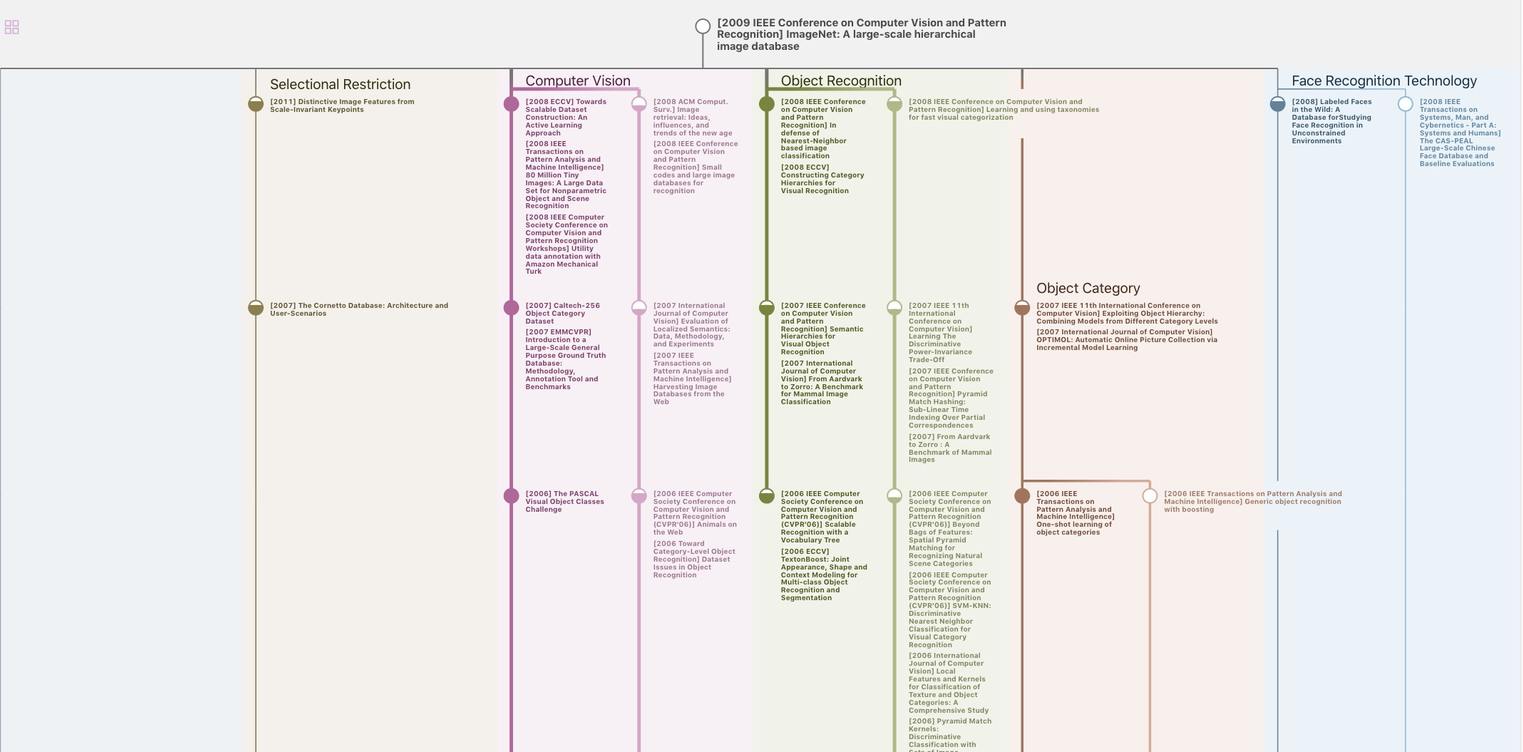
生成溯源树,研究论文发展脉络
Chat Paper
正在生成论文摘要